Title
题目
Pathological Asymmetry-Guided Progressive Learning for Acute Ischemic Stroke Infarct Segmentation
病理性不对称引导的渐进学习用于急性缺血性脑卒中梗死分割
01
文献速递介绍
中风已经成为第二大致命疾病,大约70%的中风是缺血性的。众所周知,梗死范围与急性缺血性脑卒中(AIS)患者的功能结果密切相关 。计算机断层扫描(CT)和磁共振成像(MRI)是估计梗死范围的两种主要方式。MRI相对耗时长、设备不易获得且费用昂贵,因此在患者入院时,CT成为首选影像学检查 。然而,手动评估梗死缺乏效率和准确性。因此,在中风的诊断和治疗过程中,急需计算机辅助的精确量化估计AIS梗死在非对称CT上的应用。
,AIS早期阶段在非对称CT上的缺血组织表现相当不明显,这导致AIS梗死在非对称CT上的分割依然棘手。特别是如图1.(a)所示,由缺血组织引起的病理性低密度变化微妙,并且容易被正常生理变化或健康脑组织混淆。为了区分梗死区域和正常脑组织,在临床实践中放射科医师通常利用双侧半球的对称比较。如图1.(b)左侧所示,他们首先找到理想的中矢状线(MSL),然后沿着MSL比较双侧半球的差异,以识别病理性不对称性进一步定位梗死区域。
Abstract
摘要
量化梗死估计在急性缺血性脑卒中(AIS)患者的诊断、治疗和预后中至关重要。由于缺血组织的早期变化细微且易受正常脑组织干扰,因此仍然是一项非常具有挑战性的任务。然而,现有方法通常忽视或混淆由内在和病理变化引起的不同类型解剖不对称对分割的贡献。此外,低效的领域知识利用导致AIS梗死分割的误分割。受此启发,我们提出了一种病理性不对称引导的渐进学习(PAPL)方法用于AIS梗死分割。PAPL模仿人类观察到的分步学习模式,包括三个渐进阶段:知识准备阶段、正式学习阶段和检查改进阶段。首先,知识准备阶段积累了梗死分割任务的准备领域知识,帮助学习特定于领域的知识表示,通过构建对比学习任务增强病理性不对称的区分能力。然后,正式学习阶段通过学习的知识表示有效地进行端到端训练,其中设计的特征补偿模块(FCM)可以利用体积医学图像中相邻切片之间的解剖相似性来帮助聚合丰富的解剖上下文信息。最后,检查改进阶段鼓励从前一阶段改进梗死预测,其中提出的感知细化策略(RPRS)通过自适应区域收缩和扩展进一步利用双侧差异比较来纠正误分割的梗死区域。在公共和内部NCCT数据集上的广泛实验表明,所提出的PAPL方法优越性,有望帮助改进脑卒中评估和治疗。
Method
方法
This section introduces the proposed PAPL for AIS infarctsegmentation. Firstly, Fig.2 describes the overall architecture.Then, three progressive stages of the PAPL in Fig.2 are specifiedsequentially in subsections III.C-E.
这一部分介绍了提出的用于AIS梗死分割的PAPL方法。首先,图2描述了整体架构。然后,PAPL的三个渐进阶段在图2中依次在III.C至III.E小节中详细说明。
Conclusion
结论
The changes of ischemic brain tissue on NCCT are subtleand difficult to identify in the early stage of AIS. Therefore,it remains a very challenging task for early AIS infarctsegmentation on NCCT. Current methods generally ignore orconfuse the different contributions of intrinsic and pathologicalasymmetries in the bilateral hemisphere differences to AISinfarct segmentation when integrating this domain knowledgeinto DL methods. Inspired by this, we propose a pathological asymmetry-guided progressive learning (PAPL) methodfor facilitating AIS infarct segmentation. PAPL mimics thestep-by-step learning patterns observed in humans, includingthree progressive stages: knowledge preparation stage, formallearning stage, and examination improvement stage. Basedon this preview-learn-correct progressive strategy, we canachieve further better AIS infarct segmentation. Extensiveexperiments on the public AISD and in-house XWHD datasetsdemonstrated the superiority of the proposed PAPL, which ispromising to help better evaluate brain tissue status and predictprognosis. Besides, our method can be easily integrated intoother segmentation backbones, making it highly applicable.
缺血性脑组织在非对称CT上的变化微妙且难以在急性缺血性脑卒中(AIS)的早期阶段识别。因此,在非对称CT上进行早期AIS梗死分割仍然是一项极具挑战性的任务。当前方法通常在将这种领域知识整合到深度学习方法中时,忽视或混淆双侧半球的内在和病理性不对称性对AIS梗死分割的不同贡献。受此启发,我们提出了一种病理性不对称引导的渐进学习(PAPL)方法,以促进AIS梗死分割。PAPL模仿了人类观察到的逐步学习模式,包括知识准备阶段、正式学习阶段和检查改进阶段。基于这种预览-学习-校正的渐进策略,我们可以实现更好的AIS梗死分割效果。在公共AISD和内部XWHD数据集上进行的大量实验显示了提出的PAPL方法的优越性,有望帮助更好地评估脑组织状态并预测预后。此外,我们的方法可以轻松集成到其他分割主干中,具有广泛的适用性。
Results
结果
A. Segmentation Comparison With the State-of-the-art MethodsTable II gives the quantitative comparison of the proposedPAPL and different SOTA segmentation methods on theAISD and XWHD datasets. The best results are highlightedin boldface. General medical image segmentation methodsincluding U-Net, CENet, Attention U-Net andU-Net++ are difficult to capture subtle hypo-attenuationchanges caused by the AIS infarct, which only achieve poorsegmentation performance. TransU-Net can provide longdistance dependent information to help perceive the bilateraldifference of the image. Therefore, the TransU-Net [46] canachieve better performance compared to the aforementionedmethods. The ablation studies for the aligning process showthat these segmentation methods including U-Net , CENet and U-Net++ and TransU-Net can gain furtherperformance improvement when the NCCT scans are alignedto standard symmetric space. One reason is that the aligningprocess reduces the differences in the spatial distributionbetween training images and test images and makes the spatialdistributions between them closer. Besides, these stroke lesionspecific segmentation methods including ISP-Net , XNet and CLCI-Net can more effectively extract thehypo-attenuation features of AIS infarcts and achieve bettersegmentation performance. Especially, these brain symmetricmodeling-based methods including SEAN, ADN [5], SANNet and IS-Net can gain further improvement byincorporating the domain knowledge of the brain hemispheresinto the deep networks. For our PAPL, it can suppressundesirable effects for the infarct segmentation from theintrinsic asymmetries caused by normal physiological changes.Therefore, the proposed PAPL achieved the best segmentationperformance of 0.5468 and 0.4899 DSCs on the AISD andXWHD datasets respectively.
A. 与最先进方法的分割比较 表II 给出了所提出的PAPL方法与AISD和XWHD数据集上不同最先进分割方法的定量比较。最佳结果以粗体突出显示。包括U-Net 、CENet 、Attention U-Net 和U-Net++在内的一般医学图像分割方法难以捕捉由AIS梗死引起的微妙低密度变化,因此仅实现了较差的分割性能。TransU-Net 可以提供远距离依赖信息,有助于感知图像的双侧差异。因此,与上述方法相比,TransU-Net 可以实现更好的性能。校准过程的剔除研究表明,这些包括U-Net、CENet 、U-Net++ 和TransU-Net 的分割方法在将非对称CT扫描校准到标准对称空间时可以进一步提高性能。一个原因是校准过程减少了训练图像与测试图像之间的空间分布差异,并使它们之间的空间分布更接近。此外,这些特定于中风病变的分割方法,包括ISP-Net 、XNet 和CLCI-Net ,可以更有效地提取AIS梗死的低密度特征,并实现更好的分割性能。特别是,这些基于大脑对称建模的方法,如SEAN 、ADN、SANNet 和IS-Net,通过将大脑半球的领域知识整合到深度网络中,可以进一步提高性能。对于我们的PAPL方法,它可以抑制由正常生理变化引起的内在不对称性对梗死分割产生的不良影响。因此,所提出的PAPL在AISD和XWHD数据集上分别实现了最佳的分割性能,分别为0.5468和0.4899的DSC(Dice相似系数)。
Figure
图
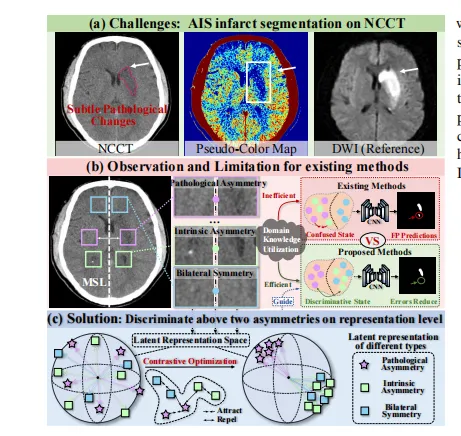
Fig. 1: (a) The AIS infarct segmentation on NCCT is avery challenging task due to subtle pathological changes. (b)The limitation of existing methods is that they commonlyignore or confuse the different contributions of intrinsic andpathological asymmetries in the bilateral hemisphere differencesto segmentation. © Therefore, we propose to discriminate thesetwo asymmetries on the representation level to fully leveragethe domain knowledge for facilitating the infarct segmentation.
Fig. 1: (a) 急性缺血性脑卒中(AIS)在非对称CT上的梗死分割是一项非常具有挑战性的任务,由于病理变化微妙。(b) 现有方法的局限性在于它们通常忽视或混淆双侧半球差异中内在和病理性不对称性的不同贡献对分割的影响。© 因此,我们提出在表示层面区分这两种不对称性,以充分利用领域知识促进梗死分割。
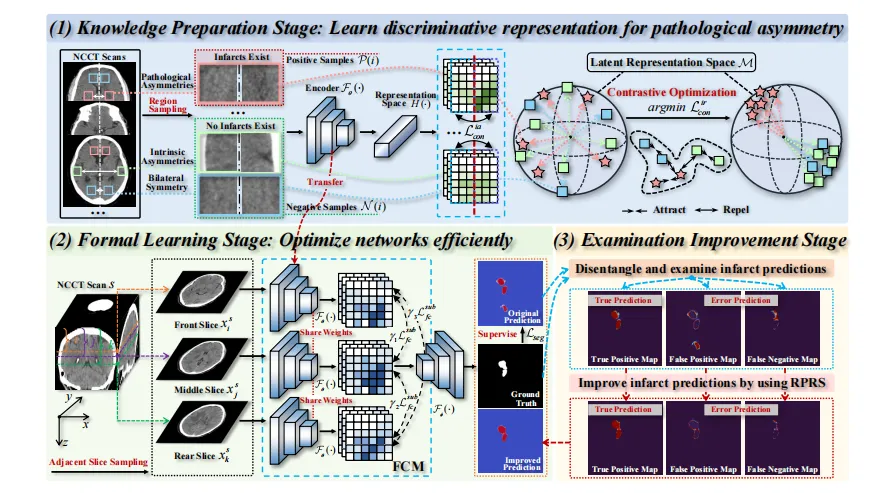
Fig. 2: The overall illustration of the proposed pathological asymmetry-guided progressive learning (PAPL), including threeprogressive stages: (1) knowledge preparation stage, (2) formal learning stage, and (3) examination improvement stage.
Fig. 2: 所提出的病理性不对称引导的渐进学习(PAPL)的总体示意图,包括三个渐进阶段:(1) 知识准备阶段,(2) 正式学习阶段,和 (3) 检查改进阶段。
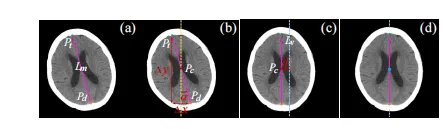
Fig. 3: The illustration of the symmetry space transformationof the NCCT Scans. By the affine transformation includingrotation (b) and translation ©, the given slice can be transformed from the unaligned state (a) to the aligned state (d).
Fig. 3: 非对称CT扫描的对称空间转换示意图。通过包括旋转(b)和平移(c)的仿射变换,给定的切片可以从未对齐状态(a)转换为对齐状态(d)。
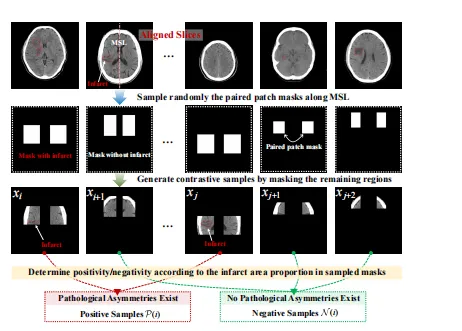
Fig. 4: The construction of positive and negative samples by the designed region mask sampling strategy.
Fig. 4: 通过设计的区域掩码采样策略构建正负样本的示意图。
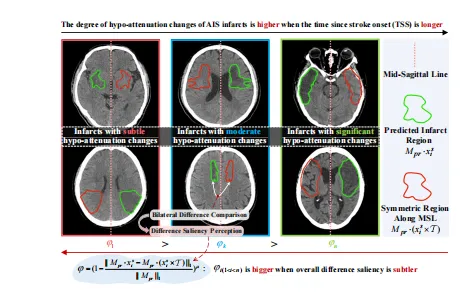
Fig. 5: Evaluating the difference saliency degree φ in infarct related bilateral region intensity comparison.
Fig. 5: 评估梗死相关双侧区域强度比较中的差异显著度φ。
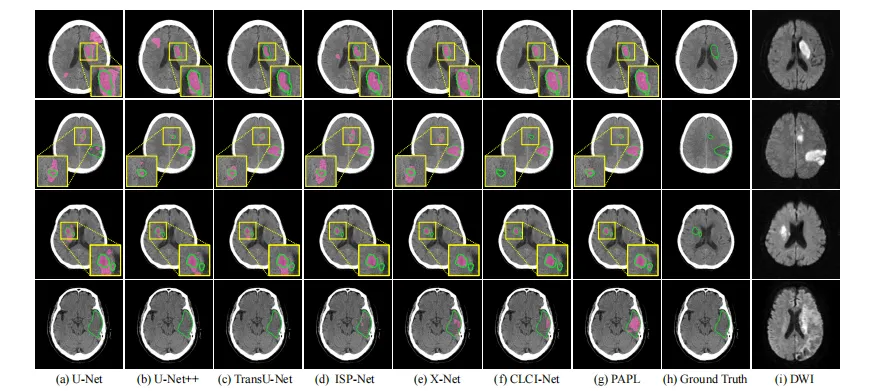
Fig. 6: Visualizing segmentation comparison on AISD dataset.
Fig. 6: 在AISD数据集上可视化分割比较。
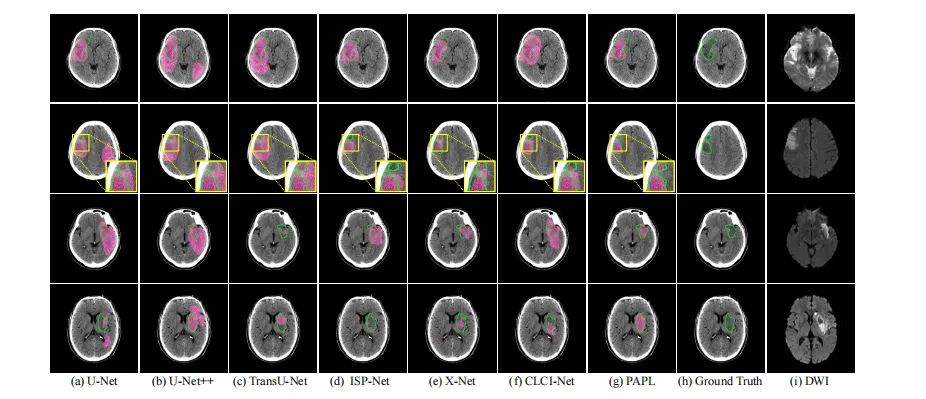
Fig. 7: Visualizing segmentation comparison on XWHD dataset.
Fig. 7: 在XWHD数据集上可视化分割比较。
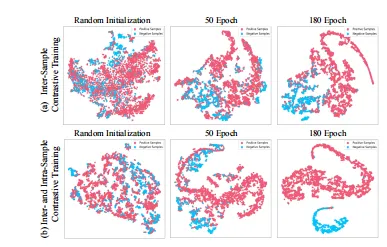
Fig. 8: Visualizing learned knowledge representations in knowledge preparation stage. Red and blue points are embeddings ofthe positive and negative samples respectively after applyingt-SNE
Fig. 8: 可视化知识准备阶段中学习到的知识表示。红色和蓝色点分别是应用t-SNE后的正样本和负样本的嵌入。
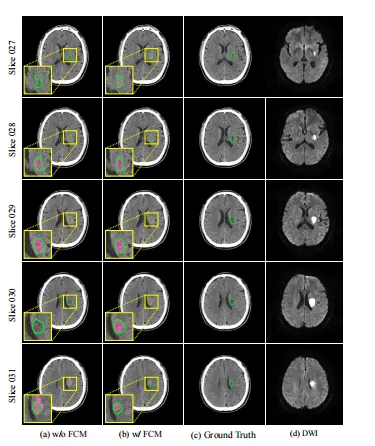
Fig. 9: Visualizing segmentation for the adjacent slices.
Fig. 9: 可视化相邻切片的分割结果。
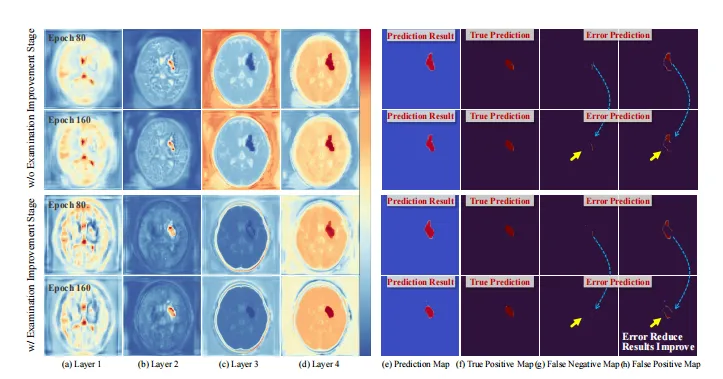
Fig. 10: Visualizing feature maps and predicted results from different training epochs.
Fig. 10: 可视化不同训练时期的特征图和预测结果。
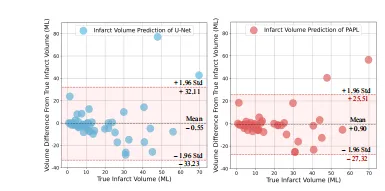
Fig. 11: The Bland-Altman plot of backbone and proposed methods for small-volume infarcts.
Fig. 11: 小体积梗死区域的主干网络和提出方法的 Bland-Altman 图。
Table
表
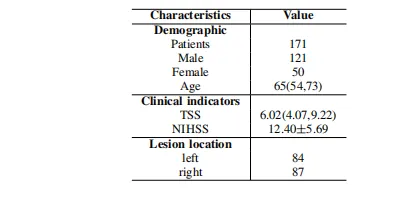
TABLE I Statistical characteristics distribution of AIS patients on XWHD. The Age and TSS are reported in the form of the (median,quartile). The NIHSS is reported in the form of the mean±standard deviation.
表格 I 急性缺血性脑卒中(AIS)患者在XWHD上的统计特征分布。年龄和TSS以(中位数,四分位数)的形式报告。NIHSS以均值±标准差的形式报告。
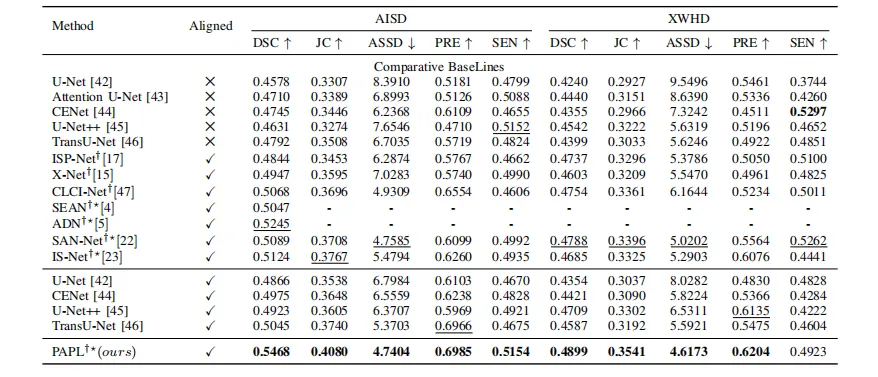
TABLE II Performance comparison between other methods and our PAPL on the AISD and XWHD datasets. † means stroke lesion-specific segmentation methods and means brain symmetric modeling-based methods. The best and second performances are in bold and underlined respectively.
表格 II 其他方法与我们的PAPL在AISD和XWHD数据集上的性能比较。† 表示中风病变特定分割方法, 表示基于脑对称建模的方法。最佳和次佳性能分别用粗体和下划线标出。
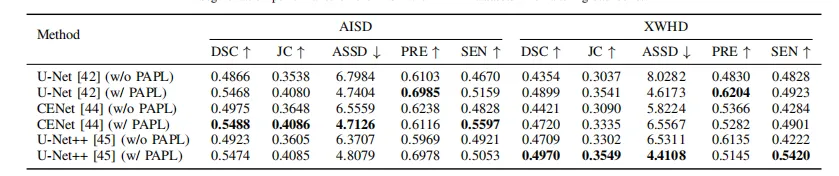
TABLE III Segmentation performance on the AISD and XWHD datasets when altering backbones.
表格III 在改变主干网络时在AISD和XWHD数据集上的分割性能。
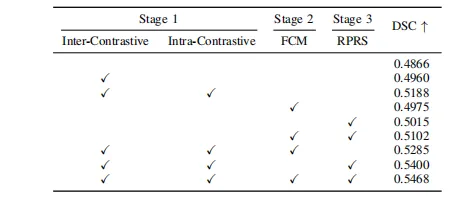
TABLE IV Ablation analysis of different submodules on the AISD dataset.
表格 IV 在AISD数据集上不同子模块的剔除分析。

TABLE V Performance comparison between different methods for small (≤70 ml) and big volume (>70 ml) infarcts on the AISD dataset.
表格 V 急性缺血性脑卒中(AISD)数据集中小(≤70 ml)和大(>70 ml)梗死区域不同方法的性能比较
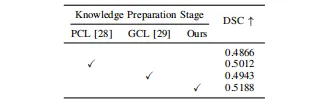
TABLE VISegmentation performance when altering different self-supervised CL methods in Knowledge Preparation Stage.
表格 VI 在知识准备阶段使用不同自监督对比学习(CL)方法时的分割性能。
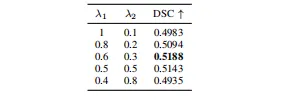
TABLE VIIAblation analysis of weight parameter λ1 and λ2 settings.
表格 VII权重参数λ₁和λ₂设置的剔除分析。
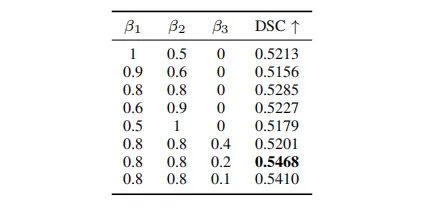
TABLE VIIIAblation analysis of weight parameter β1, β2 and β3 settings
表格 VIII 权重参数β₁、β₂和β₃设置的剔除分析