01
文献速递介绍
虽然深度学习在医疗保健研究中产生了显著影响,但其在医疗保健领域的影响无疑比在其他应用领域更慢、更有限。造成这种情况的一个重要原因是,由于患者隐私问题,可供更广泛的机器学习研究社区使用的患者数据相对匮乏。尽管医疗服务提供商、政府和私营企业越来越多地以电子方式收集大量且多样化的患者数据,这些数据对科学家来说可能极其宝贵,但由于患者隐私问题,这些数据通常无法被更广泛的研究社区使用。此外,即使研究人员能够获得此类数据,确保适当的数据使用和保护也是一个受到严格法律要求管控的漫长过程。这可能显著减缓研究的步伐,从而影响这些研究对患者护理的好处。高质量和真实感的合成数据集可以用来加速医学领域的方法论进步(Dube 和 Gallagher,2013;Buczak等,2010)。虽然有为电子健康记录生成医疗数据的方法(Dube和Gallagher,2013;Buczak等,2010),但由于医学图像是高维的,医学图像合成的研究更加困难。随着生成对抗网络(GAN)(Goodfellow等,2014)的发展,高维图像生成成为可能。特别是,条件GAN(Mirza和Osindero,2014)可以生成基于给定模式的图像,例如,根据它们的标签构建图像来合成带标签的数据集。在医学图像合成领域,Teramoto等(2020)提出了一种渐进式生长条件GAN来生成肺癌图像,并得出结论,合成图像可以辅助深度卷积神经网络训练。Yu等(2021)最近使用条件GAN生成的宫颈细胞分类中的异常细胞图片来解决类不平衡问题。此外,Shorten和Khoshgoftaar(2019)对基于GAN的论证在医学图像中的作用进行了全面的调查。
Title
题目
Backdoor attack and defense in federated generative adversarial network-based medical image synthesis
联邦生成对抗网络基础医学图像合成中的后门攻击与防御
Methods
方法
The goal of medical image synthesis is to generate high-quality synthetic data that can be employed for open research to argue the limited datasets and balance the training data, and finally hasten DL methodological advancements in medicine. In our study, we explore conditional GAN in the FL setting, i.e., conditional FedGAN. In this section, we first introduce our setting for FedGAN in Section 3.1 Next, we discuss the scope for adversarial attacks and determine the best way to implement the backdoor attack that involves data poisoning in Section 3.2. Then, we suggest the potential strategies for defending against such attack in FedGAN to build a robust FL system in Section 3.3.
医学图像合成的目标是生成高质量的合成数据,这些数据可以用于公开研究,以弥补有限的数据集和平衡训练数据,最终加速医学中的深度学习方法论进步。在我们的研究中,我们探索了在联邦学习(FL)设置中的条件生成对抗网络,即条件 FedGAN。在这一部分,我们首先在第3.1节介绍我们对 FedGAN 的设置。接下来,在第3.2节,我们讨论对抗性攻击的范围,并确定实施涉及数据中毒的后门攻击的最佳方法。然后,在第3.3节中,我们提出在 FedGAN 中防御此类攻击的潜在策略,以构建一个健壮的 FL 系统。
Conclusions
结论
Motivated by the idea of backdoor attacks in classification models, this work investigates the pitfalls of backdoor attacks in training conditional FedGAN models. We conduct extensive experiments to investigate the backdoor attack on two public datasets and evaluate among different types and sizes of triggers. Based on our key observations on malicious clients’ loss patterns, we propose FedDetect as an effective defense strategy against backdoor attacks in FedGAN. We comprehensively conduct quantitative and qualitative assessments on the fidelity and utility of the synthetic images under different training conditions. We demonstrate the FedDetect significantly outperforms the alternative baselines and preserves comparable data utility as attack-free vanilla FedGAN. As the first step towards understanding backdoor attacks in FedGAN for medical image synthesis, our work brings insight into building a robust and trustworthy model to advance medical research with synthetic data. Furthermore, we hope to highlight that FedDetect involves only lightweight improvement on the server aggregation step. This makes FedDetect flexible to integrate into different GAN-based federated generative models. Our future work includes scaling up the FL system with more clients, generalizing FedDetect to other deep generative models, e.g., Diffusion models (Song et al., 2021), and considering other variants of backdoor attacks, e.g., frequency-injection based attack (Feng et al., 2022).
受分类模型中后门攻击思想的启发,本工作研究了在训练条件 FedGAN 模型时后门攻击的陷阱。我们进行了广泛的实验,以研究两个公共数据集上的后门攻击,并评估不同类型和大小的触发器。基于我们对恶意客户端损失模式的关键观察,我们提出 FedDetect 作为一种有效的防御策略,以抵御 FedGAN 中的后门攻击。我们全面进行定量和定性评估,以检验在不同训练条件下合成图像的忠实度和实用性。我们展示了 FedDetect 显著优于其他替代基线,并保留了与无攻击的原始 FedGAN 相当的数据实用性。作为理解医学图像合成中 FedGAN 后门攻击的第一步,我们的工作为构建健壮且值得信赖的模型提供了洞见,以便利用合成数据推进医学研究。此外,我们希望强调 FedDetect 仅涉及服务器聚合步骤的轻量级改进。这使得 FedDetect 可以灵活地集成到不同的基于 GAN 的联邦生成模型中。我们未来的工作包括扩大 FL 系统,增加更多客户端,将 FedDetect 推广到其他深度生成模型,例如扩散模型(Song 等,2021),并考虑其他变体的后门攻击,例如基于频率注入的攻击(Feng 等,2022)。
Figure
图
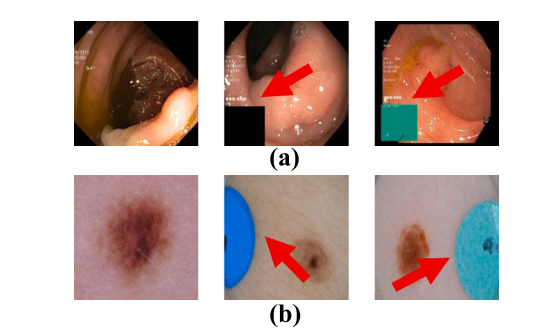
Fig. 1. Example medical images with backdoor-alike noisy patches from (a)KVASIR dataset (Pogorelov et al., 2017) (b) ISIC dataset (Codella et al., 2018).
图 1. 来自 (a) KVASIR 数据集(Pogorelov 等,2017年)(b) ISIC 数据集(Codella 等,2018年)的带有类似后门的噪声贴片的医疗图像示例。
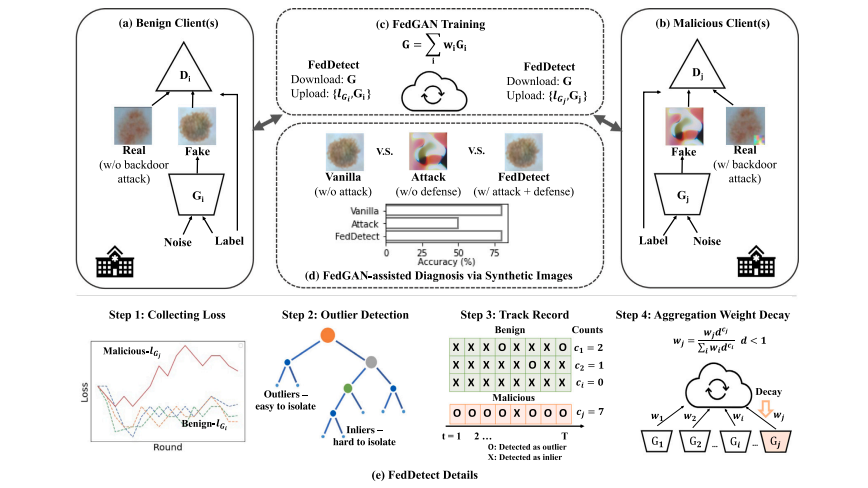
Fig. 2. The overview of our proposed framework. Specifically, our FedGAN scenario consists of benign clients shown in (a), malicious clients shown in (b), and a global conditional FedGAN server as illustrated in ©. During the training, the malicious discriminator is fed with poisoned images. The discriminator and generator are trained against each other locally following the training protocol enforced by the FedGAN organizer. The generators upload their training loss and aggregate parameters per global iteration as described in ©. In the end, we expect the server generator to produce high-quality images for medical research in terms of fidelity and diversity to assist medical diagnosis tasks as demonstrated as Vanilla in (d). However, the attack degrades the central generator’s performance, generating noisy images with little value for medical diagnosis. We propose FedDetect to defend against such attack. As illustrated in (e), FedDetect consists of four steps. First, the FL server collects the local generator loss and then passes them to the isolation forest for anomaly detection. Because outliers are located distantly from inliers, they are supposed to be isolated at the early stage in the construction of the isolation trees. The outliers are flagged as potential adversarial and the server tracks the record of the number of each client being flagged. Finally, the FedGAN server decays the weight of those potential malicious clients based on the track record per round.
图 2. 我们提出的框架概览。具体来说,我们的 FedGAN 方案包括 (a) 中所示的良性客户端,(b) 中所示的恶意客户端,以及 © 中所示的全局条件 FedGAN 服务器。在训练过程中,恶意鉴别器被喂入被污染的图像。鉴别器和生成器在 FedGAN 组织者强制执行的训练协议下本地对抗训练。生成器上传它们的训练损失和每次全局迭代的聚合参数,如 © 所描述。最终,我们期望服务器生成器为医学研究产生高质量的图像,以忠实度和多样性方面协助医学诊断任务,如 (d) 中的 Vanilla 所演示。然而,攻击降低了中央生成器的性能,生成对医学诊断价值不大的带噪声图像。我们提出 FedDetect 来抵御这种攻击。如 (e) 所示,FedDetect 包括四个步骤。首先,FL 服务器收集本地生成器损失,然后将它们传递给孤立森林进行异常检测。由于异常值远离正常值,它们应该在构建孤立树的早期阶段被孤立。这些异常值被标记为潜在的对抗性,服务器记录每个客户端被标记的次数。最后,FedGAN 服务器根据每轮的记录降低这些潜在恶意客户端的权重。
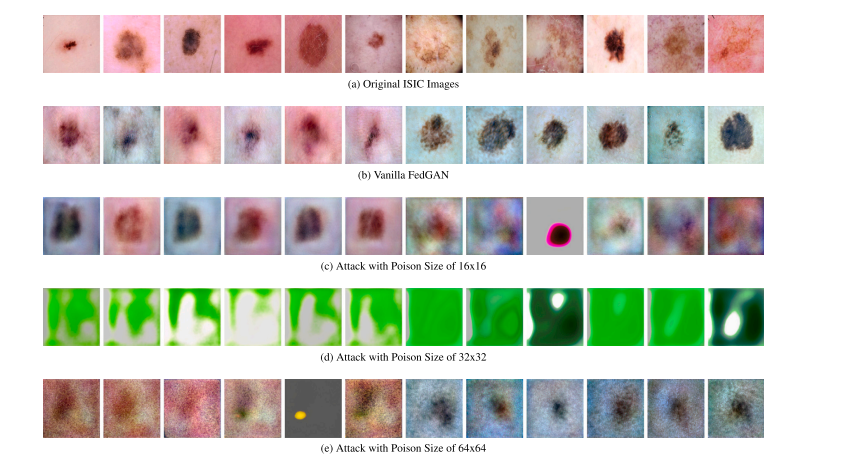
Fig. 3. Visualization on ISIC attacking.
图 3. ISIC 攻击的可视化展示。
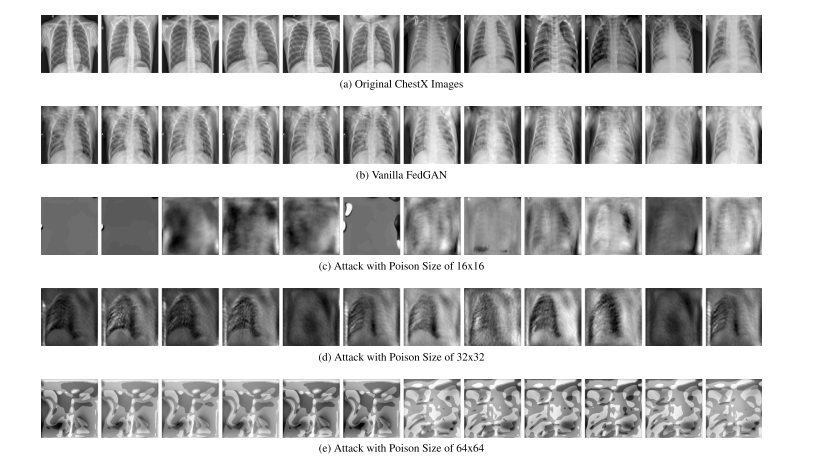
Fig. 4. Visualization on ChestX attacking.
图 4. ChestX 攻击的可视化展示。
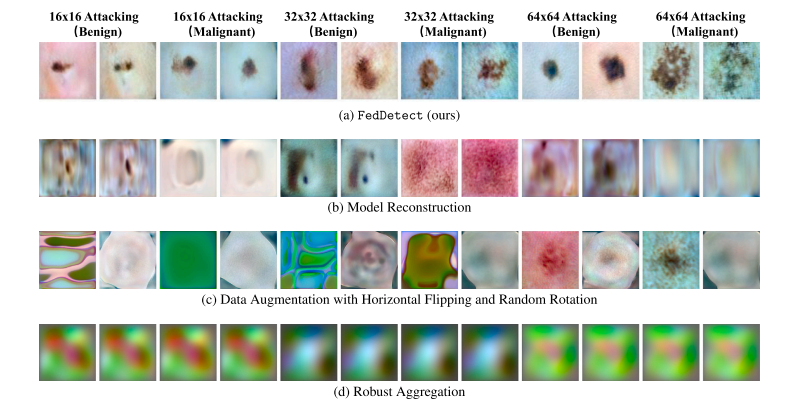
Fig. 5. Visualization on different defense strategies for ISIC.
图 5. 对 ISIC 不同防御策略的可视化展示。
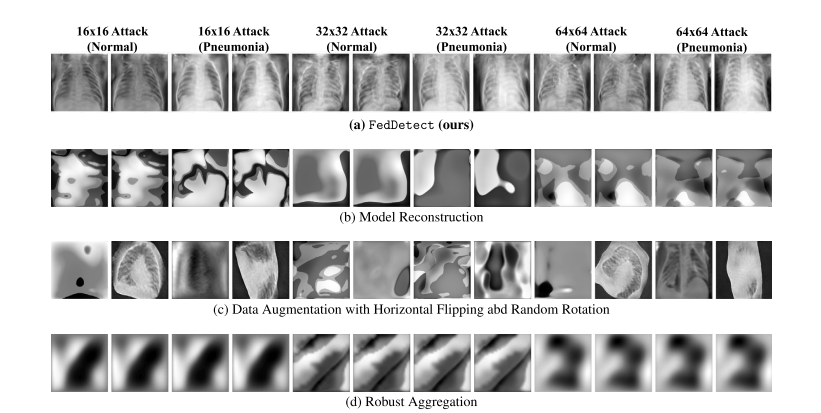
Fig. 6. Visualization on different defense strategies for ChestX.
图 6. 对 ChestX 不同防御策略的可视化展示。
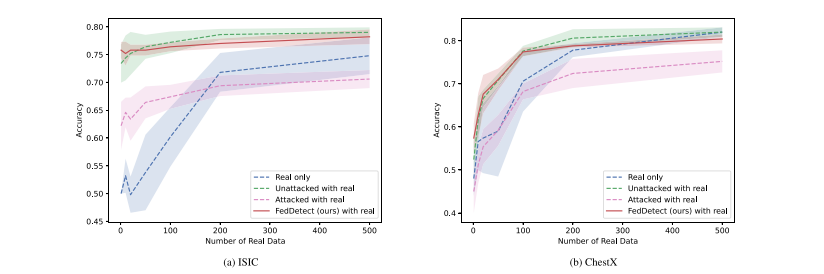
Fig. 7. Classification accuracy on synthetic data augmentation with different number of real training data per class.
图 7. 使用不同数量的每类真实训练数据进行合成数据增强的分类准确性。
Table
表
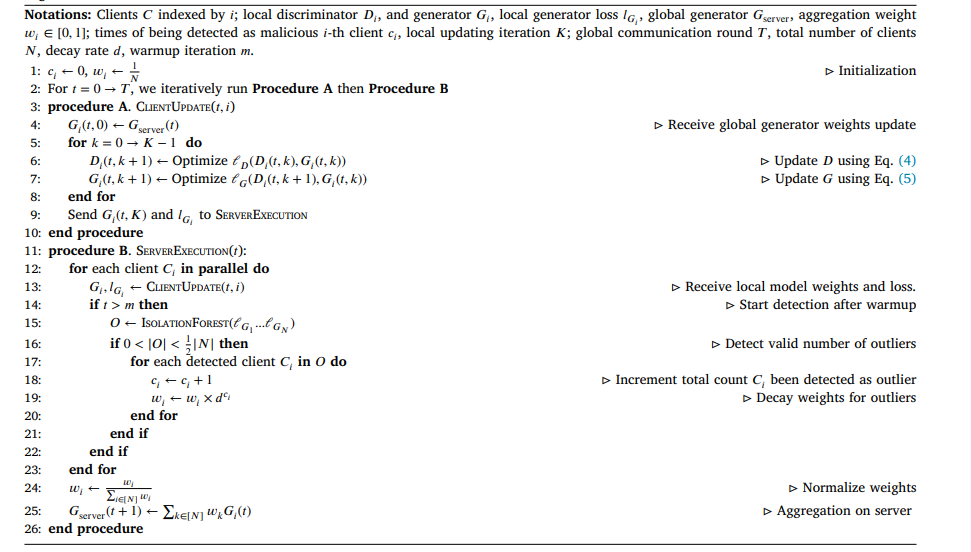
Algorithm 1 FedDetect
算法 1 FedDetect
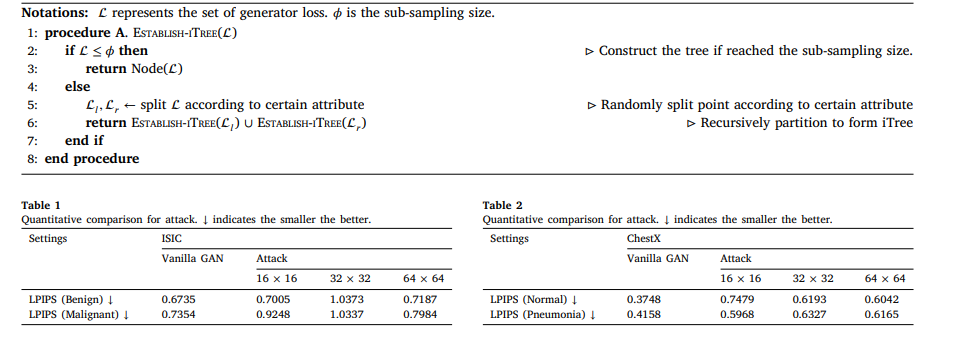
Algorithm 2 Establish iTree
算法 2 建立 iTree(孤立树)

Table 3 Quantitative metrics of defense for ISIC. ↓ indicates the smaller the better.
表 3 ISIC 防御的定量指标。↓ 表示数值越小越好。

Table 4 Quantitative metrics of defense for ChestX. ↓ indicates the smaller the better
表 4 ChestX 防御的定量指标。↓ 表示数值越小越好。