Title
题目
Alzheimer’s disease diagnosis from multi-modal data via feature inductive learning and dual multilevel graph neural network
通过特征归纳学习和双重多级图神经网络从多模态数据中诊断阿尔茨海默病
01
文献速递介绍
阿尔茨海默病(AD)是一种常见于老年人的慢性神经退行性疾病,也是导致痴呆的主要原因之一(Khachaturian, 1985; Kucmanski 等, 2016)。AD已经成为21世纪成本最高、死亡率最高、负担最重的疾病之一(Sharma 等, 2019)。因此,诊断AD对于预防其发生和发展具有重要的现实意义(Scheltens 等, 2021)。此外,AD的确切病因仍然不清楚(Gaugler 等, 2022)。识别与AD相关的风险因素对于理解疾病的发病机制以及辅助精确诊断和治疗具有重要意义。
结构磁共振成像(MRI)能够在无创条件下捕捉大脑的结构变化(Vemuri 和 Jack, 2010),因此在AD诊断领域得到了广泛应用(Zarei 等, 2010)。另一方面,随着基因组学的不断发展,研究人员发现一些单核苷酸多态性(SNP)与AD患者的特定大脑结构变化高度相关(Harold 等, 2009; Feulner 等, 2010)。同时,与AD发展相关的神经病理学变化也受到了广泛关注。研究人员发现,脑脊液(CSF)中A-beta蛋白和tau蛋白的异常变化与AD高度相关(Hansson 等, 2006; Kunkle 等, 2019)。此外,研究还表明,年龄、性别和体重也与AD的发生有关(Harold 等, 2009; Scheltens 等, 2021)。
Aastract
摘要
Multi-modal data can provide complementary information of Alzheimer’s disease (AD) and its developmentfrom different perspectives. Such information is closely related to the diagnosis, prevention, and treatment ofAD, and hence it is necessary and critical to study AD through multi-modal data. Existing learning methods,however, usually ignore the influence of feature heterogeneity and directly fuse features in the last stages.Furthermore, most of these methods only focus on local fusion features or global fusion features, neglectingthe complementariness of features at different levels and thus not sufficiently leveraging information embeddedin multi-modal data. To overcome these shortcomings, we propose a novel framework for AD diagnosis thatfuses gene, imaging, protein, and clinical data. Our framework learns feature representations under the samefeature space for different modalities through a feature induction learning (FIL) module, thereby alleviatingthe impact of feature heterogeneity. Furthermore, in our framework, local and global salient multi-modalfeature interaction information at different levels is extracted through a novel dual multilevel graph neuralnetwork (DMGNN). We extensively validate the proposed method on the Alzheimer’s Disease NeuroimagingInitiative (ADNI) dataset and experimental results demonstrate our method consistently outperforms otherstate-of-the-art multi-modal fusion methods. The code is publicly available on the GitHub website.
多模态数据可以从不同角度提供关于阿尔茨海默病(AD)及其发展过程的互补信息,这些信息与AD的诊断、预防和治疗密切相关,因此通过多模态数据研究AD是必要且关键的。然而,现有的学习方法通常忽略了特征异质性的影响,并且在最后阶段直接融合特征。此外,大多数这些方法仅关注局部融合特征或全局融合特征,忽视了不同层次特征的互补性,从而未能充分利用嵌入在多模态数据中的信息。为克服这些不足,我们提出了一种新颖的AD诊断框架,该框架融合了基因、成像、蛋白质和临床数据。我们的框架通过特征归纳学习(FIL)模块,在相同特征空间下学习不同模态的特征表示,从而减轻了特征异质性的影响。此外,在我们的框架中,通过一种新颖的双重多级图神经网络(DMGNN)提取不同层次的局部和全局显著多模态特征交互信息。我们在阿尔茨海默病神经影像计划(ADNI)数据集上对所提出的方法进行了广泛验证,实验结果表明,我们的方法始终优于其他最先进的多模态融合方法。代码已在GitHub网站上公开提供。
Method
方法
In order to better compare the differences between other methodsand the proposed method of this paper, we have drawn an overviewof existing research and frameworks. Fig. 1(a) corresponds to thefirst question in the introduction, the traditional multi-modal featurerepresentation method still has feature heterogeneity. Fig. 1(b) showsthe second problem in the introduction, that is, the existing methodscannot jointly learn local and global features. These problems all affectthe model’s learning of multi-modal feature information. Fig. 1©presents the framework of our method. Among them, the above FILmodule is used to solve the first problem, and the following multi-levelfusion learning module of local and global features is used to solve thesecond problem. As shown in Fig. 1, we build a sparse multi-modalnetwork and build a FIL-DMGNN model to inductively learn local andglobal feature information to capture the pathogenic factors of differentmodalities, and further explore the relationship between other modalities and interrelationship patterns. Specifically, we first preprocess andnormalize the data of each modality into sequence information, andbuild a multi-modal network by Pearson coefficient (PC). Second, welearn the representation information of different modal features in astandard feature representation space based on the FIL module. Then,the local and global feature information under multiple levels is learnedbased on the DMGNN network. Finally, the feature extraction module isused to obtain the individual representation features for AD diagnosistasks, and the features are interpreted through the gradient saliencymechanism. Table 2 summarizes the important symbols used in thispaper. Scalars are represented by ordinary lowercase and uppercaseletters. Vectors and matrices are denoted by bold lowercase letters andbold uppercase letters, respectively.
为了更好地比较其他方法与本文提出方法的差异,我们绘制了现有研究和框架的概述。图1(a)对应于引言中的第一个问题,即传统的多模态特征表示方法仍然存在特征异质性。图1(b)展示了引言中的第二个问题,即现有方法无法同时学习局部和全局特征。这些问题都会影响模型对多模态特征信息的学习。图1©展示了我们方法的框架,其中,上述的FIL模块用于解决第一个问题,而以下的多级局部和全局特征融合学习模块用于解决第二个问题。如图1所示,我们构建了一个稀疏的多模态网络,并建立了FIL-DMGNN模型,以归纳学习局部和全局特征信息,从而捕捉不同模态的致病因素,并进一步探索其他模态之间的关系及其相互关系模式。具体来说,我们首先对每种模态的数据进行预处理和规范化,将其转换为序列信息,并通过皮尔逊系数(PC)构建多模态网络。其次,我们基于FIL模块在标准特征表示空间中学习不同模态特征的表示信息。然后,基于DMGNN网络学习多个层次下的局部和全局特征信息。最后,使用特征提取模块获取用于AD诊断任务的个体表示特征,并通过梯度显著性机制对这些特征进行解释。表2总结了本文使用的重要符号。标量用普通小写和大写字母表示。向量和矩阵分别用粗体小写字母和粗体大写字母表示。
Conclusion
结论
In this paper, we address AD-related issues using multi-modal data.In order to make full use of the complementary information betweenmulti-modal data, we construct the FIL-DMGNN model. A commonlatent feature space among multi-modal features is found throughthe FIL module. The DMGNN can better learn the local and globalfeatures. Also, the multi-level fusion operation can effectively utilizethe early and late fusion features. After three prediction tasks basedon different modality data, we also perform the statistical analysisand corresponding biological interpretation of the features extractedby the model. Experimental results illustrate the superiority of ourmodel in AD disease prediction and risk factor mining. The risk markersextracted by our model were highly correlated with the developmentof AD, which was verified in existing studies. This provides a referencefor future disease research and clinical diagnosis.
在本文中,我们利用多模态数据解决与阿尔茨海默病(AD)相关的问题。为了充分利用多模态数据之间的互补信息,我们构建了FIL-DMGNN模型。通过FIL模块找到多模态特征之间的共同潜在特征空间,DMGNN能够更好地学习局部和全局特征。此外,多级融合操作可以有效利用早期和晚期的融合特征。在基于不同模态数据的三个预测任务之后,我们还对模型提取的特征进行了统计分析和相应的生物学解释。实验结果表明,我们的模型在AD疾病预测和风险因素挖掘方面具有优势。模型提取的风险标志物与AD的发展高度相关,并在现有研究中得到了验证。这为未来的疾病研究和临床诊断提供了参考。
Figure
图
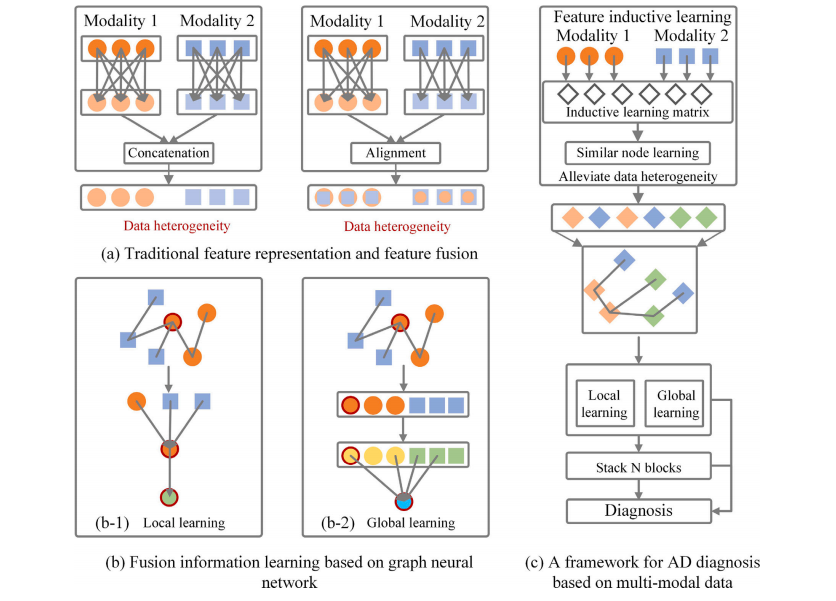
Fig. 1. Summary of existing research and the proposed framework. Fig. 1(a) shows two common practices of traditional multi-modal learning methods, Concatenation and Alignment.When the data is processed into sequence information, the traditional method learns the data within the modality, and then splices or aligns the learned multi-modal information.The former method cannot exchange information between multi-modal data, and Alignment is unable to fully exchange information. Fig. 1(b) shows the learning methods of twotarget node features of the GNN in the current multi-modal learning. Red circles indicate target nodes. b-1: Local information learning, ignoring unexpected information of adjacentnodes, b-2: Global feature learning, focusing on information interaction between global nodes. Fig. 1© uses the FIL method to perform feature representation and alleviate dataheterogeneity. Afterward, through multi-level local and global feature fusion, the effective feature information is maximized for diagnostic tasks.
图1. 现有研究和所提出框架的概述。图1(a)展示了传统多模态学习方法的两种常见做法,分别是拼接和对齐。当数据被处理为序列信息时,传统方法在模态内学习数据,然后将学习到的多模态信息进行拼接或对齐。前者无法在多模态数据之间交换信息,而对齐方法无法充分交换信息。图1(b)展示了当前多模态学习中GNN的两种目标节点特征学习方法。红色圆圈表示目标节点。b-1:局部信息学习,忽略了相邻节点的意外信息;b-2:全局特征学习,关注全局节点之间的信息交互。图1©使用FIL方法进行特征表示,缓解数据异质性。之后,通过多级局部和全局特征融合,最大化诊断任务中的有效特征信息。
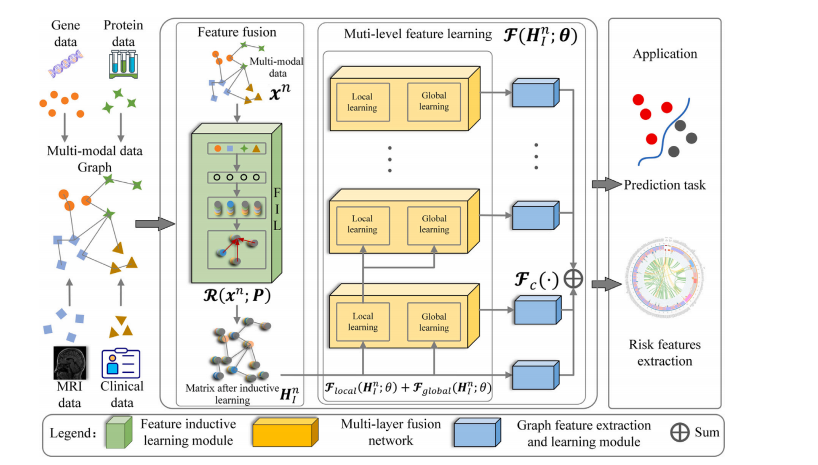
Fig. 2. The proposed framework for AD diagnosis. The left part of Fig. 2 is the preprocessing part of multi-modal data, which serializes and produces graph data. The featurerepresentation in the middle corresponds to the function in the following text, projecting multi-modal data into a potential common feature space. The intermediate multi-levelfeature fusion corresponds to the function in the following text, which further fuses the feature information (yellow module) and extracts it (blue module). Finally, on the rightare the two tasks of AD diagnosis and risk factor identification implemented in this article..
图2. 用于阿尔茨海默病(AD)诊断的所提出框架。图2的左部分是多模态数据的预处理部分,它将数据序列化并生成图数据。中间的特征表示对应于下文中的函数,将多模态数据投射到一个潜在的公共特征空间。中间的多级特征融合对应于下文中的函数,进一步融合特征信息(黄色模块)并提取特征(蓝色模块)。最后,右侧是本文中实施的AD诊断和风险因素识别这两个任务。
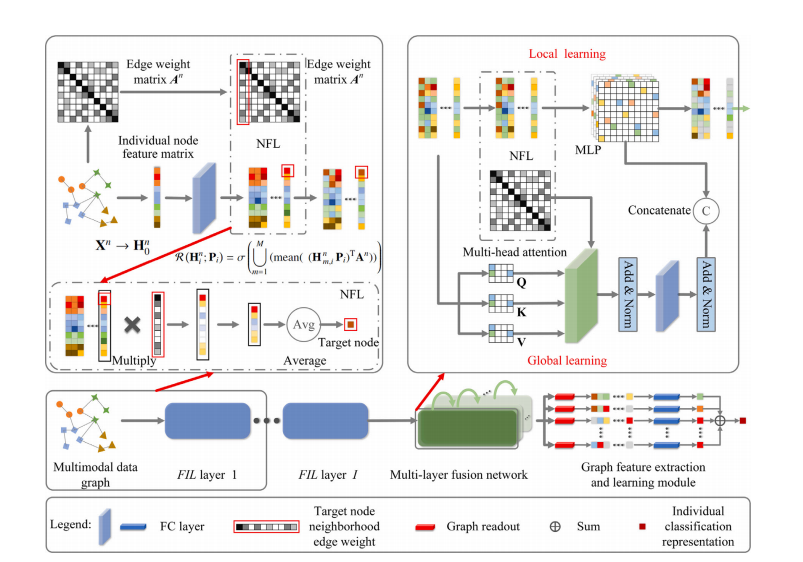
Fig. 3. FIL-DMGNN detailed process. This figure mainly represents the specific process details of the framework, including the FIL module (function ) in the feature representationsection, the local and global feature learning modules in multi-level feature fusion, and the graph feature extraction module.
图3. FIL-DMGNN的详细过程。该图主要展示了框架的具体过程细节,包括特征表示部分的FIL模块(函数)、多级特征融合中的局部和全局特征学习模块,以及图特征提取模块。
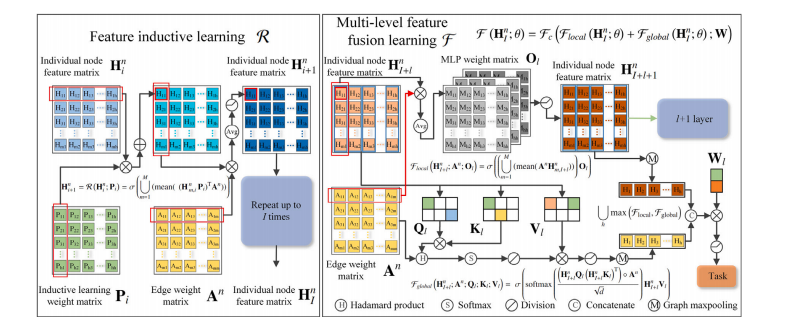
Fig. 4. Local and global feature learning extraction algorithm diagram. This figure is an algorithm description of the process in Fig. 3. The left function represents the algorithmof the FIL module. Project the feature matrix through inductive learning matrix P (searching for potential common feature space). The adjacency matrix is introduced to alleviatethe ‘‘learning competition’’ phenomenon of different modes. The right function is divided into three parts, local learning, global feature learning, and the graph feature extractionlearning part for diagnostic tasks. MLP is used to interact with multi-channel information, and the multi-head attention mechanism enhances global information learning. Graphmax pooling extracts the most representative information of each hidden image for diagnostic tasks..
图4. 局部和全局特征学习提取算法图。此图是对图3中过程的算法描述。左侧的函数表示FIL模块的算法。通过归纳学习矩阵P对特征矩阵进行投影(寻找潜在的公共特征空间)。引入邻接矩阵以缓解不同模态之间的“学习竞争”现象。右侧的函数分为三个部分:局部学习、全局特征学习,以及用于诊断任务的图特征提取学习部分。使用MLP(多层感知器)进行多通道信息的交互,多头注意机制增强全局信息学习。图最大池化提取每个隐藏图像中最具代表性的信息,以用于诊断任务。
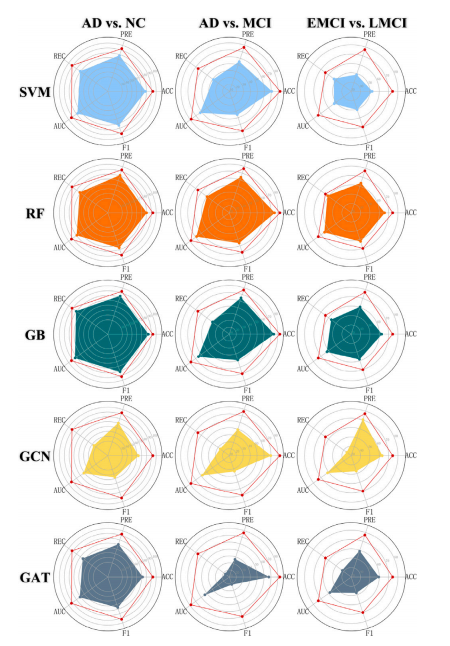
Fig. 5. Comparison of prediction performance between other models based on multimodal data and our model under three tasks. The red line in each radar sub-imagerepresents the performance of our model under the classification task, and the colorcoverage area in the sub-image is the performance of the corresponding model on theleft.
图5. 基于多模态数据的其他模型与我们的模型在三项任务下的预测性能比较。每个雷达子图中的红线表示我们模型在分类任务下的性能,子图中的彩色覆盖区域表示左侧对应模型的性能。
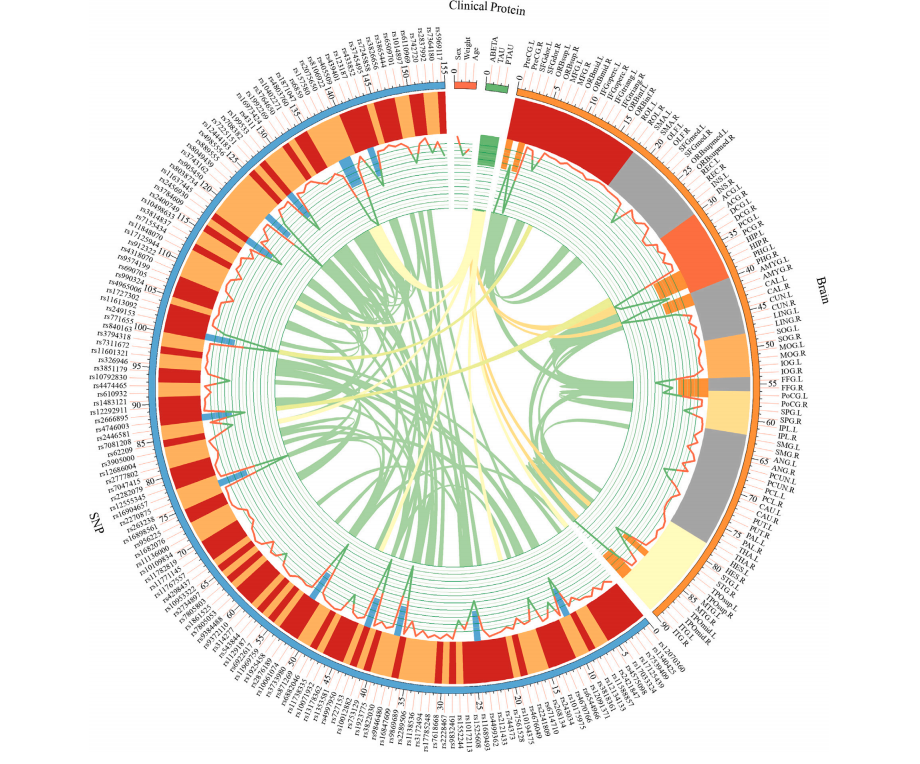
Fig. 6. Feature importance of Top30 risk features and interactive information visualization diagram. The outermost layer of the figure is the name of all features, from outside toinside, the location clustering information of all features (plots layer), the feature importance of risk features (line layer), and the interaction information layer between risk features(links layer). The innermost part is the feature association map contains five kinds of correlation information, including green: correlation information with modal features, orange:correlation information between the patient’s clinical features, and SNP, light orange: correlation information between protein and brain region, yellow: correlation informationbetween protein and SNP, light green, association information between SNP and brain region.
图6. 前30名风险特征的重要性和交互信息可视化图。图的最外层是所有特征的名称,从外到内依次为所有特征的位置聚类信息(图层)、风险特征的重要性(线条层)以及风险特征之间的交互信息层(链接层)。最内部是特征关联图,包含五种关联信息,包括:绿色表示与模态特征的关联信息,橙色表示患者临床特征与SNP之间的关联信息,浅橙色表示蛋白质与脑区之间的关联信息,黄色表示蛋白质与SNP之间的关联信息,浅绿色表示SNP与脑区之间的关联信息。
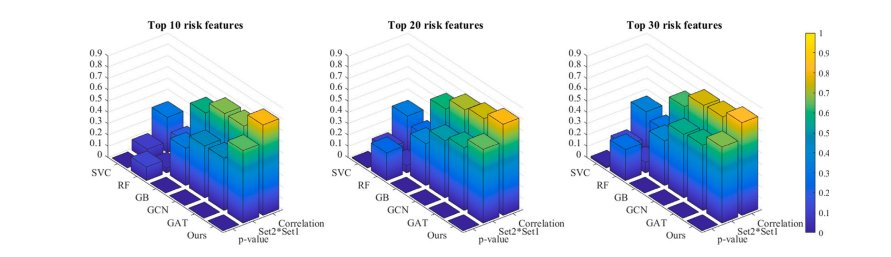
Fig. 7. Performance comparison chart of feature extraction by different methods. The correlation indicates the degree of correlation between the first pair of canonical variablesobtained by CCA of the TOP risk characteristics and AD. Set2*Set1 represents the proportion of AD information explained by the first pair of canonical variables obtained throughCCA of the TOP risk features we extracted. For example, the first pair of canonical variables of the TOP10 risk features explained 62.2% of the information of AD.
图7. 不同方法提取特征的性能比较图。相关性表示通过CCA(典型相关分析)获得的前一对典型变量之间的相关程度,这些变量来自于提取的TOP风险特征与阿尔茨海默病(AD)之间的关系。Set2*Set1表示我们提取的TOP风险特征通过CCA获得的前一对典型变量解释的AD信息比例。例如,TOP10风险特征的前一对典型变量解释了AD信息的62.2%。
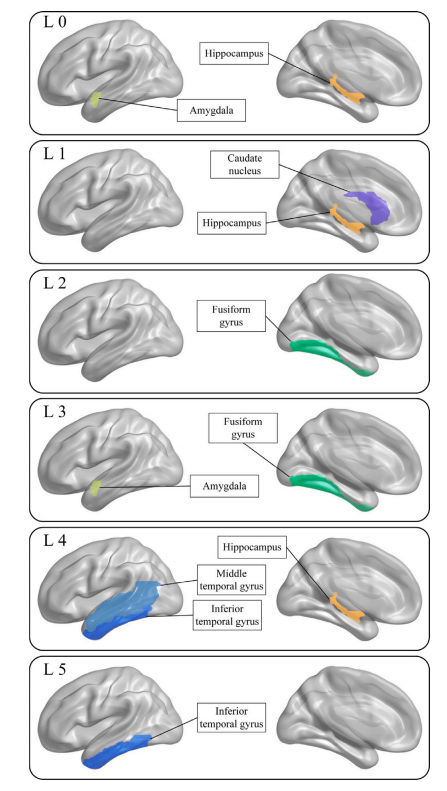
Fig. 8. Schematic diagram of risky brain region information from low to high levelsunder the individual perspective based on interpretability extraction (Subject ID136𝑆0426). The left side is the outer surface of the brain area, and the right sideis the inner section of the brain area. L represents the number of layers of the DMGNNmodule, for example, L0 represents the extracted risk brain area after the featureinformation is re-expressed by the FIL module.
图8. 基于可解释性提取的个体视角下,从低到高风险水平的大脑区域信息示意图(Subject ID 136𝑆0426)。左侧是大脑区域的外表面,右侧是大脑区域的内截面。L表示DMGNN模块的层数,例如,L0表示在特征信息通过FIL模块重新表达后提取的风险大脑区域
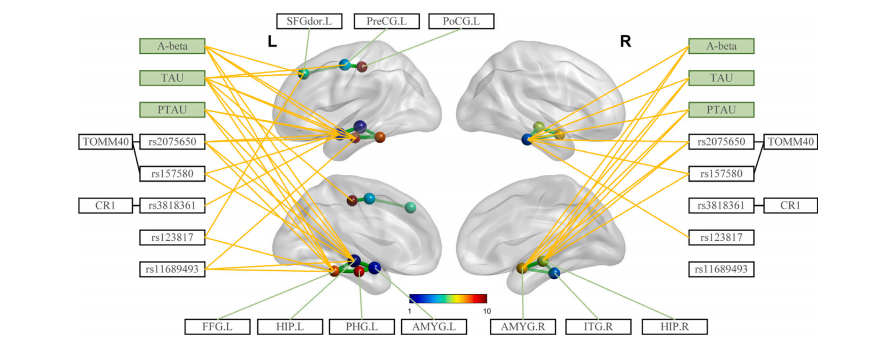
Fig. 9. Risk feature pairs between brain regions and other modal features under AD vs.NC prediction.
图9. 在AD与NC预测中,大脑区域与其他模态特征之间的风险特征对。
Table
表
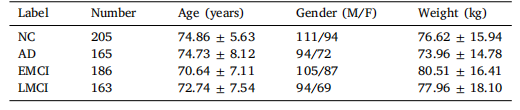
Table 1The demographic information of our dataset.
表1我们数据集的人口统计信息。
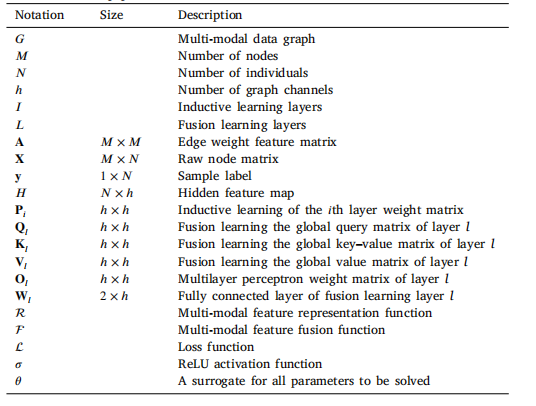
Table 2Notations in this paper.
表2本文中的符号说明。
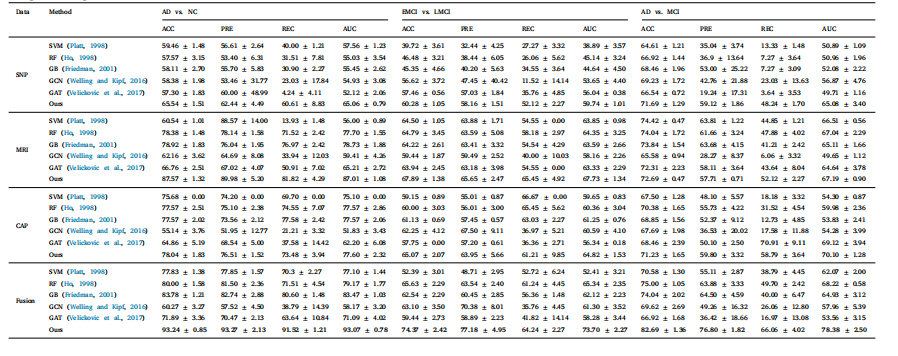
Table 3Comparative experiment results (%)
表3对比实验结果(%)
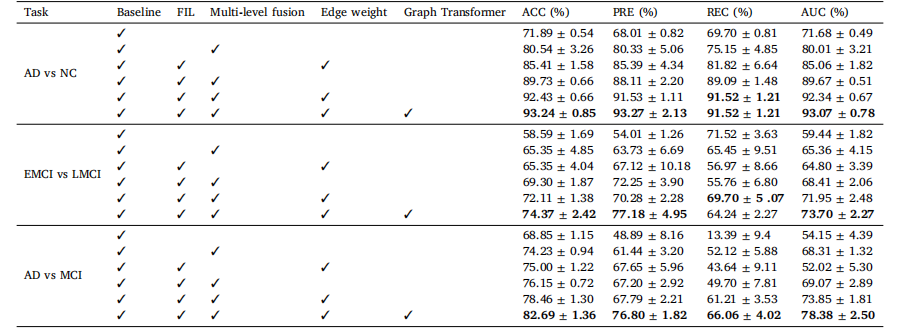
Table 4Ablation experiment results (%)
表4消融实验结果(%)

Table 5Significance analysis of different models under three tasks based on McNemar test.
表5基于McNemar检验的三项任务中不同模型的显著性分析。
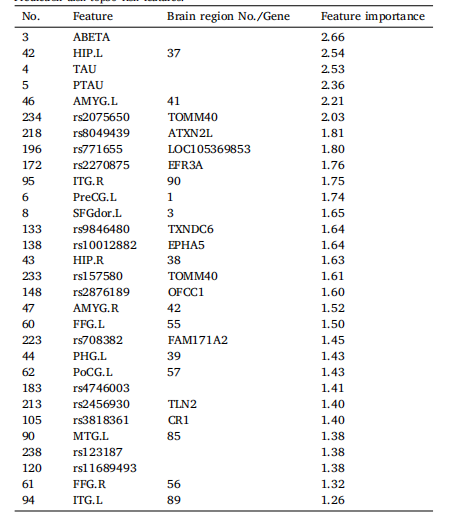
Table 6Prediction task top30 risk features.
表6 预测任务中排名前30的风险特征。
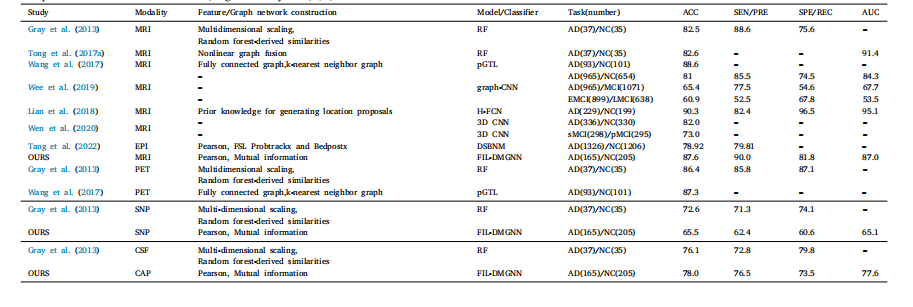
Table 7Comparison with the related methods (single-modality data) (%).
表7 与相关方法(单一模态数据)的比较(%)。
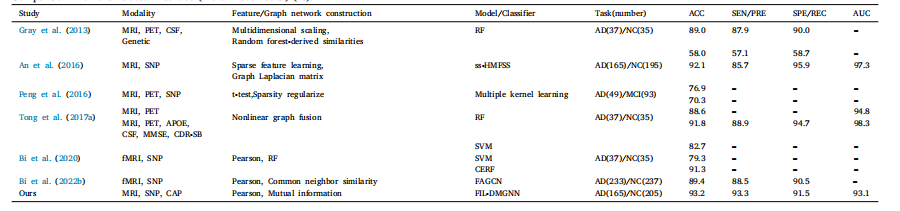
Table 8Comparison with the related methods (multi-modal data) (%).
表8 与相关方法(多模态数据)的比较(%)。

Table 9CCA of Risk Features Extracted From Prediction Model.
表9 从预测模型中提取的风险特征的典型相关分析(CCA)结果。