Title
题目
Pan-cancer integrative histology-genomic analysis via multimodal deep learning
多模式深度学习实现的全癌症整合组织学-基因组学分析
01
文献速递介绍
癌症的定义包括肿瘤和组织微环境中标志性的组织病理学、基因组学和转录组学的异质性,这些异质性导致治疗反应率和患者预后的可变性(Marusyk等,2012年)。目前许多癌症类型的临床范式涉及对组织病理学特征的手动评估,如肿瘤浸润、无形态、坏死和有丝分裂,然后将这些特征用作分级和分期的标准,以将患者分成不同的风险组进行治疗决策(Amin等人,2017年)。例如,在肿瘤、淋巴结和转移(TNM)分期系统中,原发肿瘤根据肿瘤的严重程度(如大小、生长、非典型性)被分类为不同的分期,然后用于治疗规划、手术资格、放射剂量和其他治疗决策(Marusyk等,2012年;Chang等,2013年;Heindl等,2015年;Kather等,2018年;Tarantino等,2021年)。
然而,组织病理学特征的主观解释已经证明存在着很大的观察者之间和观察者内部的变异性,并且同一等级或分期的患者仍然在预后上有显著的变异性。尽管已经建立了许多组织病理学生物标志物用于诊断任务,但大多数都是基于肿瘤细胞的形态和位置,缺乏对肿瘤微环境中的基质、肿瘤和免疫细胞的空间组织如何影响患者风险的细致理解(Marusyk等,2012年;Chang等,2013年;Heindl等,2015年;Kather等,2018年;Tarantino等,2021年)。
最近在计算病理学中取得的深度学习的进步使得可以利用全切片图像(WSIs)进行癌症的自动化诊断和肿瘤微环境中形态表型的定量化。利用弱监督学习,切片级别的临床注释可以用来指导深度学习算法重现常规诊断任务,如癌症检测、分级和亚型划分(Campanella等,2019年;Lu等,2021年)。尽管这种算法在狭义问题上可以达到与人类专家相当的性能,但对新型预后形态特征的量化受到限制,因为使用主观人类注释进行训练可能无法提取到迄今为止未被识别的特性,这些特性可以用于改善患者预后评估(Echle等,2021年)。为了捕获在常规临床工作流程中未提取的更客观和预后性形态特征,最近的基于深度学习的方法提出使用基于结果的标签,如无疾病生存和总生存时间作为地面真值进行监督(Harder等,2019年;Courtiol等,2019年;Kather等,2019a、2019b年;Kulkarni等,2020年;Wuclzyn等,2020年、2021年)。事实上,最近的研究表明,利用深度学习进行自动生物标志物发现的潜力巨大,并可以发现新型和预后性形态决定因素(Beck等,2011年;Echle等,2021年;Diao等,2021年)。
Abstract
摘要
The rapidly emerging field of computational pathology has demonstrated promise in developing objective prognostic models from histology images. However, most prognostic models are either based on histology orgenomics alone and do not address how these data sources can be integrated to develop joint image-omicprognostic models. Additionally, identifying explainable morphological and molecular descriptors from thesemodels that govern such prognosis is of interest. We use multimodal deep learning to jointly examine pathologywhole-slide images and molecular profile data from 14 cancer types. Our weakly supervised, multimodal deeplearning algorithm is able to fuse these heterogeneous modalities to predict outcomes and discover prognosticfeatures that correlate with poor and favorable outcomes. We present all analyses for morphological and molecular correlates of patient prognosis across the 14 cancer types at both a disease and a patient level in an interactive open-access database to allow for further exploration, biomarker discovery, and feature assessment.
计算病理学这一迅速发展的领域已经展示了从组织学图像中开发客观预后模型的潜力。然而,大多数预后模型要么基于仅仅组织学,要么基于基因组学,而没有解决如何整合这些数据来源以开发联合图像-基因组预后模型的问题。此外,从这些模型中识别能够决定预后的可解释形态和分子描述符也是一个感兴趣的问题。我们使用多模态深度学习来共同检验14种癌症类型的病理学全切片图像和分子谱数据。我们的弱监督、多模态深度学习算法能够融合这些异构模态来预测结果,并发现与不良和有利结果相关的预后特征。我们在一个交互式开放获取的数据库中呈现了对14种癌症类型的病人预后的形态和分子相关性的所有分析,以允许进一步的探索、生物标志物发现和特征评估。
Results
结果
Deep-learning-based multimodal integrationIn order to address the challenges in developing joint imageomic biomarkers that can be used for cancer prognosis, wepropose a deep-learning-based multimodal fusion (MMF) algorithm that uses both H&E WSIs and molecular profile features(mutation status, copy-number variation, RNA sequencing[RNA-seq] expression) to measure and explain relative risk ofcancer death (Figure 1A). Our multimodal network is capableof not only integrating these two modalities in weakly supervised learning tasks such as survival-outcome prediction butalso explaining how histopathology features, molecular features, and their interactions correlate with low- and high-riskpatients (Figures 1B–1E). After risk assessment within a patientcohort, our network uses both attention- and attribution-basedinterpretability as an untargeted approach for estimating prognostic markers across all patients (Figures 1B–1F). Our studyuses 6,592 gigapixel WSIs from 5,720 patient samples across14 cancer types from the TCGA (Table S1). For each cancertype, we trained our multimodal model in a 5-fold cross-validation using our weakly supervised paradigm and conductedablation analyses comparing the performance between unimodal and multimodal prognostic models. Following training andmodel evaluation, we conducted extensive analyses on theinterpretability of our networks, investigating local- andglobal-level image-omic explanations for each cancer type,quantifying the tissue microarchitecture corresponding relevantmorphology, and also investigating shifts in feature importancewhen comparingunimodal interpretability versus multimodalinterpretability.
基于深度学习的多模态整合
为了解决开发可用于癌症预后的联合图像组学生物标志物的挑战,我们提出了一种基于深度学习的多模态融合(MMF)算法,该算法利用H&E全切片图像和分子特征(突变状态、拷贝数变异、RNA测序[RNA-seq]表达)来衡量和解释癌症死亡的相对风险(图1A)。我们的多模态网络不仅能够在弱监督学习任务中整合这两种模态,如生存结果预测,还能够解释组织病理学特征、分子特征及其相互作用与低风险和高风险患者的相关性(图1B–1E)。在患者队列内进行风险评估后,我们的网络使用基于注意力和归因的可解释性作为一种无针对性的方法来估计所有患者的预后标志(图1B–1F)。我们的研究使用了来自TCGA的14种癌症类型中的5,720个患者样本的6,592个千兆像素WSI(表S1)。对于每种癌症类型,我们使用我们的弱监督范式在5倍交叉验证中训练了我们的多模态模型,并进行了消融分析,比较了单模态和多模态预后模型的性能。在训练和模型评估之后,我们对网络的可解释性进行了广泛的分析,研究了每种癌症类型的局部和全局水平的图像组解释,量化了与相关形态学对应的组织微结构,并调查了在单模态可解释性与多模态可解释性之间进行比较时特征重要性的变化。
Figure
图
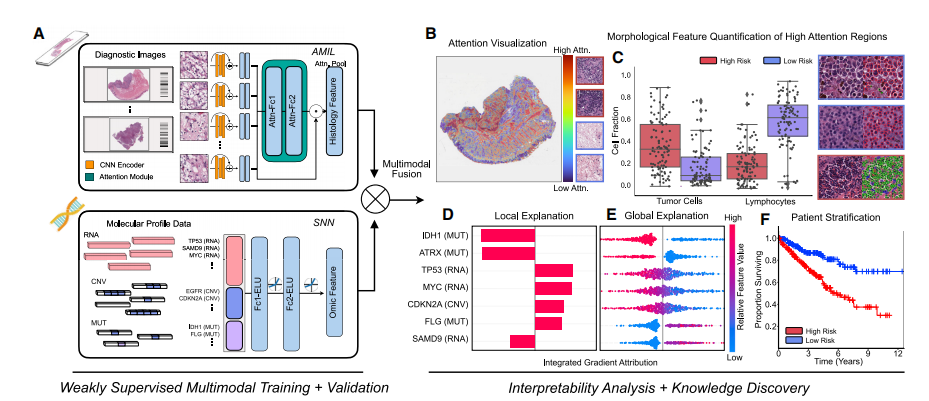
Figure 1. Pathology-Omic Research Platform for Integrative Survival Estimation (PORPOISE) workflow(A) Patient data in the form of digitized high-resolution formalin-fixed paraffin-embeded (FFPE) H&E histology glass slides (known as WSIs) with correspondingmolecular data are used as input in our algorithm. Our multimodal algorithm consists of three neural network modules together: (1) an attention-based multipleinstance learning (AMIL) network for processing WSIs, (2) a self-normalizing network (SNN) for processing molecular data features, and (3) a multimodal fusionlayer that computes the Kronecker Product to model pairwise feature interactions between histology and molecular features.(B) For WSIs, per-patient local explanations are visualized as high-resolution attention heatmaps using attention-based interpretability, in which high-attentionregions (red) in the heatmap correspond to morphological features that contribute to the model’s predicted risk score.© Global morphological patterns are extracted via cell quantification of high-attention regions in low- and high-risk patient cohorts.(D) For molecular features, per-patient local explanations are visualized using attribution-based interpretability in integrated gradients.(E) Global interpretability for molecular features is performed via analyzing the directionality, feature value, and magnitude of gene attributions across all patients.(F) Kaplan-Meier analysis is performed to visualize patient stratification of low- and high-risk patients for individual cancer types.
图1. 用于整合生存估计的病理组学研究平台(PORPOISE)工作流程(A)患者数据以数字化的高分辨率福尔马林固定石蜡包埋(FFPE)H&E组织学玻璃切片(称为WSI)及其相应的分子数据的形式输入我们的算法。我们的多模态算法由三个神经网络模块组成:(1)基于注意力的多实例学习(AMIL)网络用于处理WSI,(2)用于处理分子数据特征的自归一化网络(SNN),(3)多模态融合层计算克罗内克积,以模拟组织学和分子特征之间的成对特征相互作用。
(B)对于WSI,通过基于注意力的可解释性,将每个患者的局部解释以高分辨率的注意力热图形式可视化,其中热图中的高注意力区域(红色)对应于对模型预测的风险分数做出贡献的形态学特征。
(C)通过对低风险和高风险患者队列中的高注意力区域的细胞计量来提取全局形态学模式。
(D)对于分子特征,使用归因可解释性以综合梯度的形式可视化每个患者的局部解释。
(E)通过分析所有患者的基因归因的方向性、特征值和幅度,执行分子特征的全局可解释性。
(F)执行Kaplan-Meier分析以可视化低风险和高风险患者的个体癌症类型分层。
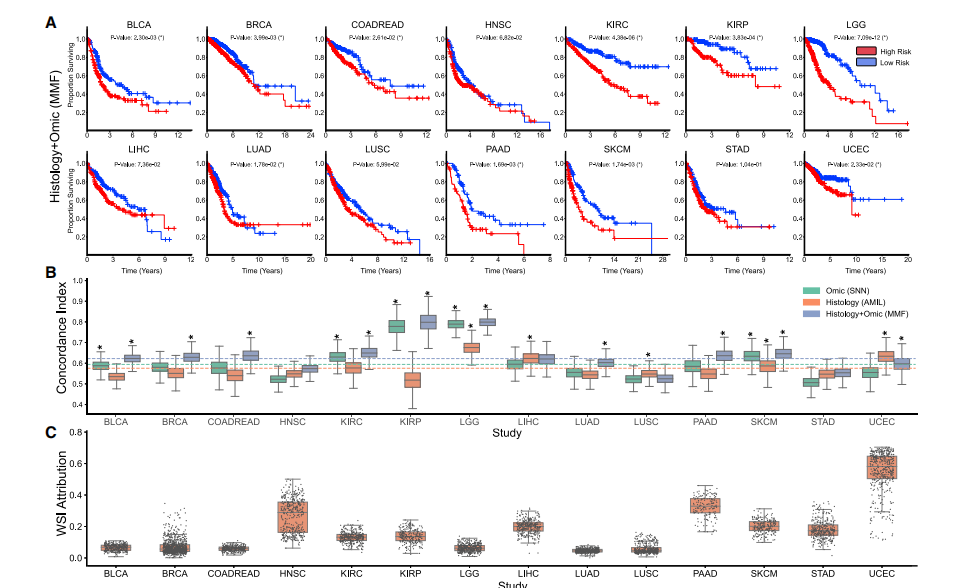
Figure 2. Model performances of PORPOISE and understanding impact of multimodal training(A) Kaplan-Meier analysis of patient stratification of low- and high-risk patients via MMF across all 14 cancer types. Low and high risks are defined by the median50% percentile of hazard predictions via MMF. Log rank test was used to test for statistical significance in survival distributions between low- and high-riskpatients (*p < 0.05).(B) c-Index performance of SNN, AMIL, and MMF in each cancer type in a 5-fold cross-validation (n = 5,720). Horizontal line for each model shows averagec-Index performance across all cancer types. Boxplots correspond to c-Indices of 1,000 bootstrap replicates on the aggregated risk predictions.© Distribution of WSI attribution across 14 cancer types. Each dot represents the proportion of feature attribution given to the WSI modality input compared withmolecular feature input. Attributions were computed on the aggregated risk predictions in each disease model. Boxes indicate quartile values and whiskersextend to data points within 1.53 the interquartile range.See also Figures S1–S3, S11, S12 and Tables S1, S2, and S3.
图2. PORPOISE模型性能及多模态训练影响的理解
(A)通过MMF对所有14种癌症类型的低风险和高风险患者进行分层的Kaplan-Meier分析。低风险和高风险是通过MMF对风险预测的中位数50%百分位数来定义的。使用log rank检验测试低风险和高风险患者之间的生存分布的统计学显著性(*p < 0.05)。
(B)在5倍交叉验证(n = 5,720)中每种癌症类型中SNN、AMIL和MMF的c-Index性能。每个模型的水平线显示了所有癌症类型的平均c-Index性能。箱线图对应于在聚合风险预测上的1,000个bootstrap复制品的c-Index。
(C)14种癌症类型的WSI归因分布。每个点表示给定于WSI模态输入与分子特征输入相比的特征归因的比例。在每个疾病模型中计算了聚合风险预测的归因。方框表示四分位数值,而横线则延伸至相对于四分位距1.53倍的数据点。
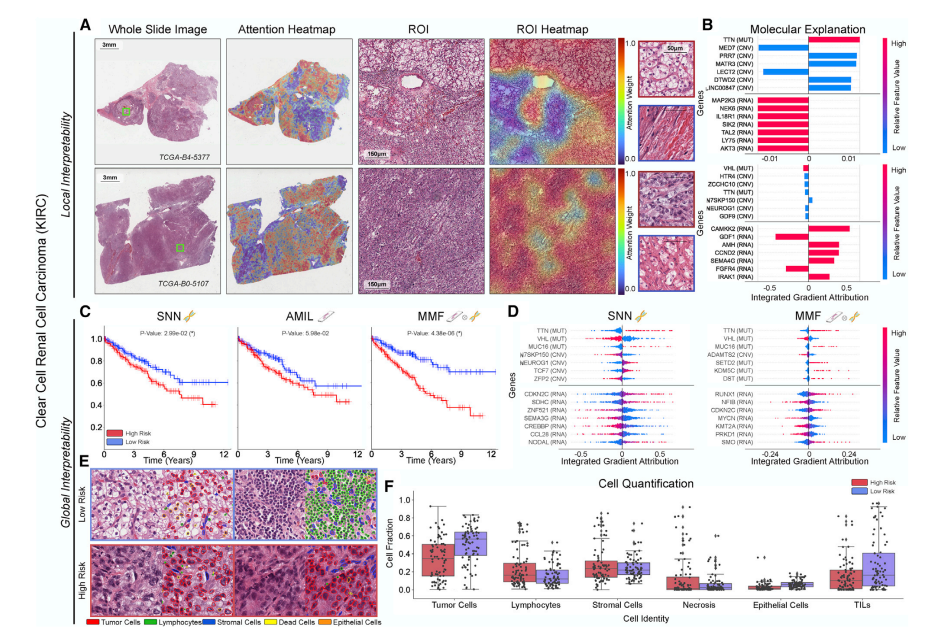
Figure 3. Quantitative performance, local model explanation, and global interpretability analyses of PORPOISE on clear cell renal cell carcinoma (KIRC)(A) For KIRC (n = 345), high attention for low-risk cases (top, n = 80) tends to focus on classic clear cell morphology, while in high-risk cases (bottom, n = 80), highattention often corresponds to areas with decreased cytoplasm or increased nuclear to cytoplasmic ratio.(B) Local gene attributions for the corresponding low- (top) and high-risk (bottom) cases.© Kaplan-Meier curves for omics only (left, ‘‘SNN’’), histology only (center, ‘‘AMIL’’), and multimodal fusion (right, ‘‘MMF’’), showing improved separation usingMMF. Logrank test was used to test for statistical significance in survival distributions between low- and high-risk patients (with * marked if p-Value < 0.05).(D) Global gene attributions across patient cohorts according to unimodal interpretability (left, ‘‘SNN’’) and multimodal interpretability (right, ‘‘MMF’’). SNN andMMF were both able to identify immune-related and prognostic markers such as CDKN2C and VHL in KIRC. MMF additionally attributes to other immune-related/prognostic genes such as RUNX1 and NFIB in KIRC.(E) Exemplar high-attention patches from low- (top) and high-risk (bottom) cases with corresponding cell labels.(F) Quantification of cell types in high-attention patches for each disease overall, showing increased tumor and TIL presence. Boxes indicate quartile values andwhiskers extend to data points within 1.53 the interquartile range.See also Figures S2–S11 and Table S4.
图3. PORPOISE在清晰细胞肾细胞癌(KIRC)上的定量性能、局部模型解释和全局可解释性分析
(A)对于KIRC(n = 345),低风险病例(顶部,n = 80)的高注意力往往集中在经典的清晰细胞形态学上,而在高风险病例(底部,n = 80)中,高注意力通常对应于细胞质减少或核质比增加的区域。
(B)对应于低风险(顶部)和高风险(底部)病例的局部基因归因。
(C)仅组学(左侧,“SNN”)、仅组织学(中间,“AMIL”)和多模态融合(右侧,“MMF”)的Kaplan-Meier曲线,显示使用MMF可以改善分离效果。使用logrank检验测试低风险和高风险患者之间生存分布的统计学显著性(如果p-Value < 0.05,则标记*)。
(D)根据单模态可解释性(左侧,“SNN”)和多模态可解释性(右侧,“MMF”)在患者队列中的全局基因归因。SNN和MMF都能够在KIRC中识别与免疫相关和预后相关的标志物,如CDKN2C和VHL。MMF还额外归因于其他免疫相关/预后相关基因,如RUNX1和NFIB。
(E)低风险(顶部)和高风险(底部)病例的示例高注意力区域,带有相应的细胞标签。
(F)每种疾病中高注意力区域的细胞类型定量,显示肿瘤和TIL存在增加。方框表示四分位数值,而横线则延伸至相对于四分位距1.53倍的数据点。
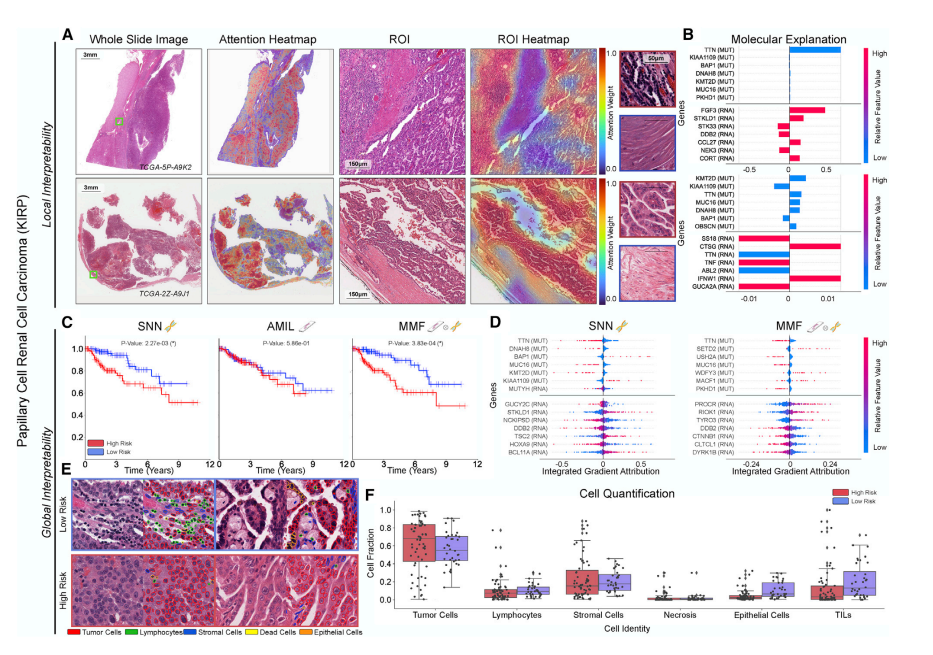
Figure 4. Quantitative performance, local model explanation, and global interpretability analyses of PORPOISE in papillary renal cell carcinoma (KIRP)(A) For KIRP (n = 253), low-risk cases (top, n = 36) often have high attention paid to complex and curving papillary architecture, while for high-risk cases (bottom, n= 63), high attention is paid to denser areas of tumor cells.(B) Local gene attributions for the corresponding low- (top) and high-risk (bottom) cases.© Kaplan-Meier curves for omics only (left, ‘‘SNN’’), histology only (center, ‘‘AMIL’’), and multimodal fusion (right, ‘‘MMF’’), showing improved separation usingMMF. Logrank test was used to test for statistical significance in survival distributions between low- and high-risk patients (with * marked if p-value < 0.05).(D) Global gene attributions across patient cohorts according to unimodal interpretability (left, ‘‘SNN’’) and multimodal interpretability (right, ‘‘MMF’’). SNN andMMF were both able to identify prognostic markers such as BAP1 in KIRP. MMF additionally attributes to other immune-related/prognostic genes such asPROCR and RIOK1 in KIRP.(E) Exemplar high-attention patches from low- (top) and high-risk (bottom) cases with corresponding cell labels.(F) Quantification of cell types in high-attention patches for each disease overall, showing increased epithelial cell and TIL presence. Boxes indicate quartilevalues and whiskers extend to data points within 1.53 the interquartile range.See also Figures S2–S11 and Table S4.
图4. PORPOISE在乳头状肾细胞癌(KIRP)中的定量性能、局部模型解释和全局可解释性分析
(A)对于KIRP(n = 253),低风险病例(顶部,n = 36)通常会将高注意力集中在复杂且弯曲的乳头结构上,而对于高风险病例(底部,n = 63),高注意力集中在肿瘤细胞密度较高的区域。
(B)对应于低风险(顶部)和高风险(底部)病例的局部基因归因。
(C)仅组学(左侧,“SNN”)、仅组织学(中间,“AMIL”)和多模态融合(右侧,“MMF”)的Kaplan-Meier曲线,显示使用MMF可以改善分离效果。使用logrank检验测试低风险和高风险患者之间生存分布的统计学显著性(如果p-value < 0.05,则标记*)。
(D)根据单模态可解释性(左侧,“SNN”)和多模态可解释性(右侧,“MMF”)在患者队列中的全局基因归因。SNN和MMF都能够识别KIRP中的预后标志物,如BAP1。MMF还额外归因于其他免疫相关/预后相关基因,如PROCR和RIOK1。
(E)低风险(顶部)和高风险(底部)病例的示例高注意力区域,带有相应的细胞标签。
(F)每种疾病中高注意力区域的细胞类型定量,显示上皮细胞和TIL存在增加。方框表示四分位数值,而横线则延伸至相对于四分位距1.53倍的数据点。
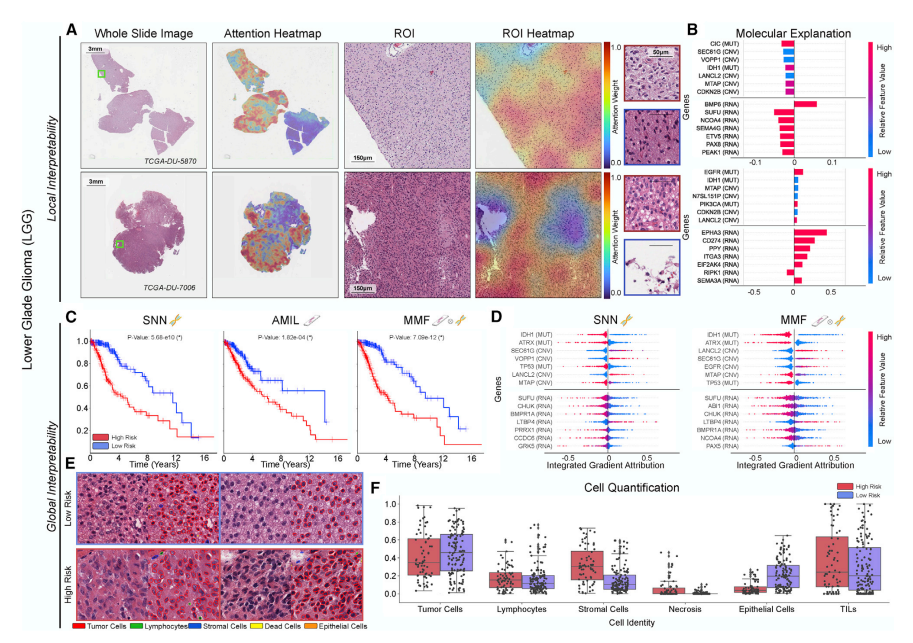
Figure 5. Quantitative performance, local model explanation, and global interpretability analyses of PORPOISE on lower-grade gliomas (LGGs)(A) For LGGs (n = 404), high attention for low-risk cases (top, n = 133) tends to focus on dense regions of tumor cells, while in high-risk cases (bottom, n = 68), highattention focuses on both dense regions of tumor cells and areas of vascular proliferation.(B) Local gene attributions for the corresponding low- (top) and high-risk (bottom) cases.© Kaplan-Meier curves for omics only (left, ‘‘SNN’’), histology only (center, ‘‘AMIL’’), and multimodal fusion (right, ‘‘MMF’’), demonstrating improvement in patientstratification in MMF. Logrank test was used to test for statistical significance in survival distributions between low- and high-risk patients (with * marked if p-value< 0.05).(D) Global gene attributions across patient cohorts according to unimodal interpretability (left, ‘‘SNN’’) and multimodal interpretability (right, ‘‘MMF’’). SNN andMMF were both able to identify immune-related and prognostic markers such as IDH1, ATRX, EGFR, and CDKN2B in LGGs.(E) High-attention patches from low- (top) and high-risk (bottom) cases with corresponding cell labels, showing oligodendroglioma and astrocytoma subtypesrespectively.(F) Quantification of cell types in high-attention patches for each disease overall, with statistical significance for increased necrosis in high-risk patients. Boxesindicate quartile values and whiskers extend to data points within 1.53 the interquartile range.
图5. PORPOISE在低级别胶质瘤(LGGs)上的定量性能、局部模型解释和全局可解释性分析
(A)对于LGGs(n = 404),低风险病例(顶部,n = 133)的高注意力往往集中在肿瘤细胞密集区域,而高风险病例(底部,n = 68)的高注意力则同时集中在肿瘤细胞密集区域和血管增生区域。
(B)对应于低风险(顶部)和高风险(底部)病例的局部基因归因。
(C)仅组学(左侧,“SNN”)、仅组织学(中间,“AMIL”)和多模态融合(右侧,“MMF”)的Kaplan-Meier曲线,显示使用MMF可以改善患者分层效果。使用logrank检验测试低风险和高风险患者之间生存分布的统计学显著性(如果p-value < 0.05,则标记*)。
(D)根据单模态可解释性(左侧,“SNN”)和多模态可解释性(右侧,“MMF”)在患者队列中的全局基因归因。SNN和MMF都能够识别LGGs中的免疫相关和预后相关标志物,如IDH1、ATRX、EGFR和CDKN2B。
(E)低风险(顶部)和高风险(底部)病例的示例高注意力区域,带有相应的细胞标签,显示分别为寡脂质瘤和星形胶质瘤亚型。
(F)每种疾病中高注意力区域的细胞类型定量,高风险患者中增加的坏死具有统计学显著性。方框表示四分位数值,而横线则延伸至相对于四分位距1.53倍的数据点。
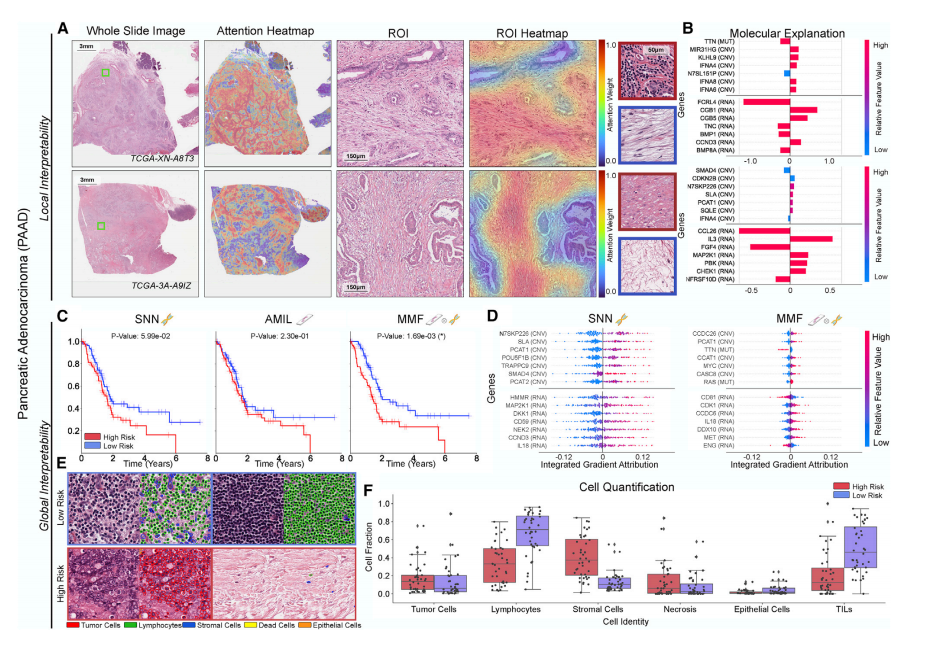
Figure 6. Quantitative performance, local model explanation, and global interpretability analyses of PORPOISE on pancreatic adenocarcinoma (PAAD)(A) For PAAD (n = 160), high attention for low-risk cases (top, n = 40) tends to focus on stroma-contained dispersed glands and aggregates of lymphocytes, whilein high-risk cases (bottom, n = 40), high attention focuses on tumor-associated and myxoid stroma.(B) Local gene attributions for the corresponding low- (top) and high-risk (bottom) cases from (A) and (G).© Kaplan-Meier curves for omics only (left, ‘‘SNN’’), histology only (center, ‘‘AMIL’’), and multimodal fusion (right, ‘‘MMF’’), demonstrating SNN and AMILshowing poor separation of patients with low survival, with better stratification following multimodal integration. Logrank test was used to test for statisticalsignificance in survival distributions between low- and high-risk patients (with * marked if p-value < 0.05).(D) Global gene attributions across patient cohorts according to unimodal interpretability (left, ‘‘SNN’’) and multimodal interpretability (right, ‘‘MMF’’). SNN andMMF were both able to identify immune-related and prognostic markers such as IL8, EGFR, and MET in PAAD. MMF additionally shifts attribution to otherimmune-related/prognostic genes such as CD81, CDK1, and IL9.(E) High-attention patches from low- (top) and high-risk (bottom) cases with corresponding cell labels.(F) Quantification of cell types in high-attention patches for each disease overall, showing increased lymphocyte and TIL presence in low-risk patients, as well asincreased necrosis presence in PAAD. Boxes indicate quartile values and whiskers extend to data points within 1.53 the interquartile range.
图6. PORPOISE在胰腺腺癌(PAAD)上的定量性能、局部模型解释和全局可解释性分析
(A)对于PAAD(n = 160),低风险病例(顶部,n = 40)的高注意力往往集中在含有分散的腺体和淋巴细胞聚集的基质内,而高风险病例(底部,n = 40)的高注意力则集中在与肿瘤相关的粘液样基质上。
(B)从(A)和(G)对应的低风险(顶部)和高风险(底部)病例的局部基因归因。
(C)仅组学(左侧,“SNN”)、仅组织学(中间,“AMIL”)和多模态融合(右侧,“MMF”)的Kaplan-Meier曲线,显示SNN和AMIL在显示低生存率患者的分离效果差,而多模态融合后的分层效果更好。使用logrank检验测试低风险和高风险患者之间生存分布的统计学显著性(如果p-value < 0.05,则标记*)。
(D)根据单模态可解释性(左侧,“SNN”)和多模态可解释性(右侧,“MMF”)在患者队列中的全局基因归因。SNN和MMF都能够识别PAAD中的免疫相关和预后相关标志物,如IL8、EGFR和MET。MMF还额外将归因转移到其他免疫相关/预后相关基因,如CD81、CDK1和IL9。
(E)低风险(顶部)和高风险(底部)病例的示例高注意力区域,带有相应的细胞标签。
(F)每种疾病中高注意力区域的细胞类型定量,显示低风险患者中淋巴细胞和TIL存在增加,以及PAAD中坏死存在增加。方框表示四分位数值,而横线则延伸至相对于四分位距1.53倍的数据点。
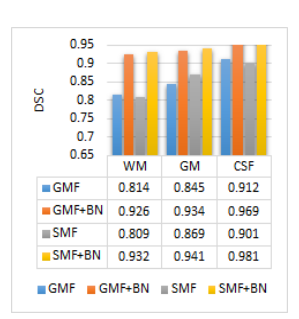
Figure 7. TIL quantification in patient risk groups
TIL quantification in high-attention regions of predicted low- (BLCA n = 90, BRCA n = 220, COADREAD n = 74, HNSC n = 96, KIRC n = 80, KIRP n = 36, LGG n =133, LIHC n = 85, LUAD n = 105, LUSC n = 97, PAAD n = 40, SKCM n = 29, STAD n = 53, UCEC = 104) and high-risk patient cases (BLCA n = 93, BRCA n = 223,COADREAD n = 80, HNSC n = 103, KIRC n = 80, KIRP n = 63, LGG n = 68, LIHC n = 84, LUAD n = 89, LUSC n = 103, PAAD n = 40, SKCM n = 55, STAD n = 78,UCEC = 125) across 14 cancer types. For each patient, the top 1% of scored high-attention regions (512 3 512 403 image patches) were segmented andanalyzed for tumor and immune cell presence. Image patches with high tumor-immune co-localization were indicated as positive for TIL presence (and negativeotherwise). Across all patients, the fraction of high-attention patches containing TIL presence was computed and visualized in the boxplots. A two-sample t test
was computed for each cancer type to test the if the means of the TIL fraction distributions of low- and high-risk patients had a statistically significant difference(*p < 0.05). Boxes indicate quartile values and whiskers extend to data points within 1.53 the interquartile range.
图7. 患者风险组中的TIL定量分析
在14种癌症类型中预测低风险(BLCA n = 90,BRCA n = 220,COADREAD n = 74,HNSC n = 96,KIRC n = 80,KIRP n = 36,LGG n = 133,LIHC n = 85,LUAD n = 105,LUSC n = 97,PAAD n = 40,SKCM n = 29,STAD n = 53,UCEC n = 104)和高风险(BLCA n = 93,BRCA n = 223,COADREAD n = 80,HNSC n = 103,KIRC n = 80,KIRP n = 63,LGG n = 68,LIHC n = 84,LUAD n = 89,LUSC n = 103,PAAD n = 40,SKCM n = 55,STAD n = 78,UCEC n = 125)患者病例中TIL的定量分析。对于每个患者,对评分的高注意力区域(512 * 512像素图像块)的前1%进行分割并进行肿瘤和免疫细胞存在性分析。具有高肿瘤-免疫共定位的图像块被标记为TIL存在(否则为阴性)。在所有患者中,计算并可视化了包含TIL存在的高注意力区域的比例。对于每种癌症类型,进行了双样本t检验,以测试低风险和高风险患者的TIL比例分布的均值是否存在统计学显著差异(*p < 0.05)。方框表示四分位数值,而横线则延伸至相对于四分位距1.53倍的数据点