Title
题目
Robust breast cancer detection in mammography and digital breast tomosynthesis using an annotation-efficient deep learning approach
鲁棒的乳腺癌检测在乳房X光摄影术 和数字化乳腺层析扫描中使用一个 标注高效的深度学习方法
01
文献速递介绍
乳腺癌依然是全球面临的一大挑战,2018年导致超过60万人死亡。为了实现更早的癌症检测,全球各地的健康组织推荐进行筛查性乳腺X光摄影,据估计这能将乳腺癌死亡率降低20-40%。尽管筛查性乳腺X光摄影的价值明确无误,但是较高的假阳性和假阴性率,以及专家阅片可用性的不一致性,都留下了提高质量和获取机会的空间。为了解决这些限制,最近对于应用深度学习于乳腺X光摄影的兴趣激增这些努力突显了两个主要困难:获取大量的标注训练数据和确保跨人群、获取设备及模态的泛化。这里我们提出了一种注释高效的深度学习方法,它在乳腺X光摄影分类中达到了最先进的性能,成功扩展到数字乳腺体层摄影(DBT;“3D乳腺X光摄影”),在患有癌症的病人的临床阴性先前乳腺X光摄影中检测到癌症,在筛查率低的人群中表现出良好的泛化能力,以及在平均灵敏度提高14%的基础上,胜过了五名全职乳腺影像专家中的五名。通过从DBT数据创建新的“最大怀疑投影”(MSP)图像,我们的渐进式训练的多实例学习方法有效地仅使用乳腺级别的标签对DBT检查进行训练,同时保持了基于定位的可解释性。总而言之,我们的结果展示了向提高全球筛查性乳腺X光摄影的准确性和获取性的软件的前景。
Fig
图
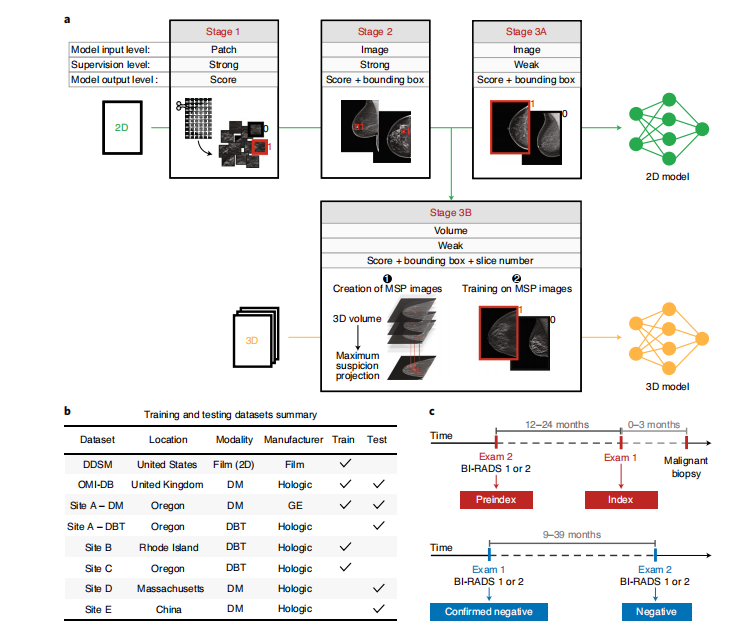
Fig. 1 | Model training approach and data summary. a, To effectively leverage both strongly and weakly labeled data while mitigating overfitting, we progressively trained our deep learning models in a series of stages. Stage 1 consists of patch-level classification using cropped image patches from 2D mammograms15. In Stage 2, the model trained in Stage 1 is used to initialize the feature backbone of a detection-based model. The detection model, which outputs bounding boxes with corresponding classification scores, is then trained end-to-end in a strongly supervised manner on full images. Stage 3 consists of weakly supervised training, for both 2D and 3D mammography. For 2D mammography (Stage 3A), the detection network is trained for binary classification in an end-to-end, multiple-instance learning fashion where an image-level score is computed as a maximum over bounding box scores. For 3D mammography (Stage 3B), the model from Stage 2 is used to condense each DBT stack into an optimized 2D projection by evaluating the DBT slices and extracting the most suspicious region of interest at each x–y spatial location. The model is then trained on these MSP images using the approach in Stage 3A. b, Summary of training and testing datasets. c, Illustration of exam definitions used here.
图 1 | 模型训练方法及数据概述。a,为了有效利用强标签和弱标签数据同时减轻过拟合,我们 通过一系列阶段逐步训练我们的深度学习模型。第一阶段由使用从2D 乳房X光摄影术中裁剪的图像块进行的片段级分类组成。在第二阶段,第一阶段中训练的模型被用来初始化检测模型的特征主干。然后,这个检测模型,它输出带有相应分类分数的边界框,然后在完整图像上以强监督方式端到端训练。第三阶段 包括弱监督训练,适用于2D和3D乳房X光摄影术。对于2D乳房X光摄影术(第3A阶段),检测网络以端到端、多实例学习方式进行二分类训练,其中图像级分数计算为边界框分数的最大值。对于 3D乳房X光摄影术(第3B阶段),第二阶段的模型被用来通过评估DBT切片并在每个x–y空间位置提取最可疑的感兴趣区域,将每个DBT堆栈压缩成一个优化的2D投影。然后,模型根据 第3A阶段中的方法在这些MSP图像上进行训练。b,训练和测试数据集的概述。c,此处使用的考试定义说明。
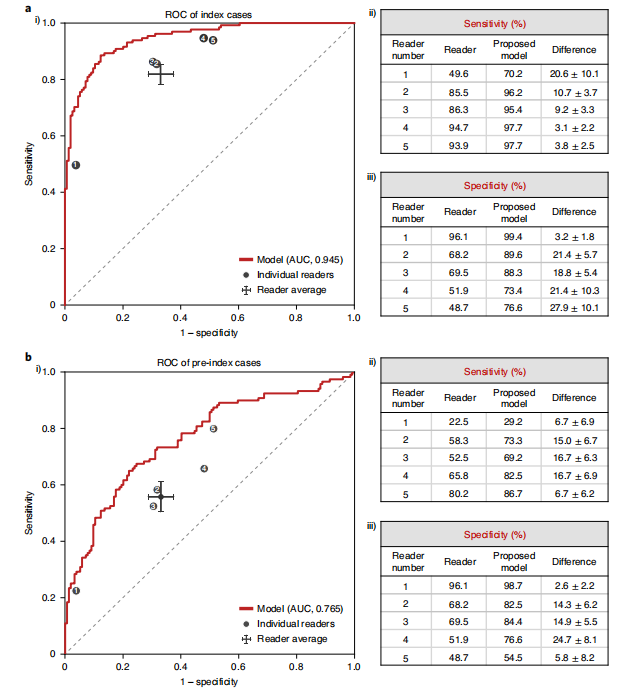
Fig. 2 | Reader study results. a, Index cancer exams and confirmed negatives. i, The proposed deep learning model outperformed all 5 radiologists on the set of 131 index cancer exams and 154 confirmed negatives. Each data point represents a single reader, and the ROC curve represents the performance of the deep learning model. The cross corresponds to the mean radiologist performance with the lengths of the cross indicating 95% confidence intervals.
ii, Sensitivity of each reader and the corresponding sensitivity of the proposed model at a specificity chosen to match each reader. iii, Specificity of each reader and the corresponding specificity of the proposed model at a sensitivity chosen to match each reader. b, Pre-index cancer exams and confirmed negatives. i, The proposed deep learning model also outperformed all five radiologists on the early-detection task. The dataset consisted of 120 pre-index cancer exams—which are defined as mammograms interpreted as negative 12–24 months prior to the index exam in which cancer was found—and 154 confirmed negatives. The cross corresponds to the mean radiologist performance, with the lengths of the cross indicating 95% confidence intervals.ii, Sensitivity of each reader and the corresponding sensitivity of the proposed model at a specificity chosen to match each reader. iii, Specificity of each reader and the corresponding specificity of the proposed model at a sensitivity chosen to match each reader. For the sensitivity and specificity tables, the s.d. of the model minus reader difference was calculated via bootstrapping.
图 2 | 阅读者研究结果。a,索引癌症检查和确认的阴性。i,提出的深度学习模型在 131个索引癌症检查和154个确认的阴性集合上超越了所有5位放射科医生。每个数据点代表一个单独的阅读者,而ROC曲线代表了 深度学习模型的表现。十字架对应于平均放射科医生的表现,十字架的长度表示95%置信区间。
ii,每位阅读者的敏感性以及在选择与每位阅读者匹配的特异性下提出模型的相应敏感性。iii,每位 阅读者的特异性以及在选择与每位阅读者匹配的敏感性下提出模型的相应特异性。b,索引前癌症检查和确认的阴性。i,提出的深度学习模型也在早期检测任务上超过了所有五位放射科医生。数据集由120个索引前 癌症检查组成——这些检查被定义为在发现癌症的索引检查前12-24个月解读为阴性的乳房X光摄影——和154个确认的阴性。十字架对应于平均放射科医生的表现,十字架的长度表示95%置信区间。ii,每位阅读者的敏感性以及在选择与每位阅读者匹配的特异性下提出模型的相应敏感性。iii,每位阅读者的特异性以及在选择与每位阅读者匹配的敏感性下提出模型的相应特异性。对于敏感性和特异性表格,通过自举法计算了模型减去阅读者差异的标准差
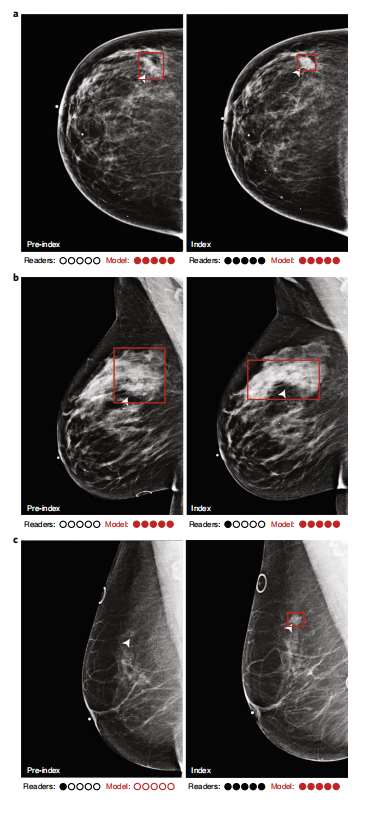
Fig. 3 | Examples of index and pre-index cancer exam pairs. Images from three patients with biopsy-proven malignancies are displayed. For each patient, an image from the index exam from which the cancer was discovered is shown on the right, and an image from the prior screening exam acquired 12–24 months earlier and interpreted as negative is shown on the left. From top to bottom, the number of days between the index and pre-index exams is 378, 629, and 414. The dots below each image indicate reader and model performance. Specifically, the number of infilled black dots represent how many of the five readers correctly classified the corresponding case, and the number of infilled red dots represent how many times the model would correctly classify the case if the model score threshold was individually set to match the specificity of each reader. The model is thus evaluated at five binary decision thresholds for comparison purposes, and we note that a different binary score threshold may be used in practice. Red boxes on the images indicate the model’s bounding box output. White arrows indicate the location of the malignant lesion. a, A cancer that was correctly classified by all readers and the deep learning model at all thresholds in the index case, but detected by only the model in the pre-index case. b, A cancer that was detected by the model in both the pre-index and index cases, but detected by only one reader in the index case and zero readers in the pre-index case. c, A cancer that was detected by the readers and the model in the index case, but detected by only one reader in the pre-index case. The absence of a red bounding box indicates that the model did not detect the cancer.
图 3 | 索引及索引前癌症检查对的例子。展示了三位经活组织检查证实为恶性的患者的图像。对于每位患者,右侧展示了发现癌症的索引检查的图像,而左侧展示了12-24个月前进行的、解读为阴性的前一次筛查检查的图像。从上到下,索引检查与索引前检查之间的天数分别为378天、629天和414天。每张图像下方的点表示阅读者和模型的表现。具体来说,填充的黑点的数量表示五位阅读者中有多少人正确分类了相应的病例,填充的红点的数量表示如果模型分数阈值被单独设置以匹配每位阅读者的特异性,模型将正确分类该病例的次数。因此,模型在五个二进制决策阈值下进行评估,以便进行比较,我们注意到在实践中可能会使用不同的二进制分数阈值。图像上的红色框表示模型的边界框输出。白色箭头指示恶性病变的位置。a,在索引案例中,所有阅读者和深度学习模型在所有阈值下均正确分类的癌症,但在索引前案例中仅由模型检测到。b,在索引前和索引案例中均由模型检测到的癌症,但在索引案例中仅有一位阅读者检测到,在索引前案例中无阅读者检测到。c,在索引案例中被阅读者和模型检测到的癌症,但在索引前案例中仅有一位阅读者检测到。没有红色边界框表明模型未检测到癌症。
Table
表
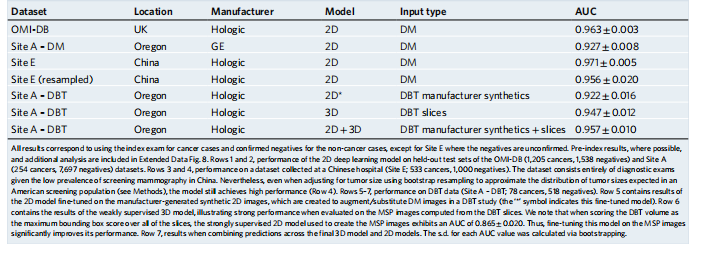
Table 1 | Summary of additional DM and DBT evaluation
表 1 | 附加DM和DBT评估摘要