Title
题目
A Bayesian network for simultaneous keyframe and landmark detection inultrasonic cine
用于超声电影中同时检测关键帧和地标的贝叶斯网络
01
文献速递介绍
超声电影(Ultrasonic cine)是超声检查中常见的保存形式,允许实时回顾移动结构和流动模式,提供额外的诊断信息,并有助于识别微妙的异常(Mitchell等,2019)。在临床常规中,手动识别超声电影中的感兴趣事件并进一步进行测量和诊断是基本任务(Lang等,2015)。然而,即使对于有经验的专业人员来说,执行这项重复性任务也可能具有挑战性,特别是在处理复杂的解剖变异、图像质量欠佳或患者病例负担较重的情况下。因此,市场上对能够简化并提高这一关键过程准确性的自动化辅助工具的需求正在增加(Ouyang等,2020)。
自动识别超声心动图中的心动周期相位以测量各种心脏参数是一个重要的临床应用,尤其是在可能缺乏心电图数据的即时护理场景中,这可以减少观察者之间的变异性(Ciusdel等,2020;Zhang等,2023)。Dezaki等(2017)基于心脏体积的变化提出了结构化损失来识别舒张末期(ED)和收缩末期(ES)帧。Dai等(2023)利用心脏泵送的周期性设计了自监督正则化项,以便帧的特征表现出时间的周期性。然而,这些方法实现关键帧检测的前提是心脏体积在不同时间相位内单调变化。我们认为,依赖这一假设的模型可能缺乏对心律不齐患者的适应性,因此需要设计更为通用的关键帧检测模型。此外,对于定量描述任务,必须在超声电影中找到具有清晰目标形态的帧。因此,需要引入更多的目标特征,如纹理、时间或空间特征(Wang等,2022)。
Aastract
摘要
医学影像中准确的地标检测对于量化各种解剖结构以及辅助诊断和治疗计划至关重要。在超声电影中,地标检测通常与关键帧的识别相关联,这些关键帧代表特定事件的发生,例如在特定时间相位测量目标尺寸。现有方法主要将地标和关键帧检测作为独立任务处理,而未能利用它们之间的潜在关联。此外,由于超声成像的内在特性,这两项任务受制于观察者之间的变异性,从而可能导致更高的不确定性。在本文中,我们提出了一种贝叶斯网络,用于在超声电影中实现同时关键帧和地标检测,特别是在训练数据极为稀疏的情况下。我们采用了从粗到细的地标检测架构,并提出了一种自适应贝叶斯超图,用于在基于热图回归的结果上进行坐标精化。此外,我们提出了“顺序损失”来训练双向门控循环单元(GRU),以基于序列中的相对可能性识别关键帧。进一步地,为了利用这两项任务之间的潜在关联性,我们使用共享编码器来提取两项任务的特征,并通过时序和运动信息的交互来提高检测精度。在两个内部数据集(多视图经食道和经胸超声心动图)和一个公共数据集(经胸超声心动图)上的实验表明,我们的方法优于现有的最先进方法。左心耳、主动脉瓣环和左心室尺寸测量的平均绝对误差分别为2.40毫米、0.83毫米和1.63毫米。
Method
方法
We aim to identify the keyframe in the ultrasonic cine and locatelandmarks in the keyframe to measure anatomical parameters ultimately. For this multi-task model M, we represent the training data𝐷𝑡𝑟𝑎𝑖𝑛 = {(𝑋𝑖 , 𝐼𝑖 , 𝑌𝑖 )}𝑁𝑖=1, where 𝑋𝑖 = {𝑥1 …𝑥𝑇 } denotes the 𝑖th cinesample, T is the number of frames, 𝐼𝑖 denotes the index of keyframe,and 𝑌𝑖 denotes the landmark coordinates in the keyframe. We remarkthat the annotation of each training sample is sparse, containing onlyone keyframe and a set of landmark annotations on it. In the test phaseof this sparse problem, for an ultrasonic cine 𝑋𝑡 , the model predictsits keyframe and landmarks, i.e., (𝐼̂𝑡 ,𝑌𝑡 ) = 𝑀(𝑋𝑡 ). The overview ofproposed multi-task detection model is shown in Fig. 1 and furtherdetailed below.
我们旨在识别超声电影中的关键帧并定位关键帧中的地标,以最终测量解剖参数。对于这个多任务模型 M,我们将训练数据表示为 𝐷𝑡𝑟𝑎𝑖𝑛 = {(𝑋𝑖 , 𝐼𝑖 , 𝑌𝑖 )}𝑁𝑖=1,其中 𝑋𝑖 = {𝑥1 …𝑥𝑇 } 表示第 𝑖 个超声电影样本,T 是帧数,𝐼𝑖 表示关键帧的索引,𝑌𝑖 表示关键帧中地标的坐标。我们指出,每个训练样本的标注是稀疏的,只包含一个关键帧及其上的一组地标标注。在这个稀疏问题的测试阶段,对于一个超声电影 𝑋𝑡 ,模型预测其关键帧和地标,即 (𝐼̂𝑡 ,𝑌𝑡 ) = 𝑀(𝑋𝑡 )。所提出的多任务检测模型的概述如图1所示,具体细节如下。
Conclusion
结论
In this article, we propose a Bayesian multi-task network to tacklethe challenges of keyframe and landmark detection in ultrasonic cinewith high uncertainty. Following the coarse-to-fine detection architecture, we propose ABHG to explicitly model the structural relationshipof landmarks and fine-tune the prediction of heatmap-based regression. To overcome the potential limitations arising from informationpropagation and overfitting due to the scale of the hypergraph, weimplement adaptive expansion within the 8-neighborhood directionsof hyper-nodes. Furthermore, we establish a synergistic relationshipbetween the receptive field of hyper-nodes and the landmark offset andintroduce MC Dropout during testing to further improve the landmarklocalization accuracy.
在本文中,我们提出了一种贝叶斯多任务网络,以应对超声电影中关键帧和地标检测所面临的高不确定性挑战。遵循从粗到细的检测架构,我们提出了ABHG,以明确建模地标的结构关系,并对基于热图回归的预测进行微调。为了解决由于超图规模导致的信息传播和过拟合的潜在限制,我们在超节点的8邻域方向内实现了自适应扩展。此外,我们在超节点的感受野与地标偏移之间建立了协同关系,并在测试期间引入了MC Dropout,以进一步提高地标定位的准确性。
Figure
图
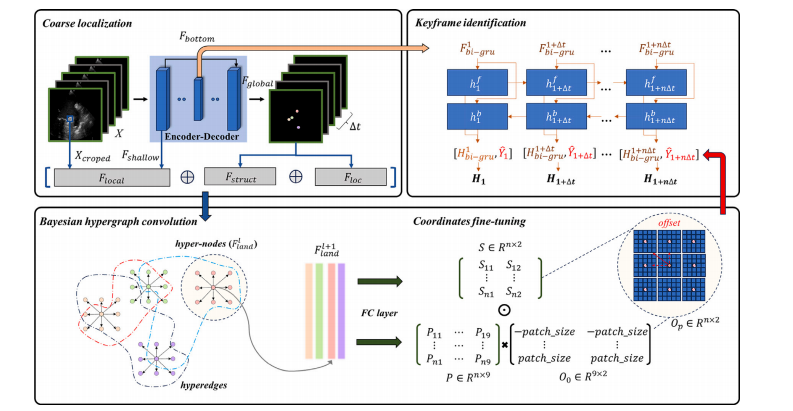
Fig. 1. The proposed architecture simultaneously identifies landmarks and keyframe for ultrasonic cine. First, a shared encoder–decoder is utilized to extract the features of eachframe and perform coarse localization of landmarks. Next, the Bayesian hypergraph is adaptively constructed by utilizing the local information of the original image, the shallowfeatures of the encoder, the structural relationships between landmarks and the coarse locations as the node features. To achieve accurate coordinate fine-tuning, we use Bayesianhypergraph convolution to establish hypernode connections within 8-neighborhoods and predict the direction and scale of the offset. Finally, the predicted landmark coordinatesand coder–decoder global features are fed into a Bi-GRU to obtain the likelihoods of keyframes.
图1. 提出的架构能够同时识别超声电影中的地标和关键帧。首先,使用共享的编码器–解码器来提取每一帧的特征,并对地标进行粗略定位。接下来,利用原始图像的局部信息、编码器的浅层特征、地标之间的结构关系和粗略定位结果作为节点特征,自适应地构建贝叶斯超图。为了实现精确的坐标微调,我们使用贝叶斯超图卷积在8邻域内建立超节点连接,并预测偏移的方向和尺度。最后,将预测的地标坐标和编码器–解码器的全局特征输入到双向门控循环单元(Bi-GRU)中,以获得关键帧的可能性。
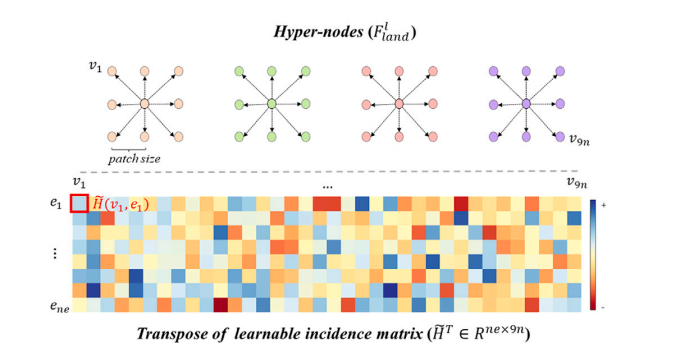
Fig. 2. The 8-neighborhood hyper-nodes and the incidence matrix of ABHG.
图2. ABHG(自适应贝叶斯超图)的8邻域超节点和关联矩阵。
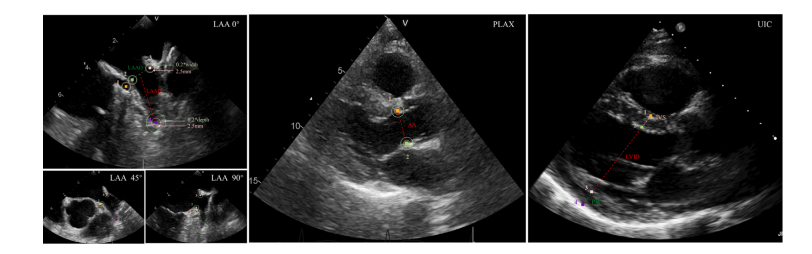
Fig. 3. In the two in-house datasets, LAA and PLAX, we annotate four anatomical landmarks on the left atrial appendage of multi-view transesophageal echocardiography: (1) theleft circumflex branch of the coronary artery, (2) the inner wall of the left auricular wall, (3) a landmark located 2 mm below the opening of the left upper pulmonary vein onthe opposite side, and (4) the tip of the left atrial appendage. Additionally, two anatomical landmarks, namely (1) the anterior inner edge and (2) the posterior inner edge, wereidentified in the parasternal long-axis view of transthoracic echocardiography. In the public dataset UIC, four landmarks are annotated: the top and bottom of the interventricularseptum, as well as the top and bottom of the posterior wall.
图3. 在两个内部数据集LAA和PLAX中,我们在多视图经食道超声心动图的左心耳上标注了四个解剖地标:(1)冠状动脉左旋支,(2)左耳壁内壁,(3)位于左上肺静脉开口对侧下方2毫米处的一个地标,以及(4)左心耳尖端。此外,在经胸超声心动图的胸骨旁长轴视图中识别了两个解剖地标,分别是:(1)前内缘和(2)后内缘。在公开数据集UIC中,标注了四个地标:室间隔的顶部和底部,以及后壁的顶部和底部。
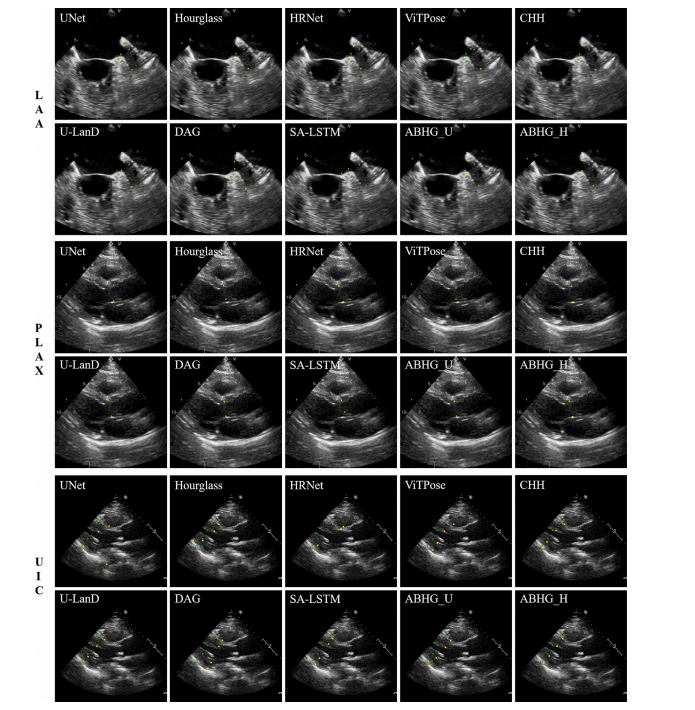
Fig. 4. Qualitative results for locating the landmarks of the left atrial appendage, aortic annulus, and left ventricle. Green points indicate ground truth, while yellow points markthe predictions.
图4. 左心耳、主动脉瓣环和左心室地标定位的定性结果。绿色点表示真实值,黄色点表示预测值。
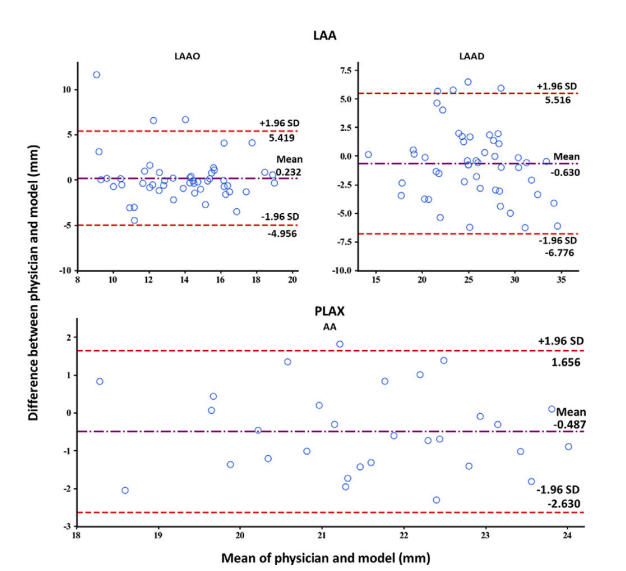
Fig. 5. Agreement between two measurements (Bland–Altman plot).
图5. 两次测量之间的一致性(Bland-Altman图)。
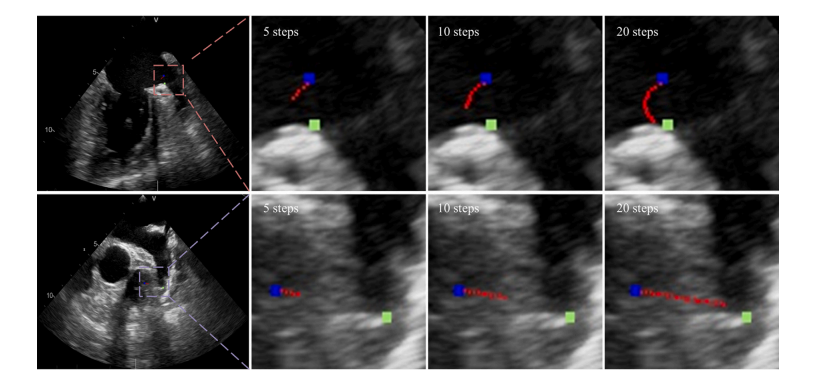
Fig. 6. Landmark trajectories over 20 iterations of ABHG. A random initial position is marked in blue, the ground truth in green, and the updating trajectory in red.
图6. ABHG在20次迭代中的地标轨迹。随机初始位置以蓝色标记,真实值以绿色标记,更新轨迹以红色标记。
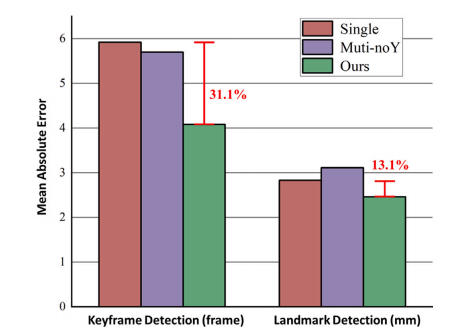
Fig. 7. Effectiveness assessment of multi-task learning. The bar chart shows thecomparison of the mean absolute errors among the single-task, simple multi-task, andproposed multi-task models on the two detection tasks.
图7. 多任务学习效果评估。柱状图显示了在两个检测任务中,单任务、简单多任务和所提出的多任务模型的平均绝对误差比较。
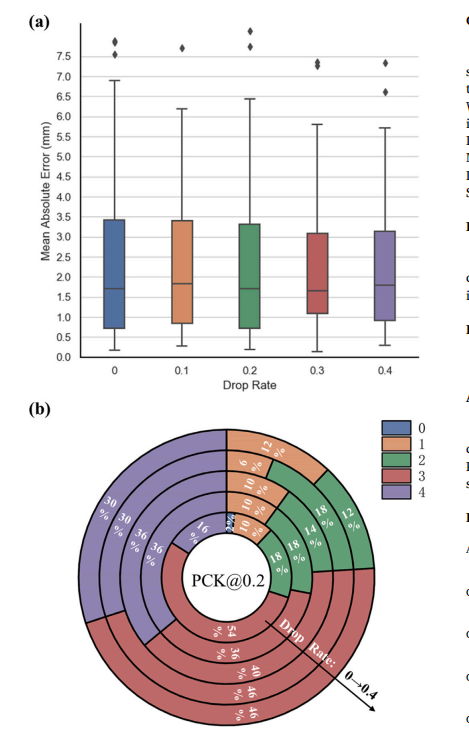
Fig. 8. Effectiveness assessment of Monte Carlo Dropout. (a) Illustration of the MAE formeasurements of left atrial appendage at different drop rates. (b) The doughnut chartshows the proportion of samples correctly detecting varying numbers of landmarksat different drop rates using the PCK@0.2 metric. Different colors indicate differentproportions of correctly detected landmarks. 0 indicates that all landmark coordinateerrors are not within tolerance, while 4 indicates that all four landmark coordinateerrors are within tolerance.
图8. 蒙特卡罗Dropout效果评估。(a) 不同Dropout率下左心耳测量的平均绝对误差(MAE)示意图。(b) 环形图显示在不同Dropout率下使用PCK@0.2指标正确检测到不同数量地标的样本比例。不同颜色表示正确检测到地标的不同比例。0表示所有地标坐标误差均不在容差范围内,而4表示所有四个地标坐标误差均在容差范围内。-
Table
表
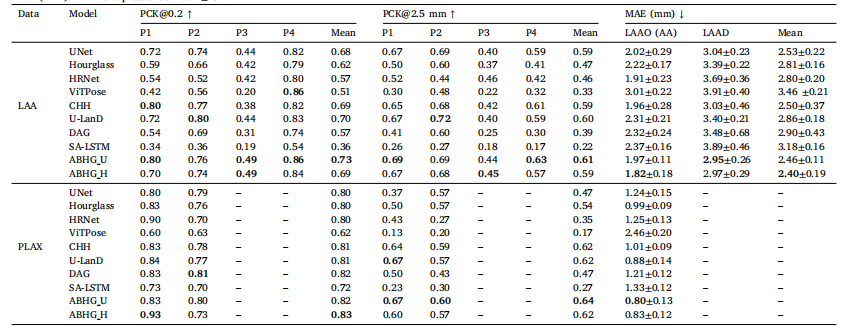
Table 1Comparison of Landmark Detection with state-of-the-art methods on LAA and PLAX datasets using evaluation metrics of Percentage of Correct Keypoints (PCK) and Mean AbsoluteError (MAE). MAE is expressed as mean ± SD.
表1 在LAA和PLAX数据集上使用正确关键点百分比(PCK)和平均绝对误差(MAE)评价指标与最先进方法进行的地标检测比较。MAE表示为平均值 ± 标准差(SD)。
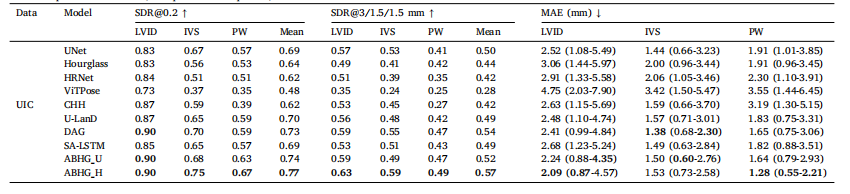
Table 2Comparison of Landmark Detection with state-of-the-art methods on the UIC dataset and by evaluation metrics of Success Detection Rate (SDR) and Mean Absolute Error (MAE).MAE is expressed as median (first quartile-third quartile).
表2在UIC数据集上使用成功检测率(SDR)和平均绝对误差(MAE)评价指标与最先进方法进行的地标检测比较。MAE表示为中位数(第一四分位数-第三四分位数)
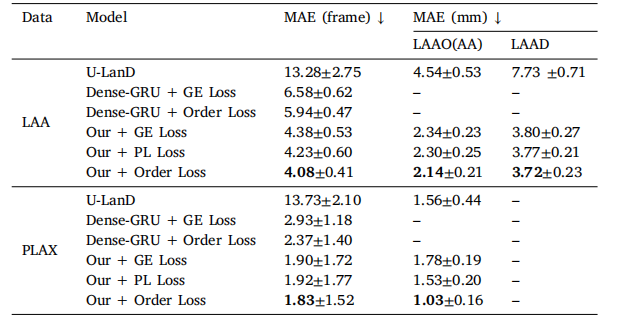
Table 3Comparison of Keyframe Detection with state-of-the-art methods on LAA and PLAXdatasets using evaluation metrics of Mean Absolute Error (MAE). MAE is expressed asmean ± SD.
表3 在LAA和PLAX数据集上使用平均绝对误差(MAE)评价指标与最先进方法进行关键帧检测的比较。MAE表示为平均值 ± 标准差(SD)。
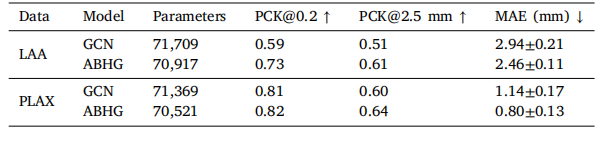
Table 4Comparison of ABHG and GCN for Landmark Detection on LAA and PLAX Datasets.MAE is expressed as mean ±SD.
表4 ABHG与GCN在LAA和PLAX数据集上进行地标检测的比较。MAE表示为平均值 ± 标准差(SD)。

Table 5Accuracy of keyframe detection under varying degrees of landmark location errors.MAE is expressed as mean ±SD.
表5 不同地标定位误差下的关键帧检测准确性。MAE表示为平均值 ± 标准差(SD)。
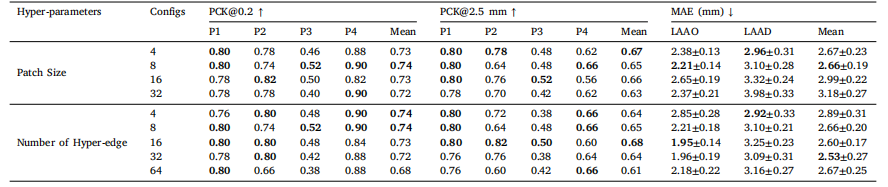
Table 6Hyper-parameters impact assessment on landmark detection model on LAA dataset using evaluation metrics of Percentage of Correct Keypoints (PCK) and Mean Absolute Error(MAE). MAE is expressed as mean ±SD.
表6 超参数对LAA数据集地标检测模型的影响评估,使用正确关键点百分比(PCK)和平均绝对误差(MAE)作为评价指标。MAE表示为平均值 ± 标准差(SD)。