本笔记来源于B站Up主: 有Li 的影像组学系列教学视频
本节(37)主要介绍一篇文献,关于 机器学习模型判断脑卒中发病时间
文献题目:Machine Learning Approach to Identify Stroke Within 4.5 Hours
2019年12月发表在 Stoke 上。
研究的目的:
to investigate the ability of machine learning techniques analyzing diffusion weighted imaging (DWI) and fluid-attenuated inversion recovery (FLAIR) MRI to identify patients within the recommended time window for thrombolysis.
由于专业的不同,重点学习一下这篇文献的方法学部分及相应的结果描述吧!
方法(Methods)
1、首先是研究的整体流程图
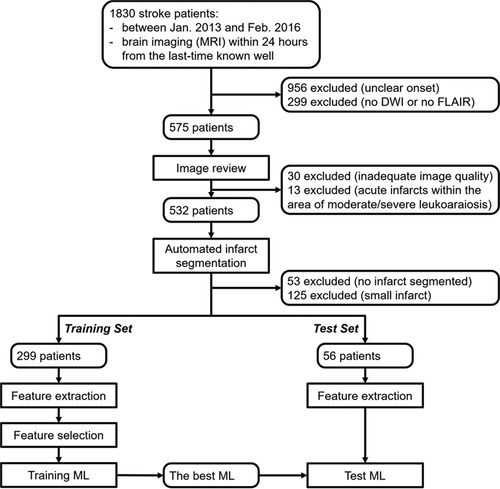
2、图像主要处理流程为:
A. Infarct regions were automatically segmented on the ADC maps by applying normalized absolute thresholding.
B. A quantile curve of ADC intensities within the brain mask was constructed for each ADC map of each subject, and an intersection point between 2 tangent lines with maximum and minimum differential coeffcients was identifed on each quantile-intensity curve. (这句没有看懂,大概意思是交代自动分割阈值是如何选择的)
C. The ADC maps were normalized
D. Each normalized ADC map was thresholded at the optimal absolute value of 0.845.
E. FLAIR images were coregistered onto ADC maps.
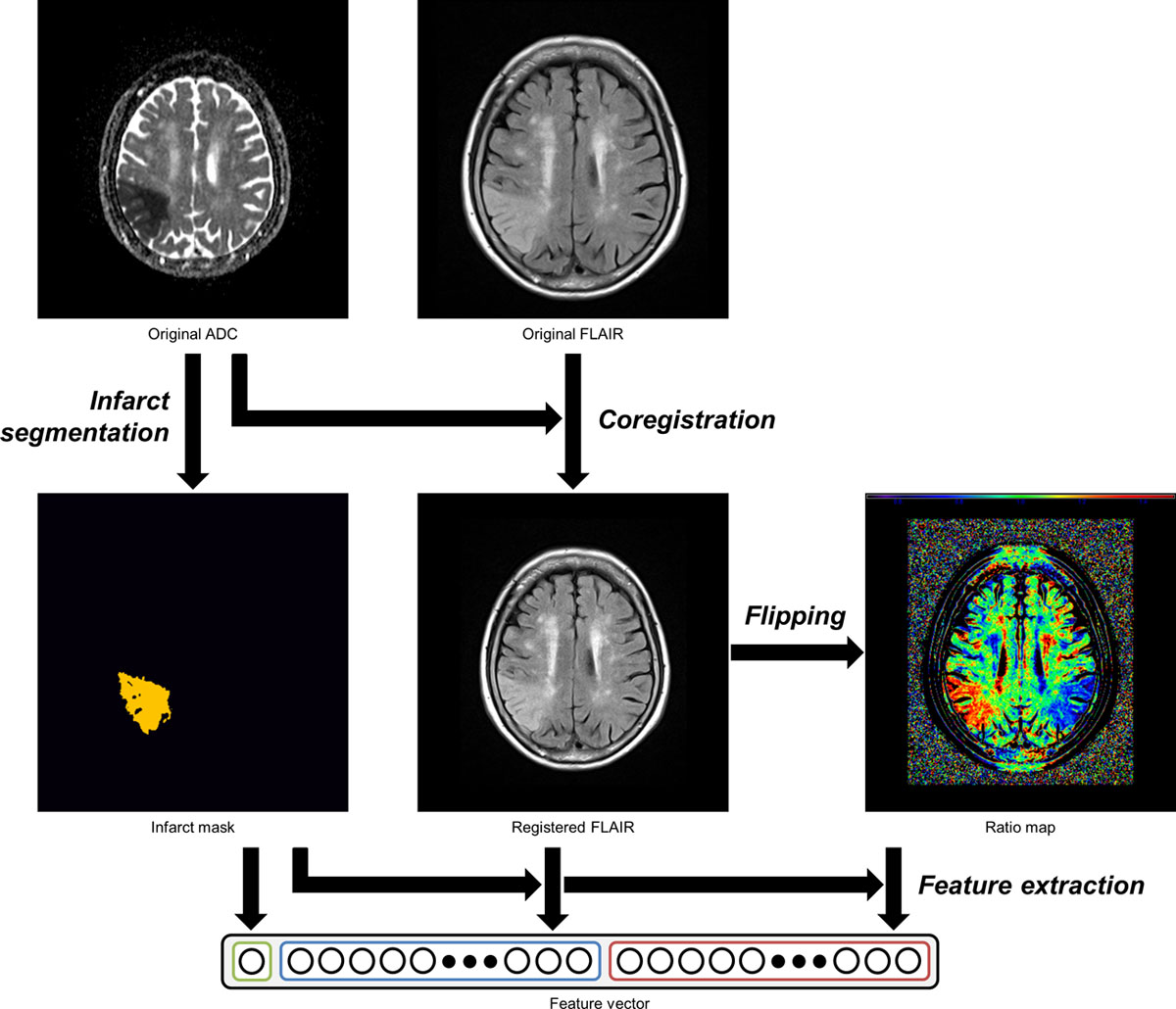
3、Generation of Ratio Maps
Ratio maps were constructed by reflecting the image around the ftted midsagittal plane, resulting in quantitative comparisons of the relative signals of the infarct regions and the contralateral side.
4、Extraction of Imaging Features
… extracted from the registered FLAIR images and FLAIR ratio maps included intensity, gradient, and texture information…
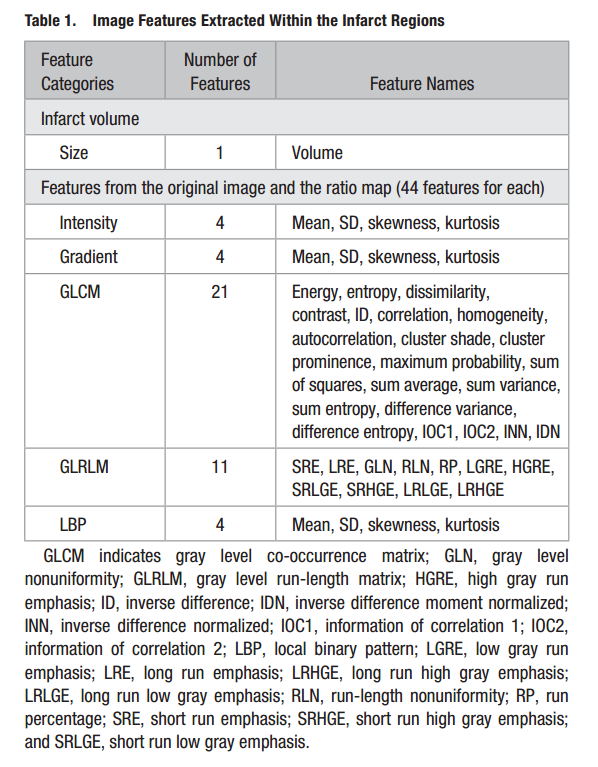
5、Machine Learning
交代了使用的机器学习算法,以及它们各自的特点。
…cluster analysis including LR, and modern classifcation theories including SVM which
needs feature selection and RF which needs not feature selection
6、Human Visual Assessment of DWI-FLAIR Mismatch
描述了人类视觉评价的过程,以及mismatch的定义
A DWI-FLAIR mismatch was defned as the presence of a visible acute ischemic lesion on DWI but no traceable parenchymal hyperintensity in the corresponding region on FLAIR imaging
7、统计方法描述:
A. 研究者使用了单因素t检验对89个特征进行筛选,并使用了Bonferroni correction对p值进行了校正。(这里研究者把p值设在了0.2,如果是常规的0.05,然后再除以89,会非常小);如果校正后特征数目小于5个,则根据p值排序,选择前5个。
B. 机器学习模型和人类视觉的表现差异。
C. Youden index来决定cutoff 值(但是后文好像没有交代结果)。
结果(Results):
首先对分组情况(training set, test set)进行了描述,基线资料、target的分布等。
自然,两组之间没有什么统计学差异。
单因素分析进行特征筛选,共筛选到34个特征用于ML建模(LR, SVM,RF)。
每个类别中表现最优的用于测试集中进行评价。尽管RF的AUROC最大,但是三者之间并没有统计学差异(如图)
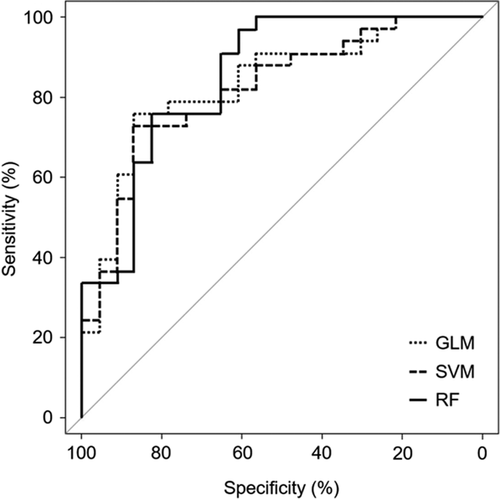
和人类视觉判断相比,ML模型在sensitivity和NPV上表现优良,但在specificity和PPV上略逊一筹。
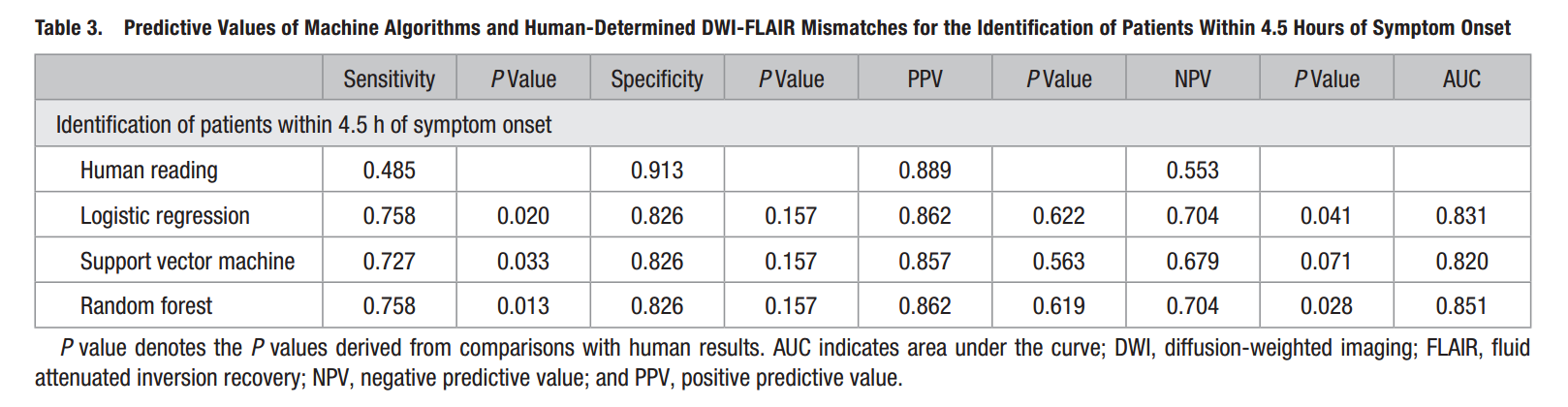
拓展:
在仔细研读文献的统计方法部分时,发现在机器学习领域,有很多统计方法和我们平时用的不太一样。
The Bonferroni correction compensates for that increase by testing each individual hypothesis at a significance level of α/m, where α is the desired overall alpha level and m is the number of hypotheses. For example, if a trial is testing m=20 hypotheses with a desired α=0.05, then the Bonferroni correction would test each individual hypothesis at α=0.05/20=0.0025.(from wikipedia)
1、Bonferroni correction
可以称作是“最简单粗暴有效”的校正方法,它拒绝了所有的假阳性结果发生的可能性,通过对p值的阈值进行校正来实现消除假阳性结果,但是该方法比较保守,容易错误地接受零假设。用的更多的是Holm’s-Bonferroni,比前者要更容易发现显著差异。(知乎@123456@Sichao Song)
Sensitivit and specifcity were compared using McNemar tests, and positive predictive value and negative predictive value were compared using the Generalized Score Statistics method, as appropriate.
2、McNemar test 和卡方检验的区别:
The McNemar is not testing for independence, but consistency in responses across two variables.
Although Chi-Square tests can be used for larger tables, McNemar tests can only be used for a 2×2 table.
3、这里的the Generalized Score Statistics method应该和 Generalized score tests 是一回事(没有找到相应的中文名)。它属于半参数方法,不受总体分布影响;最常用于重复测量数据。
Positive and negative predictive values are important measures of a medical diagnostic test performance. We consider testing equality of two positive or two negative predictive values within a paired design in which all patients receive two diagnostic tests. The existing statistical tests for testing equality of predictive values are either Wald tests based on the multinomial distribution or the empirical Wald and generalized score tests within the generalized estimating equations (GEE) framework. As presented in the literature, these test statistics have considerably complex formulas without clear intuitive insight. (ResearchGate@Andrzej S Kosinski)
参考资料:
如何计算McNemar检验,比较两种机器学习分类器
The Difference Between a Chi-Square Test and a McNemar Test