Title
题目
AutoFOX: An automated cross-modal 3D fusion framework of coronary X-ray angiography and OCT
AutoFOX:一种冠状动脉X线造影与OCT的自动化跨模态3D融合框架
01
文献速递介绍
冠状动脉疾病(CAD)仍是全球范围内的主要死亡原因(Martin等, 2024)。经皮冠状动脉介入治疗(PCI)是CAD的主要诊断和治疗手段,其中冠状动脉X线造影(XA)和光学相干断层成像(OCT)被用于提供互补信息以指导PCI(Räber等, 2018)。如图1所示,XA能够直观评估冠状动脉的整体解剖结构,而OCT则通过超高分辨率的成像技术详细评估血管壁内腔和斑块成分(如钙化、脂质和纤维)的内部形态(Bezerra等, 2009)。将XA与OCT相融合能够提供互补的信息,增强对冠状动脉解剖和斑块形态的理解,在CAD的诊断和预后中发挥重要作用。
理想的三维融合模型应能够准确提供主血管(MV)的形态细节以及侧支(SB)开口的解剖结构,这对于实现准确的血流动力学评估(如内皮剪切应力(ESS)Li等, 2018; Kweon等, 2018)和计算生理学评估具有重要意义。
现有的融合方法可分为“基于导丝”(wire-based)和“非导丝”(wireless)两大类。“基于导丝”的方法通过追踪介入设备(如导丝上的成像探头)标记对准点,通常结合技术如心电图触发的透视成像与血管内成像导丝回拉技术来跟踪X线下的探头位置(Wang等, 2013; Prasad等, 2016; Wu等, 2023),或者使用定制的系统记录标志点(Houissa等, 2019)。然而,这类方法仅适用于特定追踪设置下采集的数据,可能增加辐射剂量,并需要额外的手动操作或设备,增加了PCI工作流程的复杂性。
相比之下,“非导丝”的方法完全基于原始图像,无需改变导管室的标准操作流程。这些方法可以广泛应用于以往的数据回顾性分析。然而,现有的“非导丝”方法通常依赖于简单的等距映射(Wahle等, 2006; Tu等, 2011; Wang等, 2018),这可能限制其对复杂血管解剖结构的准确对准能力。
Aastract
摘要
Coronary artery disease (CAD) is the leading cause of death globally. The 3D fusion of coronary X-rayangiography (XA) and optical coherence tomography (OCT) provides complementary information to appreciatecoronary anatomy and plaque morphology. This significantly improve CAD diagnosis and prognosis byenabling precise hemodynamic and computational physiology assessments. The challenges of fusion lie inthe potential misalignment caused by the foreshortening effect in XA and non-uniform acquisition of OCTpullback. Moreover, the need for reconstructions of major bifurcations is technically demanding. This paperproposed an automated 3D fusion framework AutoFOX, which consists of deep learning model TransCAN for3D vessel alignment. The 3D vessel contours are processed as sequential data, whose features are extractedand integrated with bifurcation information to enhance alignment via a multi-task fashion. TransCAN showsthe highest alignment accuracy among all methods with a mean alignment error of 0.99 ± 0.81 mm along thevascular sequence, and only 0.82 ± 0.69 mm at key anatomical positions. The proposed AutoFOX frameworkuniquely employs an advanced side branch lumen reconstruction algorithm to enhance the assessment ofbifurcation lesions. A multi-center dataset is utilized for independent external validation, using the paired 3Dcoronary computer tomography angiography (CTA) as the reference standard. Novel morphological metrics areproposed to evaluate the fusion accuracy. Our experiments show that the fusion model generated by AutoFOXexhibits high morphological consistency with CTA. AutoFOX framework enables automatic and comprehensiveassessment of CAD, especially for the accurate assessment of bifurcation stenosis, which is of clinical value toguiding procedure and optimization.
冠状动脉疾病(CAD)是全球范围内的主要死亡原因。冠状动脉X线造影(XA)与光学相干断层成像(OCT)的三维融合能够提供互补的信息,以更好地了解冠状动脉解剖结构和斑块形态学。这种融合通过实现精确的血流动力学和计算生理学评估,显著提高了冠状动脉疾病的诊断与预后。然而,融合面临的挑战包括:由于XA中的缩短效应以及OCT回拉过程中的非均匀采集导致的潜在对准误差。此外,重建主要分叉处的复杂性对技术要求极高。
本文提出了一种自动化的三维融合框架——AutoFOX,它包含了用于三维血管对准的深度学习模型TransCAN。三维血管轮廓被处理为序列数据,其特征通过多任务方式提取并结合分叉信息来增强对准。TransCAN在所有方法中显示出最高的对准精度,沿血管序列的平均对准误差为0.99 ± 0.81 mm,在关键解剖位置仅为0.82 ± 0.69 mm。
提出的AutoFOX框架独特地采用了先进的侧支腔重建算法,以增强对分叉病变的评估能力。框架使用多中心数据集进行独立的外部验证,以配对的三维冠状动脉CT造影(CTA)作为参考标准。我们提出了新的形态学指标来评估融合精度。实验表明,AutoFOX生成的融合模型在形态学上一致性高,与CTA具有显著的相似性。
AutoFOX框架能够实现冠状动脉疾病的自动化和全面评估,尤其在分叉狭窄的准确评估方面具有临床价值,可用于指导手术操作和优化治疗方案。
Method
方法
To achieve automated cross-modal fusion, a series of necessarysteps need to be implemented. First, coronary vessel models for eachimage modality must be reconstructed separately. Then, their correspondences are established by treating reconstructed vessels as sequential data. To establish optimal longitudinal correspondence betweensequences, we adapt our previously proposed two-stage (coarse andfine) alignment strategy (Qin et al., 2021) to 3D alignment task, furtherenhancing it with deep learning models. Next, the relative axial rotational angle between vessels is determined. Finally, the reconstructionof the fusing model in 3D space is completed based on the alignmentresults, with optimized bifurcation structures. We proposed AutoFOXto implement the above cross-model fusion steps through three fullyautomated procedures, i.e., Initial Reconstruction (IR), Co-Registration(CR), and Fusion Reconstruction (FR). The AutoFOX workflow hasalready been implemented in a prototype software owned by ShanghaiJiao Tong University, with can be used to reproduce the data of thisstudy.
为了实现自动化的跨模态融合,需要实施一系列必要的步骤。首先,需分别重建每种图像模态的冠状动脉血管模型。然后,将重建的血管视为序列数据,建立它们之间的对应关系。为了在序列之间建立最佳的纵向对应关系,我们将之前提出的两阶段(粗略和精细)对准策略(Qin等, 2021)适配到三维对准任务中,并通过深度学习模型进一步优化。接着,确定血管之间的相对轴向旋转角度。最后,基于对准结果完成三维空间中的融合模型重建,并优化分叉结构。
我们提出了AutoFOX框架,通过三个全自动化步骤实现上述跨模态融合过程,即初始重建(IR)、配准(CR)和融合重建(FR)。AutoFOX工作流程已在上海交通大学拥有的原型软件中实现,该软件可用于复现本研究的数据。
Conclusion
结论
This paper presents AutoFOX, a fully automated cross-modal 3Dfusion framework for coronary X-ray Angiography and OCT throughthree procedures: Initial Reconstruction, Co-Registration, and FusionReconstruction. AutoFOX overcomes the limitations of existing vascular3D alignment and fusion methods with a dedicated designed multitask model TransCAN. AutoFOX treats vascular contours as sequentialdata, straightens 3D-XA to remove redundant curvature, and reducesmodel parameters through 3D to 1D transformation. Furthermore, thereconstruction algorithm is refined for SB lumen, which enhances theassessment of bifurcation lesions.
TransCAN shows the highest alignment accuracy compared withother methods. The novelty of the TransCAN lies in the deep integrationof SB information: The SB-Matching sub-task enhances the matching of SB features; The BPEG module provides SB-weighted relativeposition encoding; and the SPSP-attention reduces the computationalcomplexity of cross-attention while effectively maintaining informationinteraction at the SBs. Two TransCAN modes, nTransCAN and softTransCAN are proposed and compared. The former achieves optimalaccuracy at the overall sequence level, while the latter performs betterat clinically key positions and has greater scalability in larger datasets.The proposed CSA loss effectively enhances model robustness. Althoughsome modules bring only minor numerical improvements, they are stillof great clinical significance considering their proportion in the smalllesion length and SB ostium length. Ultimately, we evaluate the fusionmodel with an independent multi-center dataset through 5 morphological metrics using the paired CTA as the reference standard. Highmorphological consistency is observed between the CTA and the fusionmodel generated by AutoFOX, especially for clinically significant BOAand BMLA. In addition to smaller difference in absolute luminal measurements, the improved correlation with CTA further demonstratesthat the integration of OCT enhances the fusion model’s consistencywith the actual lumen structure.
One major advantage of our work is the full automation of the entireframework without the need of manual intervene. This is contributedby the special designed module within AutoFOX, making it robust tothe potential noise of the upstream output. Finally, the advanced 3D fusion model offers significant application value in guiding percutaneouscoronary intervention for CAD patients, particularly in complex bifurcation lesions. By enhancing lesion visualization, this technology enablescardiologists to make more informed decisions during procedures, optimizing treatment strategies and potentially reducing complications.Given the accumulated evidence supporting imaging-based computational physiology assessments, the fusion of OCT and XA can enhancethe evaluation accuracy of key parameters such as fractional flowreserve (FFR) and endothelial shear stress (ESS) by utilizing the precisegeometry modeling provided by Auto-FOX. Furthermore, the fused 3Dcoronary artery tree also paves the way for investigating hemodynamicmechanisms in CAD development, potentially uncovering novel insightsinto disease progression and informing more targeted therapeutic interventions. As for the analysis speed by AutoFOX, although the currentspeed falls short of meeting intraoperative real-time requirements, itmaintains high efficiency in preoperative planning and postoperativeevaluation without significantly increasing the overall diagnostic timecost. Future study on quantitative comparison of plaque distribution isworthy of investigation based on further improvement of CTA modelplaque detection performance.
Notably, our framework is not limited to any specific image contentand modalities, we believe it could serve as a universal frameworkfor fusion tasks across various 3D vascular-like structures, such asbronchial tube, gastrointestinal tract, renal artery, cerebral vessel, etc.,thereby is spotential to expand into broader clinical application scenarios. However, it should be noted that several hyperparameters in thisstudy are specifically tailored to the characteristics of coronary data,such as the number of sampling points and network layers, which maynot be universally applicable when transferring to other tasks.
本文提出了AutoFOX,这是一种完全自动化的冠状动脉X线造影(XA)与光学相干断层成像(OCT)跨模态三维融合框架,通过初始重建(Initial Reconstruction)、配准(Co-Registration)和融合重建(Fusion Reconstruction)三个步骤实现。AutoFOX通过专门设计的多任务模型TransCAN克服了现有血管三维对准和融合方法的局限性。AutoFOX将血管轮廓视为序列数据,采用三维XA直化去除冗余曲率,并通过3D到1D的转换减少模型参数。此外,重建算法针对侧支腔进行了优化,从而增强了分叉病变的评估能力。
与其他方法相比,TransCAN表现出最高的对准精度。TransCAN的创新点在于深度融合了侧支(SB)信息:SB-Matching子任务增强了侧支特征的匹配;BPEG模块提供了侧支加权的相对位置编码;SPSP注意力在降低跨注意力计算复杂度的同时,仍能有效维持侧支信息的交互。提出了两种TransCAN模式:nTransCAN和softTransCAN。前者在整体序列水平上实现了最佳精度,而后者在临床关键位置上表现更佳,并在更大数据集上具有更好的扩展性。所提出的CSA损失有效提升了模型的鲁棒性。尽管某些模块仅带来了较小的数值改进,但考虑到其在小病变长度和侧支开口长度中的比例,这些改进仍具有重要的临床意义。
最终,我们通过5种形态学指标使用独立的多中心数据集,并以配对的CTA作为参考标准,对融合模型进行了评估。在CTA和AutoFOX生成的融合模型之间观察到高度的形态学一致性,尤其是在临床显著的BOA(分支开口角度)和BMLA(分支主血管腔面积)方面。此外,AutoFOX融合模型在腔体绝对测量中的较小差异及其与CTA的相关性进一步证明了OCT的集成增强了融合模型与实际腔体结构的一致性。
本研究的一个主要优势是整个框架的完全自动化,无需人工干预。这得益于AutoFOX内部专门设计的模块,使其对上游输出的潜在噪声具有较强的鲁棒性。最后,先进的三维融合模型在指导CAD患者的经皮冠状动脉介入治疗中具有重要的应用价值,特别是在复杂分叉病变中。通过增强病变可视化,该技术使心脏病学家能够在手术过程中做出更明智的决策,优化治疗策略,并可能减少并发症。
基于成像的计算生理学评估支持证据的积累,OCT与XA的融合可通过AutoFOX提供的精确几何建模,增强对关键参数(如分数流储备FFR和内皮剪切应力ESS)的评估精度。此外,融合后的三维冠状动脉树也为研究CAD发展的血流动力学机制铺平了道路,可能揭示疾病进展的新见解,并为更有针对性的治疗干预提供指导。关于AutoFOX的分析速度,尽管目前的速度尚未达到术中实时需求,但在术前规划和术后评估中仍保持了较高的效率,并未显著增加整体诊断时间成本。未来基于进一步提高CTA模型斑块检测性能,开展斑块分布定量比较的研究值得探索。
值得注意的是,AutoFOX框架并不限于特定的图像内容和模态,我们相信它可以作为一种通用框架,适用于各种三维血管状结构的融合任务,如支气管、胃肠道、肾动脉、脑血管等,因此具有扩展到更广泛临床应用场景的潜力。然而,需要指出的是,本研究中的若干超参数是针对冠状动脉数据的特性量身定制的,例如采样点的数量和网络层数,这些参数可能在转移到其他任务时不具有普遍适用性。
Results
结果
The performance of AutoFOX is evaluated both internally for alignment accuracy of TransCAN and externally for morphological accuracyof the fusion model, using data from real world clinical practice withno overlap between them.
• To develop and evaluate TransCAN for vessel alignment, pairedXA and OCT images of 278 patients from core lab (CardHemo,Med-X Research Institute, Shanghai Jiao Tong University) wereused. Experienced analysts at the core lab performed the dataannotation using the AngioPlus Core software (version V3, PulseMedical, Shanghai, China), generating 55,104 alignment pairs.The dataset was split into training, validation, and test sets in aratio of 7:1:2 at vessel level. We followed the standard practiceby using training data to learn model parameters, tuning hyperparameters and selecting the model based on validation data. Testdata is resrved solely for evaluating model once it is finalized.Model performance and ablation studies were reported on the testdata.
• For the external validation of AutoFOX fusion model’s morphological accuracy, we used an independent dataset of 67 patientswith coronary CTA, XA, and OCT images. The data were providedby two sites: OLV Clinic, Aalst, Belgium (site1, 50 patients),and Fujian Medical University Union Hospital, Fuzhou, China(site2, 16 patients). The ethic committees of these two hospitalsapproved the retrospective analysis of these datasets. Patientsprovided written informed consent. The CTA model is used asthe reference standard for morphology assessment, which is automatically generated by CtaPlus Core software (version V2, PulseMedical, Shanghai, China).The time intervals between different image modalities acquisitionwere within 3 months in majority (86.57%) of the study population,and the rest were less than 6 months. All images were acquired priorto any coronary intervention. Additionally, the analyzed CTA andXA images were synchronized to either end-diastole or end-systole toensure temporal synchrony.
AutoFOX的性能通过内部和外部评估进行验证,分别针对TransCAN的对准精度和融合模型的形态学精度。所用数据均来源于真实临床实践,且两者之间无数据重叠。
用于开发和评估TransCAN血管对准模型的数据 来自核心实验室(CardHemo,上海交通大学Med-X研究院)的278名患者的配对XA和OCT图像。核心实验室的经验分析师使用AngioPlus Core软件(版本V3,Pulse Medical,上海,中国)对数据进行了标注,生成了55,104个对准配对。 数据集按血管级别以7:1:2的比例分为训练集、验证集和测试集。遵循标准实践,使用训练数据学习模型参数,并根据验证数据调整超参数和选择模型。测试数据仅用于最终模型的性能评估。一旦模型定型,我们仅对测试数据进行评估,并报告模型性能和消融实验结果。
用于AutoFOX融合模型形态学精度的外部验证 我们使用了一组独立的数据集,包括67名患者的冠状动脉CTA、XA和OCT图像。这些数据来自两个机构:比利时奥尔夫诊所(OLV Clinic, Aalst,比利时,50名患者)和中国福建医科大学附属协和医院(Fujian Medical University Union Hospital, Fuzhou, China,16名患者)。两家医院的伦理委员会批准了对这些数据集的回顾性分析,并获得了患者的书面知情同意。 CTA模型作为形态学评估的参考标准,由CtaPlus Core软件(版本V2,Pulse Medical,上海,中国)自动生成。
在研究人群中,大多数患者(86.57%)不同图像模态的采集时间间隔在3个月以内,其余患者的时间间隔不超过6个月。所有图像均在任何冠状动脉介入治疗之前获取。此外,分析的CTA和XA图像均同步至舒张末期或收缩末期,以确保时间同步性。
Figure
图
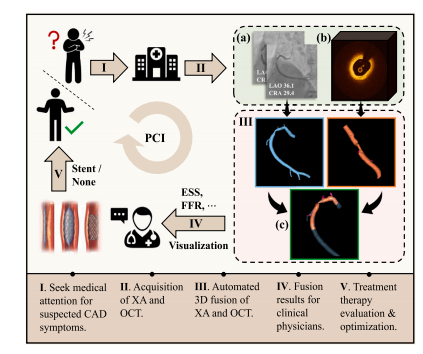
Fig. 1. Coronary artery disease diagnosis and treatment guided by the 3D fusion ofXA and OCT. (a) XA images from two views and (b) OCT pullback, and © 3D fusionmodel of coronary tree
图1. 基于XA和OCT三维融合的冠状动脉疾病诊断与治疗指导。(a) 两个视角的XA图像,(b) OCT回拉图像,© 冠状动脉树的三维融合模型。
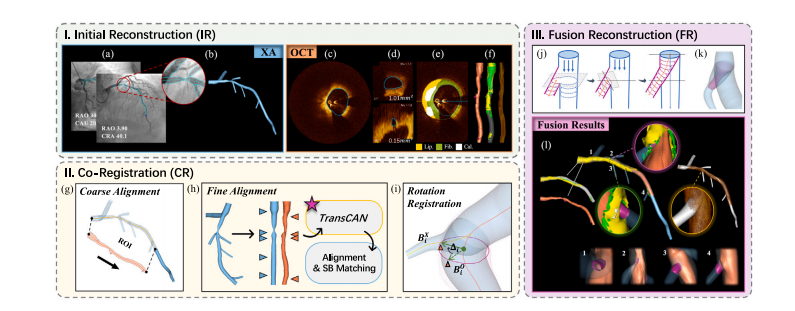
Fig. 2. Graphical illustration of the experimental setup for each individual gastrointestinal endoscopic application. For each set of experiments, the section numbers are indicated in which the experimental results are presented and discussed.
图2. 每个胃肠道内镜应用的实验设置图示。对于每组实验,标注了实验结果展示和讨论所在的章节编号。
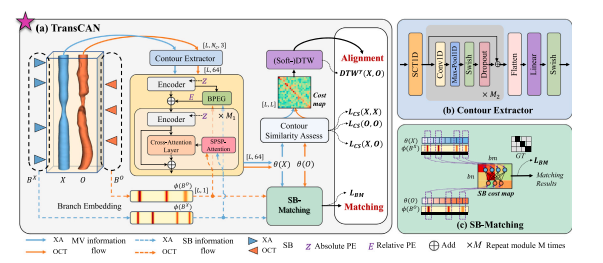
Fig. 3. The schematic of the design of (a) TransCAN and the details of (b) Contour Extractor module and © SB-Matching module in TransCAN.
图3. (a) TransCAN设计示意图,以及(b) 轮廓提取模块和© TransCAN中侧支匹配模块的详细结构。
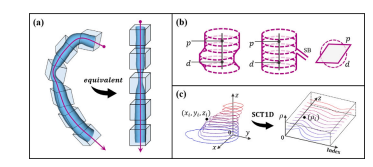
Fig. 4. Visual description of key challenges in fine-alignment. (a) The straighteningof 3D-XA with cubes representing convolution block; (b) Example slices p and d havesame area but differ in anatomical and topological characteristics. From left to rightrepresent the two slices have spatial distortions, locating on opposite directions ofa SB, and different contour shapes; © The SCT1D transforms 3D points into a 1Drepresentation.
图4. 精细对准中的关键挑战的可视化描述。(a) 三维XA的直化处理,其中方块代表卷积块;(b) 示例切片p和d具有相同的面积,但在解剖学和拓扑特性上存在差异。从左到右分别表示两个切片存在空间扭曲、位于侧支的相反方向以及轮廓形状不同;© SCT1D将三维点转换为一维表示。
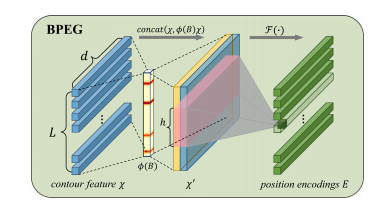
Fig. 5. Branch Position Encoder Generator (BPEG) module. 𝐿 and 𝑑 represent thelength of sequence and contour feature 𝜒, respectively. The (⋅) consists of a flatteningoperation followed by a 1D-Depthwise Separable Convolution with a kernel size of ℎ×1.
图5. 分支位置编码生成器(BPEG)模块。𝐿 和 𝑑 分别表示序列长度和轮廓特征 𝜒。(⋅) 包括一个展平操作,随后进行核大小为 ℎ×1 的一维深度可分卷积。
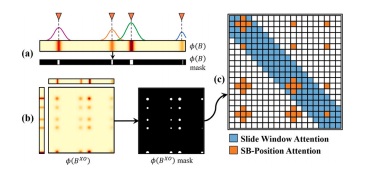
Fig. 6. Illustration of Branch Embedding and SPSP-Attention. (a) Branch Embedding𝜙(𝐵) with its binarized mask; (b) SB-position attention using 𝜙(𝐵 XO) with its binarizedmask; © SPSP-attention mask combines Slide Window attention and SB-Positionattention.
图6. 分支嵌入和SPSP注意力的示意图。(a) 分支嵌入 𝜙(𝐵) 及其二值化掩膜;(b) 使用 𝜙(𝐵 XO) 进行的侧支位置注意力及其二值化掩膜;© SPSP注意力掩膜结合了滑动窗口注意力和侧支位置注意力。
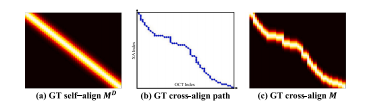
Fig. 7. Alignment matrices. (a) The GT self-align matrix 𝑀𝐷; (b) The GT cross-align path generated from annotation; © The GT cross-align matrix ?
图7. 对齐矩阵。(a) GT自对齐矩阵 𝑀𝐷;(b) 基于标注生成的GT跨模态对齐路径;© GT跨模态对齐矩阵 ?。
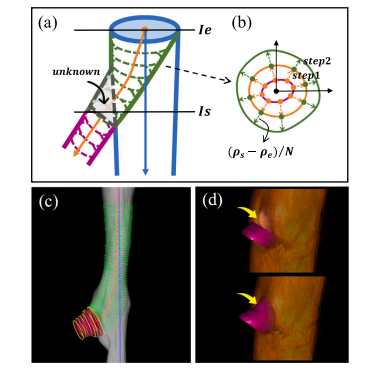
Fig. 8. Process of side branch lumen reconstruction. (a) Interpolation of OCT SB lumencontours; (b) Gradual growth of contours during the interpolation ; © Interpolationeffect of the new OCT SB lumen; (d) Repair and completion at the SB ostium afterreconstruction.
图8. 侧支腔重建过程。(a) OCT侧支腔轮廓的插值;(b) 插值过程中轮廓的逐步生长;© 新的OCT侧支腔插值效果;(d) 重建后在侧支开口处的修复与完善。
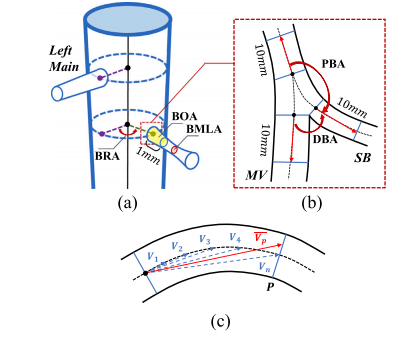
Fig. 9. Diagram of morphological metrics. (a) Definitions of BRA, BOA and BMLA; (b)Definitions of PBA and DBA; © Definition of directional vector ̄𝑉𝑝 pointing towards𝑃 .
图9. 形态学指标示意图。(a) BRA(分支角度)、BOA(分支开口角度)和BMLA(分支主血管腔面积)的定义;(b) PBA(分叉血管角度)和DBA(远端血管角度)的定义;© 指向 𝑃 的方向向量 ̄ 𝑉𝑝 的定义。
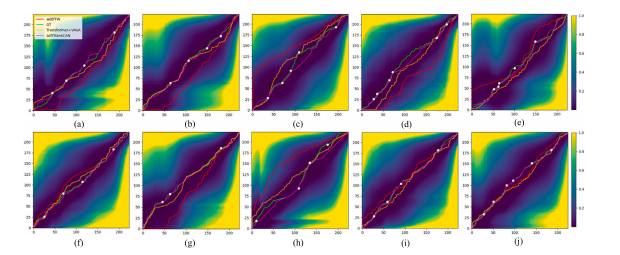
Fig. 10. Alignment results display of different methods. (a) to (j) are the alignment results of ten samples from the validation set. The background in the images shows the costpredicted by softTransCAN. The dynamic paths are from wdDTW, Transformer+VAVA, softTransCAN and GT. The paths are generated according to their respective cost maps. Thewhite dots represent key anatomical positions
图10. 不同方法的对准结果展示。(a) 至 (j) 是验证集中十个样本的对准结果。图像背景显示了softTransCAN预测的成本分布。动态路径分别来自wdDTW、Transformer+VAVA、softTransCAN和GT。这些路径是根据各自的成本图生成的。白点表示关键解剖位置。
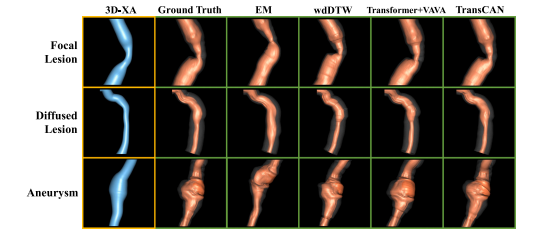
Fig. 11. The impact of different alignment methods on the fusion model for focal lesion, diffused lesion and aneurysm.
图11. 不同对准方法对融合模型在局灶性病变、弥漫性病变和动脉瘤中的影响。
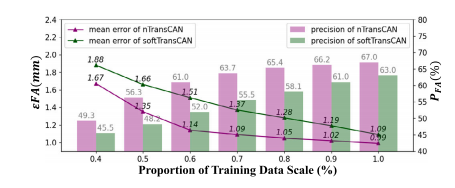
Fig. 12. The learning curve of nTransCAN and softTransCAN with changes in trainingdata scale.
图12. nTransCAN和softTransCAN在训练数据规模变化下的学习曲线。
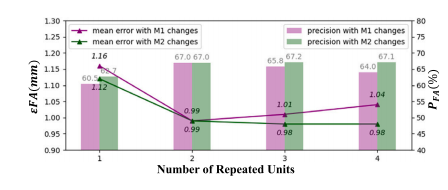
Fig. 13. The accuracy of nTransCAN with changes of the unit repetition number 𝑀1and 𝑀2 .
图13. nTransCAN在单位重复次数 𝑀1 和 𝑀2 变化下的准确性。
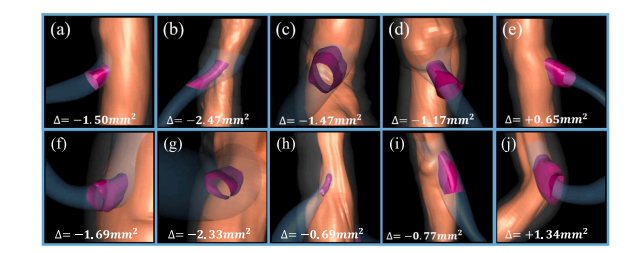
Fig. 14. Visualization of side branch ostia generated by AutoFOX (purple) overlapped with side branch of 3D-XA (transparent blue), and 𝛥 is the BOA difference between AutoFOXand 3D-XA.
图14. AutoFOX生成的侧支开口(紫色)与三维XA的侧支(透明蓝色)的可视化重叠,𝛥 表示AutoFOX与三维XA之间的BOA差异。
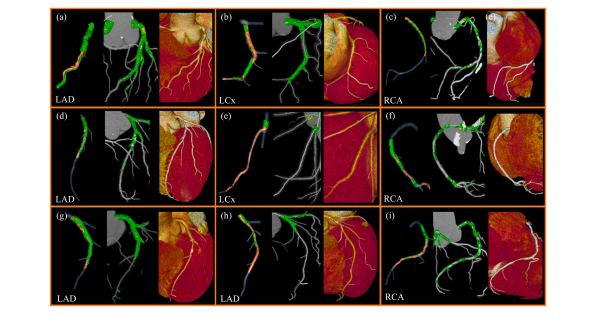
Fig. 15. The reconstructed fusion model by the AutoFOX. (a) to (i) are paired comparison between the fusion model (left), the reference CTA vessel tree model (middle) and thewhole heart CTA image (right). There is high consistency in structure and intraluminal information distribution between the AutoFOX model and the CTA model in 3D space.Orange indicates OCT lumen and green indicates fibrous plaques.
图15. AutoFOX重建的融合模型。(a) 至 (i) 为融合模型(左)、参考CTA血管树模型(中)和全心CTA图像(右)的配对对比。AutoFOX模型与CTA模型在三维空间中的结构和腔内信息分布具有高度一致性。橙色表示OCT腔体,绿色表示纤维斑块。
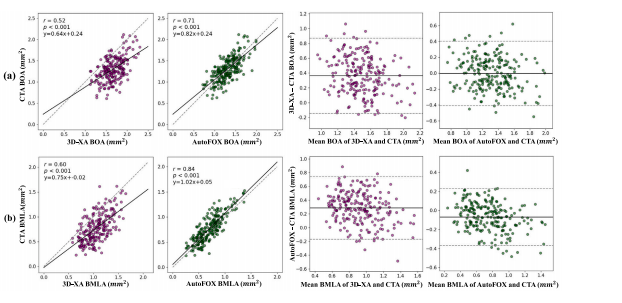
Fig. 16. Correlation and agreement between 3D-XA and CTA-model in (a) BOA and (b) BMLA.
图16. 三维XA与CTA模型在(a) BOA和(b) BMLA上的相关性和一致性。
Table
表
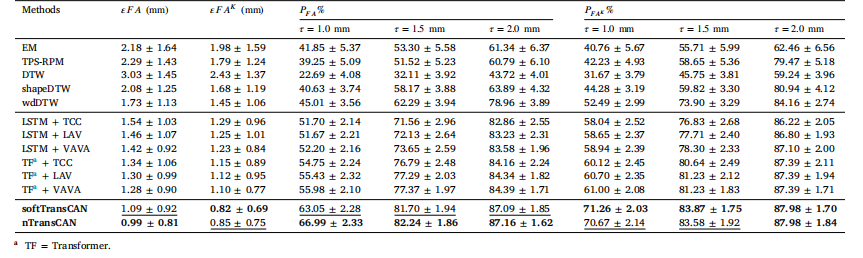
Table 1Alignment results of TransCAN and comparison methods.
表1 TransCAN及对比方法的对准结果。

Table 2Performance of ablation study on TransCAN.
表2TransCAN消融实验的性能表现。
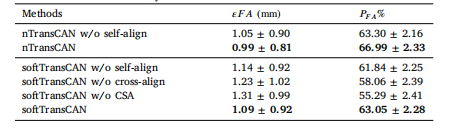
Table 3Performance of ablation study on CSA loss.
表3关于CSA损失的消融研究性能结果。
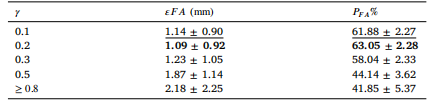
Table 4Performance of smooth parameter in SoftTransCAN.
表4SoftTransCAN中平滑参数的性能表现。

Table 5Morphologicalresults of AutoFOX
表5AutoFOX的形态学结果。
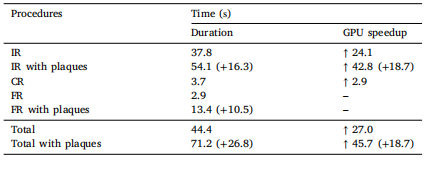
Table 6Time cost of AutoFOX.
表6AutoFOX的时间成本。