Title
题目
Biology-guided deep learning predicts prog
nosis and cancer immunotherapy response
生物学引导的深度学习预测预后和癌症免疫治疗反应
01
文献速递介绍
Substantial progress has been made in using deep learning for cancer detection and diagnosis in medical images. Yet, there is limited success on prediction of treatment response and outcomes, which has important implications for personalized treatment strategies. A significant hurdle for clinical translation of current data-driven deep learning models is lack of interpretability, often attributable to a disconnect from the underlying pathobiology. Here, we present a biology-guided deep learning approach that enables simultaneous prediction of the tumor immune and stromal microenvironment status as well as treatment outcomes from medical images. We validate the model for predicting prognosis of gastric cancer and the benefit from adjuvant chemotherapy in a multi-center international study. Further, the model predicts response to immune checkpoint inhi bitors and complements clinically approved biomarkers. Importantly, our model identifies a subset of mismatch repair-deficient tumors that are non responsive to immunotherapy and may inform the selection of patients for combination treatments.
在医学影像中的检测和诊断方面,尽管取得了一定的成功,但在治疗反应和结果的预测上仍然成功有限,这对于个性化治疗策略具有重要意义。当前数据驱动的深度学习模型在临床转化上的一个重大障碍是缺乏可解释性,这通常归因于与潜在病理生物学的脱节。在这里,我们提出了一种生物学引导的深度学习方法,该方法能够同时预测肿瘤免疫和基质微环境状态以及从医学影像中的治疗结果。我们在一个多中心国际研究中验证了该模型用于预测胃癌的预后和辅助化疗的益处。此外,该模型预测了对免疫检查点抑制剂的反应,并补充了临床批准的生物标志物。重要的是,我们的模型识别出一组错配修复缺陷的肿瘤,这些肿瘤对免疫治疗没有反应,并可能为选择患者进行联合治疗提供信息。
Results
结果
Study design and patient characteristics
The overall study design is shown in Fig. 1. We trained and indepen dently validated a deep learning model that used diagnostic CT images to classify TME and predict prognosis of patients with gastric cancer. The rationale for combining TME and outcome prediction in a single model is that they are closely related and inter-connected tasks given the established mechanistic link between the two. We hypothesize that this approach could lead to improved generalizability with the added benefit of enhanced interpretability. We tested the model for its ability to predict benefit from adjuvant chemotherapy in non-metastatic disease as well as to predict immunotherapy response in advanced gastric cancer.
We recruited patients in four academic medical centers from China and United States (Supplementary Fig 1). A total of 2799 patients met inclusion criteria and were divided into in 7 cohorts. Among these, 2496 patients in 6 cohorts were treated with surgery with or without adjuvant chemotherapy, and the clinicopathological characteristics are listed in Supplementary Data 1. The majority of these patients (n = 1806, 72.36%) had stage II or III disease, and 928 (51.38%) patients received adjuvant chemotherapy. In the 7th cohort, we included 303 patients who received anti-PD-1 immunotherapy (Supplementary Data 2). All patients had stage IV gastric cancer, and most (94%) tumors were mismatch repair deficient (dMMR) or MSI-H.
研究设计与患者特征
整体研究设计如图1所示。我们训练并独立验证了一个深度学习模型,该模型使用诊断性CT图像来分类肿瘤微环境(TME)并预测胃癌患者的预后。将TME和结果预测结合在一个模型中的理由是,鉴于两者之间已建立的机制联系,这两个任务紧密相关且相互连接。我们假设这种方法可以提高泛化能力,并增加解释能力。我们测试了该模型预测非转移性疾病中辅助化疗的益处以及预测晚期胃癌免疫疗法反应的能力。
我们在中国和美国的四所学术医学中心招募了患者(补充图1)。共有2799名患者满足纳入标准,被分为7个队列。其中,2496名患者在6个队列中接受了手术治疗,有无辅助化疗,临床病理特征列在补充数据1中。这些患者中的大多数(n = 1806, 72.36%)患有II期或III期疾病,928名(51.38%)患者接受了辅助化疗。在第7个队列中,我们包括了303名接受抗PD-1免疫疗法的患者(补充数据2)。所有患者都患有IV期胃癌,且大多数(94%)肿瘤为错配修复缺陷(dMMR)或高微卫星不稳定性(MSI-H)。
Methods
方法
This study was approved by the Institutional Review Board at four academic medical centers, including Nanfang Hospital of Southern Medical University, Sun Yat-sen University Cancer Center, Guangdong Provincial Hospital of Chinese Medicine, and Stanford University School of Medicine. Informed consent was waived for this retro spective study. We reviewed data for 5133 patients with gastric ade nocarcinoma who underwent surgical resection or immunotherapy. The inclusion criteria for the surgical cohorts were: histologically confirmed diagnosis of GC; at least 15 lymph nodes harvested; pre operative contrast-enhanced abdominal CT available; and complete clinicopathological and follow-up data available. We excluded patients whose primary tumor could not be identified on CT, who received neoadjuvant chemotherapy or had other synchronous malignant
neoplasms. For the immunotherapy cohort, the inclusion criteria were: pretreatment contrast-enhanced abdominal CT available, and clin icopathological and follow-up data available.
A total of 2799 patients in seven independent cohorts were enrolled in this study (Supplementary Fig. 1). In the Nanfang Hos pital cohort, we divided patients into training and validation cohorts by the time of surgery. The training cohort and two internal validation cohorts included 348, 202, and 636 patients who were consecutively treated at Nanfang Hospital of Southern Medical University (Guangzhou, China) from January 1, 2005 to December 31, 2008, from January 1, 2009 to June 30, 2012, and from July 1,2012 to December 31, 2016 respectively. Of note, the training cohort contains patients with complete data available that are necessary for model development. The two external validation cohorts included 125 and 1062 patients consecutively treated at Sun Yat-sen University Cancer Center (SYSUCC) between June 1, 2007 and June 30, 2013. Another interna tional external validation cohort included 123 patients treated at Stanford University Medical Center between August 1, 2000 and May 31, 2013. Additionally, we enrolled advanced GC patients treated with anti-PD1 immunotherapy at two institutions between January 1, 2019 and July 31, 2021. Clinicopathologic data including age, gender, tumor and lymph node status, tumor differentiation, Lauren histology type, carcinoem bryonic antigen (CEA), and cancer antigen 19-9 (CA19-9) were col lected. D2 lymph node dissection was performed in most patients (>90%) in accordance with the Japanese guidelines41. All patients were restaged according to the eighth edition of the American Joint Com mittee on Cancer (AJCC) staging criteria. In the training cohort, inter nal validation cohorts 1 and 2 from Nanfang Hospital, there were 173 (49.70%), 92 (45.5%), and 373 (58.6%) patients who received 5- fluorouracil–based chemotherapy, respectively. In the external vali dation cohort from SYSUCC, 559 (47.9%) patients received 5-fluorouracil–based chemotherapy.
The immunotherapy cohort consists of 303 patients with advanced GC treated at Nanfang Hospital and Guangdong Provincial Hospital of Chinese Medicine. Anti-PD-1 drugs include: Nivolumab, Pembrolizumab, and Toripalimab. Clinical data, including patient demographics, treatment information, laboratory & pathologic examinations, and computed tomography (CT) scans were acquired.
Microsatellite instability (MSI) status was assessed by either IHC or DNA sequencing.
本研究已获得包括南方医科大学南方医院、中山大学肿瘤中心、广东省中医院及斯坦福大学医学院四所学术医学中心的机构审查委员会批准。对于这项回顾性研究,免除了知情同意。我们回顾了5133名接受手术切除或免疫疗法的胃腺癌患者的数据。
手术队列的纳入标准是:组织学确认的GC诊断;至少采集15个淋巴结;有术前增强CT可用;且有完整的临床病理和随访数据。我们排除了在CT上无法识别原发肿瘤、接受新辅助化疗或有其他同步恶性肿瘤的患者。对于免疫疗法队列,纳入标准是:有术前增强CT可用,且有临床病理和随访数据。
共有2799名患者被纳入本研究的七个独立队列(补充图1)。在南方医院队列中,我们根据手术时间将患者分为训练和验证队列。训练队列和两个内部验证队列分别包括在2005年1月1日至2008年12月31日、2009年1月1日至2012年6月30日、2012年7月1日至2016年12月31日在南方医科大学南方医院(中国广州)连续治疗的348名、202名和636名患者。值得注意的是,训练队列包含了对模型开发必需的完整数据的患者。
两个外部验证队列包括在2007年6月1日至2013年6月30日之间在中山大学肿瘤中心(SYSUCC)连续治疗的125名和1062名患者。另一个国际外部验证队列包括在2000年8月1日至2013年5月31日之间在斯坦福大学医学中心治疗的123名患者。此外,我们还纳入了2019年1月1日至2021年7月31日在两所机构治疗的晚期GC患者接受抗PD-1免疫疗法。
收集的临床病理数据包括年龄、性别、肿瘤和淋巴结状态、肿瘤分化度、Lauren组织学类型、癌胚抗原(CEA)和癌抗原19-9(CA19-9)。大多数患者(>90%)根据日本指南进行了D2淋巴结切除。所有患者根据美国癌症联合委员会(AJCC)第八版分期标准重新分期。在南方医院的训练队列和内部验证队列1和2中,分别有173(49.70%)、92(45.5%)和373(58.6%)名患者接受了基于5-氟尿嘧啶的化疗。在SYSUCC的外部验证队列中,有559(47.9%)名患者接受了基于5-氟尿嘧啶的化疗。
免疫疗法队列由303名在南方医院和广东省中医院治疗的晚期GC患者组成。抗PD-1药物包括:纳武单抗、帕博利珠单抗和托利珠单抗。收集的临床数据包括患者人口统计学信息、治疗信息、实验室和病理检查以及计算机断层扫描(CT)扫描。微卫星不稳定性(MSI)状态通过免疫组化(IHC)或DNA测序评估。
Fig
图
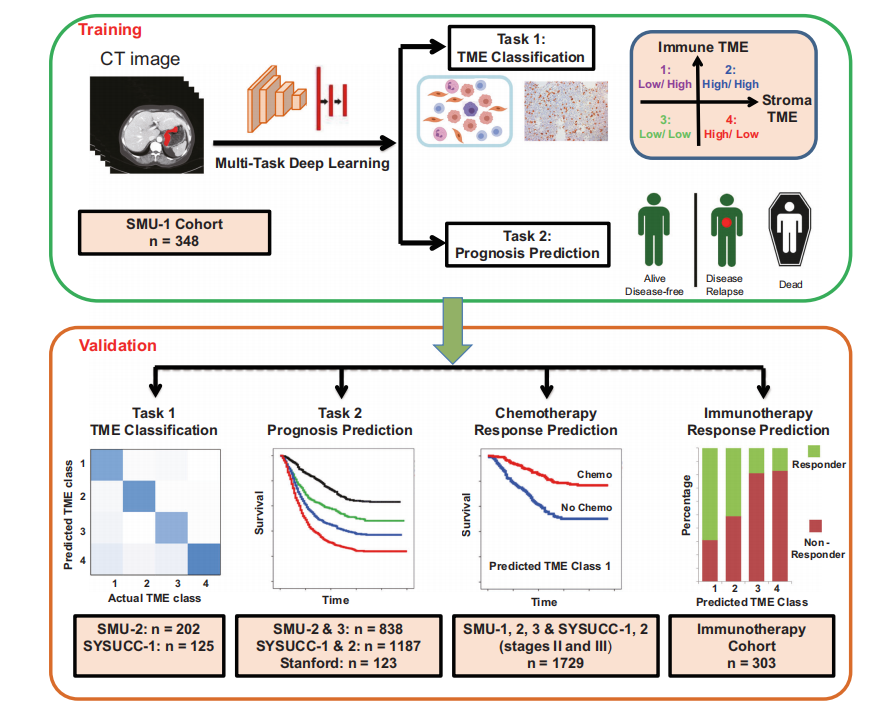
Fig. 1 | Study design for the development and validation of a deep learning model to predict TME classes and disease-free survival. Patients in the training (SMU-1) cohort and internal validation (SMU-2, 3) cohorts were recruited from Southern Medical University, Guangzhou, China. Patients in the external validation cohorts were recruited from Sun Yat-sen University Cancer Center (SYSUCC-1, 2),
Guangzhou, China and Stanford University Medical Center, Palo Alto, USA. Patients in the immunotherapy cohort were recruited from Southern Medical University, Guangzhou, China and Guangdong Provincial Hospital of Chinese Medicine,
Guangzhou, China. Both CT images and IHC-stained slides were available for patients in the SMU-1 training cohort, SMU-2 and SYSUCC-1 validation cohorts, which were used for evaluating the model’s accuracy for TME prediction. All patients had preoperative CT scans and outcomes available, which were used for testing the model’s prognostic and predictive value. CT: computer tomography;
IHC: immunohistochemistry. SMU: Southern Medical University; SYSUCC: Sun Yat sen University Cancer Center. TME: tumor microenvironment. Chemo: Chemotherapy.
图1 | 用于开发和验证预测TME类别和无病生存期的深度学习模型的研究设计。训练队列(SMU-1)和内部验证队列(SMU-2, 3)的患者来自中国广州的南方医科大学。外部验证队列的患者来自中国广州的中山大学肿瘤中心(SYSUCC-1, 2)和美国加州帕洛阿尔托的斯坦福大学医学中心。免疫疗法队列的患者来自中国广州的南方医科大学和广东省中医院。SMU-1训练队列、SMU-2和SYSUCC-1验证队列的患者都有CT图像和免疫组化(IHC)染色的切片,这些用于评估模型预测TME的准确性。所有患者都有术前CT扫描和可用的结果,这些用于测试模型的预后和预测价值。CT:计算机断层扫描;IHC:免疫组化。SMU:南方医科大学;SYSUCC:中山大学肿瘤中心。TME:肿瘤微环境。Chemo:化疗。
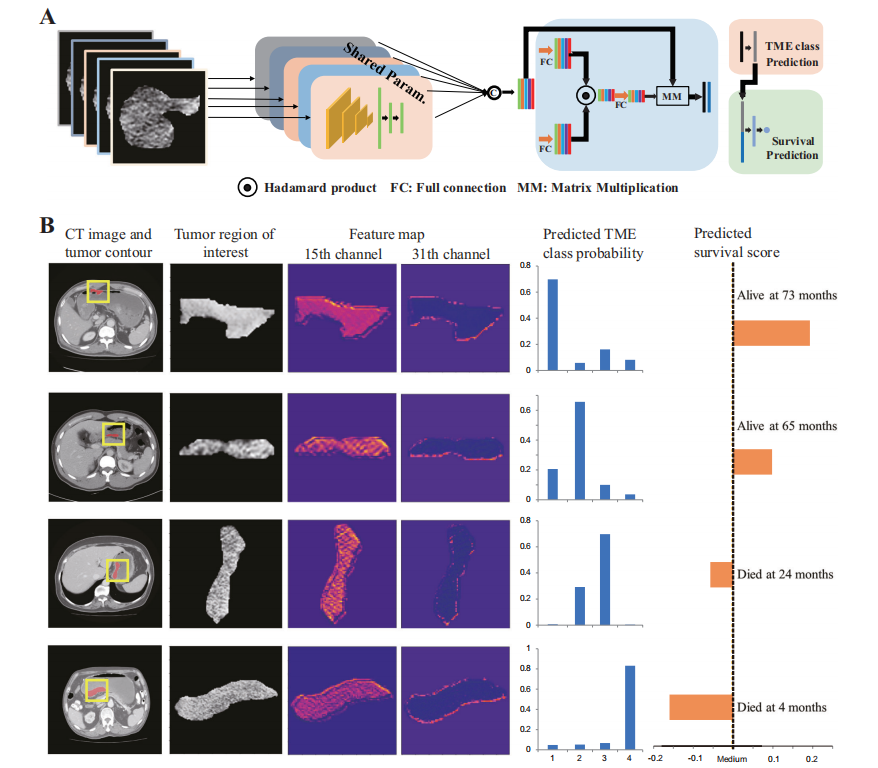
Fig. 2 | Proposed deep learning model and visualization, prediction for repre sentative cases. A Architecture of the proposed multi-task deep convolutional neural network to simultaneously classify TME and predict prognosis from CT image; (B) CT images and corresponding feature maps along with the predicted TME classes and survival scores for four representative cases, where each row corresponds to a patient with TME classes 1–4 defined by IHC. TME classes were correctly predicted for all four cases; predicted survival scores were also consistent with the actual patient outcome. TME tumor microenvironment.
图2 | 提出的深度学习模型和可视化,代表性病例的预测。A 架构为提出的多任务深度卷积神经网络,用以同时从CT图像中分类TME并预测预后;(B) CT图像及其对应的特征图,连同预测的TME类别和四个代表性病例的生存评分,其中每一行对应一位TME类别为1-4的患者,这些类别通过免疫组化(IHC)定义。所有四个病例的TME类别都被正确预测;预测的生存评分也与实际患者结果一致。TME肿瘤微环境。
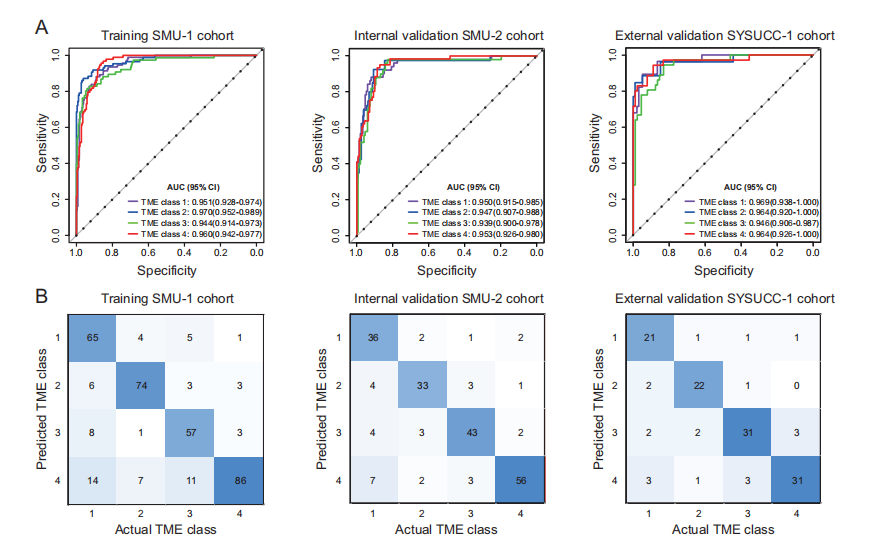
Fig. 3 | Accuracy of the deep learning model to assess TME classes. A Receiver operator characteristic (ROC) curves and (B) confusion matrices in the training SMU-1 cohort, internal validation SMU-2 cohort, and external validation SYSUCC-1 cohort. The ROC curves show the one-vs-others comparison. The confusion matrices show the pair-wise comparison; diagonal: number of cases correctly classified; off-diagonal: number of cases incorrectly classified. TME tumor micro environment, SMU Southern Medical University, SYSUCC Sun Yat-sen University Cancer Center, AUC area under the curves. Source data are provided as a Source Data file.
图3 | 深度学习模型评估TME类别的准确性。A 接收者操作特征(ROC)曲线和(B)混淆矩阵在训练SMU-1队列、内部验证SMU-2队列和外部验证SYSUCC-1队列中。ROC曲线展示了一对其他比较。混淆矩阵展示了两两比较;对角线:正确分类的病例数量;非对角线:分类错误的病例数量。TME肿瘤微环境,SMU南方医科大学,SYSUCC中山大学肿瘤中心,AUC曲线下面积。源数据提供为源数据文件。
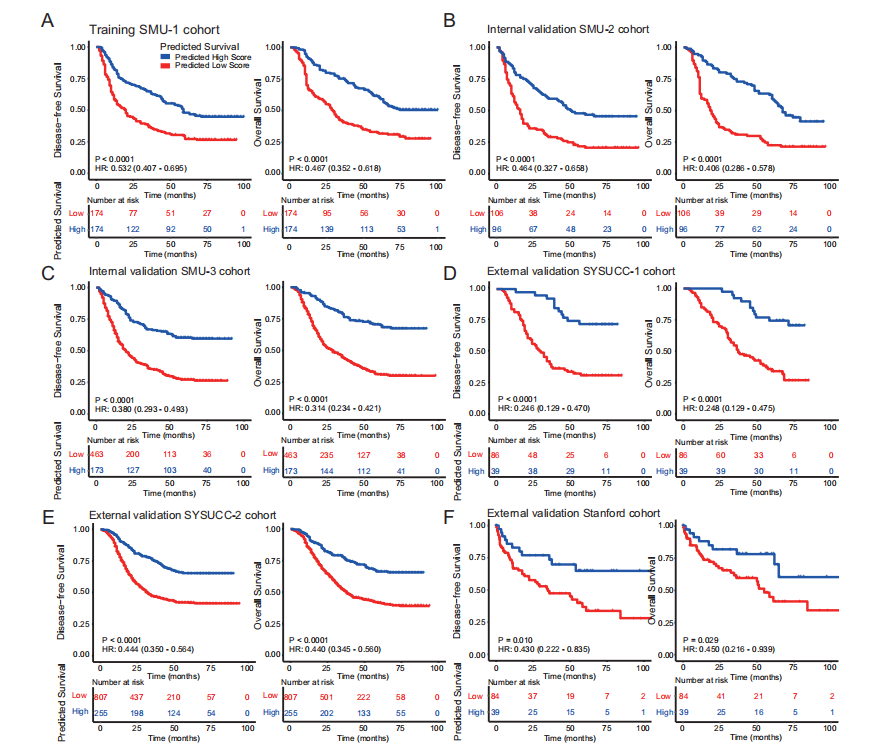
Fig. 4 | Kaplan-Meier analyses of disease-free survival (DFS) and overall survival (OS) according to the model-predicted survival score in patients with gastric cancer. A Training cohort SMU-1 (n = 348), (B) SMU-2 validation cohort (n = 202), © SMU-3 validation cohort (n = 636), (D) SYSUCC-1 validation cohort (n = 125), (E) SYSUCC-2 validation cohort (n = 1063), (F) Stanford validation cohort (n = 123).
Comparisons of the survival curves were performed with a two-sided log-rank test. SMU Southern Medical University, SYSUCC Sun Yat-sen University Cancer Center, HR Hazard ratio. Source data are provided as a Source Data file.
图4 | 根据模型预测的生存评分,胃癌患者的无病生存(DFS)和总生存(OS)的Kaplan-Meier分析。A 训练队列SMU-1(n = 348),(B) SMU-2验证队列(n = 202),© SMU-3验证队列(n = 636),(D) SYSUCC-1验证队列(n = 125),(E) SYSUCC-2验证队列(n = 1063),(F) 斯坦福验证队列(n = 123)。生存曲线的比较使用双侧log-rank测试执行。SMU 南方医科大学,SYSUCC 中山大学肿瘤中心,HR 风险比。源数据提供为源数据文件。
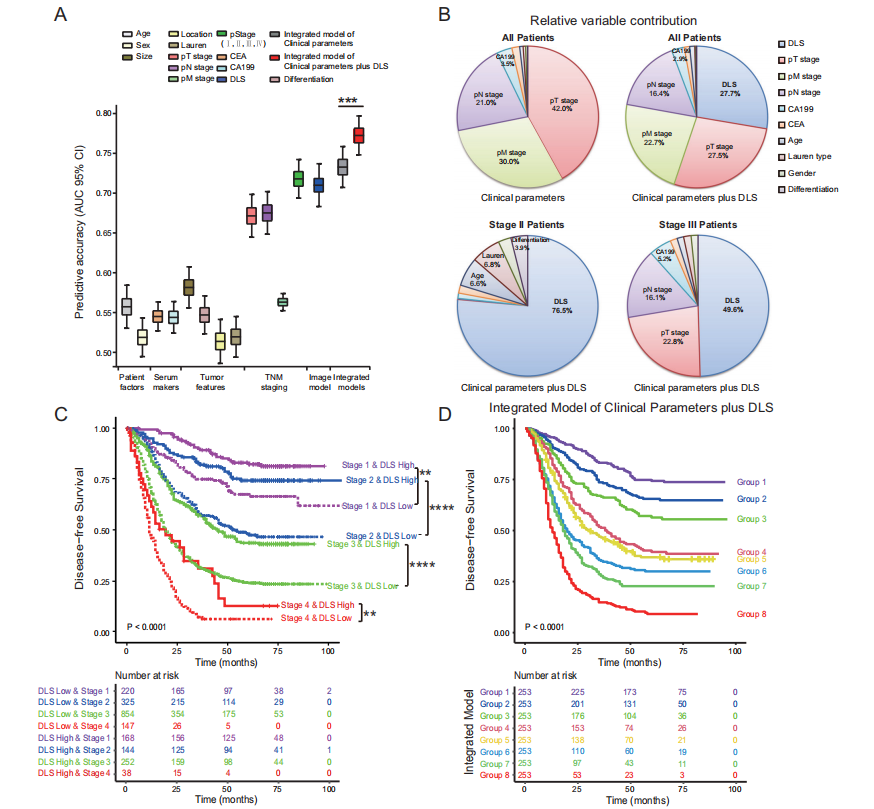
Fig. 5 | Prognosis prediction using the deep learning model and clin icopathologic risk factors. A Accuracy of prediction for disease-free survival (DFS) using clinicopathologic variable and the deep learning model (n = 2025). The center lines within the boxes represent the mean AUC value, the bounds of boxes repre sent the interquartile range (IQR) and the whiskers represent the 95% confidence intervals. B Relative variable contribution to prediction of DFS using the χ² pro portion test for clinicopathologic variables only in all patients (n = 2025); for clin icopathologic variables and DLS in all patients as well as patients with stage II and III disease. C Kaplan-Meier analysis of DFS according to the deep learning model predicted survival score within each stage in the validation cohorts (n = 2148).D Kaplan-Meier analysis of DFS according to the nomogram combining deep learning model and clinicopathologic risk factors in the validation cohorts (n = 2025). For statistical comparisons among different groups, a two-tailed t test (unpaired) was used. Comparisons of the survival curves were performed with a two-sided log-rank test. DLS deep learning survival score. P < 0.001, *P < 0.0001, P < 0.00001. Source data are provided as a Source Data file.
图5 | 使用深度学习模型和临床病理风险因素进行预后预测。A 使用临床病理变量和深度学习模型预测无病生存(DFS)的准确性(n = 2025)。箱体内的中心线代表平均AUC值,箱体的边界代表四分位距(IQR),而须代表95%置信区间。B 使用χ²比例测试,仅在所有患者(n = 2025)中对临床病理变量进行DFS预测的相对变量贡献;对所有患者以及II期和III期病患者的临床病理变量和DLS(深度学习生存评分)进行预测。C 根据深度学习模型预测的生存评分,在验证队列(n = 2148)中每个阶段的DFS的Kaplan-Meier分析。D 根据结合深度学习模型和临床病理风险因素的列线图,在验证队列(n = 2025)中DFS的Kaplan-Meier分析。对不同组之间的统计比较,使用了双尾t检验(非配对)。生存曲线的比较使用双侧log-rank测试进行。P < 0.001, *P < 0.0001, P < 0.00001。源数据提供为源数据文件。
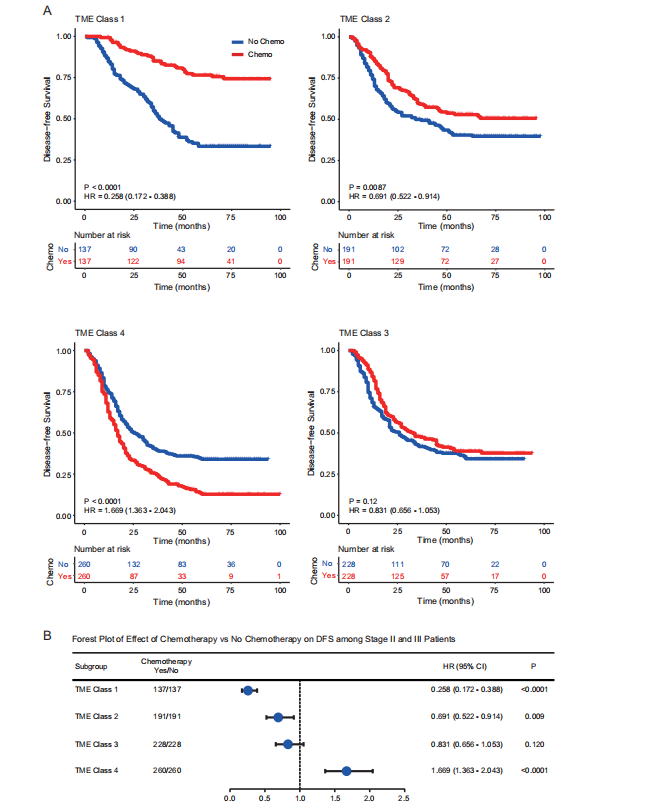
Fig. 6 | Relationship between the TME class groups and benefit from adjuvant chemotherapy in matched patients with stage II and III gastric cancer. Kaplan Meier curves of disease-free survival (DFS) for patients stratified by the receipt of chemotherapy. A TME class 1 (n = 274), TME class 2 (n = 382), TME class 3 (n = 456),TME class 4 (n = 520). B Forest plot for the effect of chemotherapy vs. no che motherapy on DFS among stage II and III patients. Comparisons of the above survival curves were performed with a two-sided log-rank test. P values reported in (B) are two-tailed from Cox proportional hazard regression analyses. Blue dot represents the HR value. Error bars represent the 95% confidence intervals. TME tumor microenvironment, DLS deep learning survival score, TME tumor micro environment, Chemo Chemotherapy, HR, Hazard ratio. Source data are provided asa Source Data file.
图6 | TME类别群体与II期和III期胃癌患者从辅助化疗中获益之间的关系。根据接受化疗与否对患者进行分层后的无病生存(DFS)的Kaplan Meier曲线。A TME类别1(n = 274),TME类别2(n = 382),TME类别3(n = 456),TME类别4(n = 520)。B 在II期和III期患者中,化疗与无化疗对DFS影响的森林图。上述生存曲线的比较使用双侧log-rank测试进行。在(B)中报告的P值是来自Cox比例风险回归分析的双尾值。蓝点代表HR值。误差条代表95%置信区间。TME肿瘤微环境,DLS深度学习生存评分,TME肿瘤微环境,Chemo化疗,HR风险比。源数据提供为源数据文件。
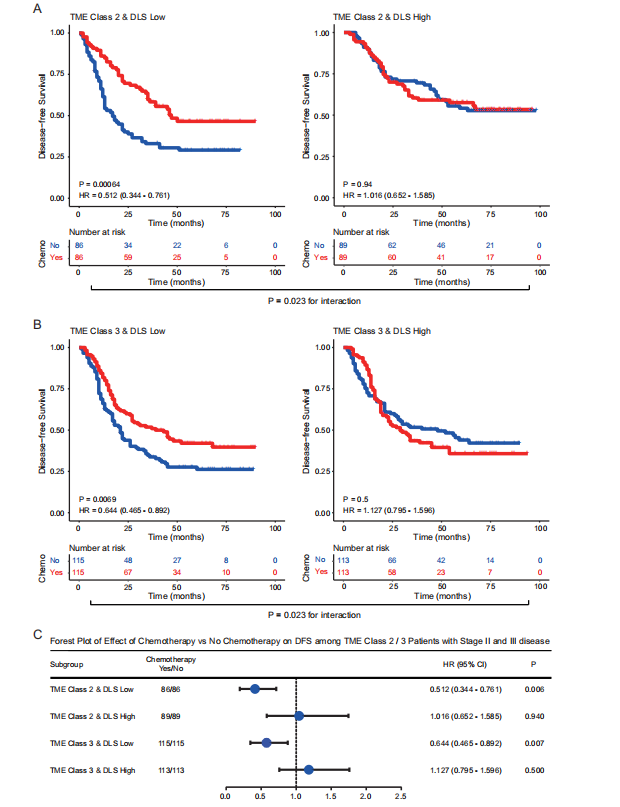
Fig. 7 | Relationship between the deep learning model and benefit from adju vant chemotherapy in matched patients with stage II and III gastric cancer.
Kaplan-Meier curves of disease-free survival (DFS) for patients stratified by the receipt of chemotherapy. A TME class 2 & DLS Low (n = 172), TME class 2 & DLS High (n = 178). B TME class 3 & DLS Low (n = 230), TME class 3 & DLS High (n = 226).
C Forest plot for the effect of chemotherapy vs. no chemotherapy on DFS among TME Class ⅔ patients with stage II and III disease. Comparisons of the above survival curves were performed with a two-sided log-rank test. P values reported in © are two-tailed from Cox proportional hazard regression analyses. Blue dot represents the HR value. Error bars represent the 95% confidence intervals. DLS
deep learning survival score, TME tumor microenvironment, Chemo Chemother apy, HR Hazard ratio. Source data are provided as a Source Data file.
图7 | 深度学习模型与II期和III期胃癌患者从辅助化疗中获益之间的关系。
根据接受化疗与否对患者进行分层后的无病生存(DFS)的Kaplan-Meier曲线。A TME类别2 & DLS低(n = 172),TME类别2 & DLS高(n = 178)。B TME类别3 & DLS低(n = 230),TME类别3 & DLS高(n = 226)。
C 在II期和III期疾病的TME类别⅔患者中,化疗与无化疗对DFS影响的森林图。上述生存曲线的比较使用双侧log-rank测试进行。在(C)中报告的P值是来自Cox比例风险回归分析的双尾值。蓝点代表HR值。误差条代表95%置信区间。DLS深度学习生存评分,TME肿瘤微环境,Chemo化疗,HR风险比。源数据提供为源数据文件。
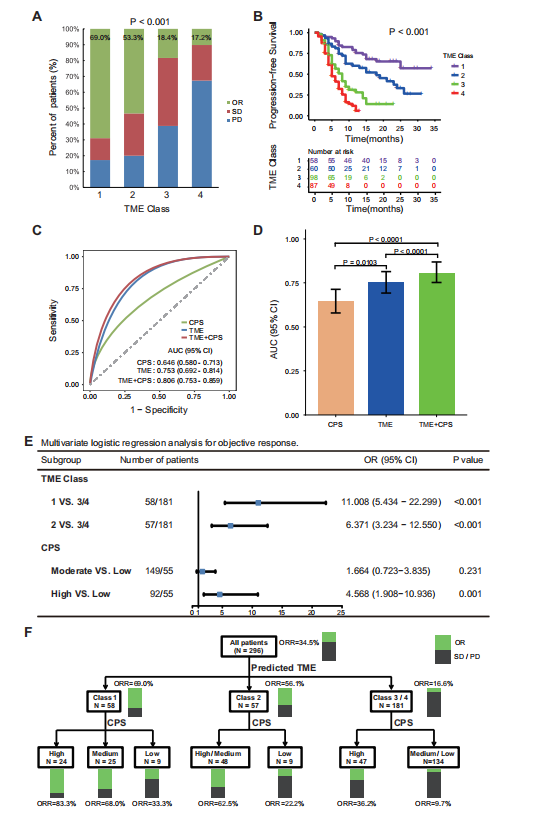
Fig. 8 | Performance of the deep learning model in predicting response and outcomes in patients treated with anti-PD-1 immunotherapy. A Response rates in patients of four TME classes predicted by the deep learning model; (B), Progression-free survival in patients of four predicted TME classes; ©, Receiver operator characteristic (ROC) curves of the predicted TME classes, CPS and com posite models combining TME classes and CPS for predicting immunotherapy response (n = 296); (D), AUC values of the predicted TME classes, CPS and com posite models combining TME classes and CPS for predicting immunotherapy response (n = 296); (E), Forest plot for the multivariate logistic regression analysis for objective response; (F), Decision tree combining the predicted TME classes and CPS. Comparisons of the survival curves were performed with a two-sided log-rank
test. Comparisons of the bar plot were performed with a two-sided t(unpaired) test. P values reported in (E) are two-tailed from logistic regression analyses. Blue dot represents the HR value. Error bars in (D) and (E) represent the 95% confidence intervals. TME tumor microenvironment, AUC area under the receiver operator characteristic curve, CPS combined positive score of PDL1 expression, OR objective response (complete and partial response), SD stable disease, PD progressive disease. Source data are provided as a Source Data file.
图8 | 深度学习模型在预测接受抗PD-1免疫疗法患者的反应和结果中的表现。A 四种TME类别患者的深度学习模型预测反应率;(B) 四种预测TME类别患者的无进展生存期;© 预测TME类别、CPS和结合TME类别与CPS的复合模型在预测免疫疗法反应中的接收者操作特征(ROC)曲线(n = 296);(D) 预测TME类别、CPS和结合TME类别与CPS的复合模型在预测免疫疗法反应中的AUC值(n = 296);(E) 客观反应的多变量逻辑回归分析的森林图;(F) 结合预测的TME类别和CPS的决策树。生存曲线的比较使用双侧log-rank测试进行。条形图的比较使用双侧t(非配对)测试进行。在(E)中报告的P值是来自逻辑回归分析的双尾值。蓝点代表HR值。在(D)和(E)中的误差条代表95%置信区间。TME肿瘤微环境,AUC接收者操作特征曲线下面积,CPS PDL1表达的综合阳性评分,OR客观反应(完全和部分反应),SD稳定疾病,PD进展性疾病。源数据提供为源数据文件。