Title
题目
Will Transformers change gastrointestinal endoscopic image analysis? Acomparative analysis between CNNs and Transformers, in terms of performance, robustness and generalization
Transformer会改变胃肠道内镜图像分析吗?基于性能、鲁棒性和泛化能力的卷积神经网络(CNN)与Transformer的比较分析
01
文献速递介绍
基于深度学习的人工智能(Artificial Intelligence, AI)技术已广泛应用于计算机视觉(Computer Vision, CV)的各个领域,包括医学图像分析。在过去十年中,AI驱动的技术在该领域迅速流行,并展示了最先进的性能(Zhou等,2020)。卷积神经网络(Convolutional Neural Networks, CNNs)在这一成功中发挥了重要作用,在分类(He等,2016)、目标检测(Redmon等,2016)和语义分割(Ronneberger等,2015)等任务中表现出色。最近,在计算机视觉领域,视觉Transformer(Vision Transformer, ViT)(Dosovitskiy等,2021)架构出现,它采用了最初为自然语言处理设计的Transformer(Vaswani等,2017)架构。ViT及其衍生模型(以下简称为Transformers)迅速在通用计算机视觉问题中占据了一席之地,并在性能上与CNNs展开竞争。Transformer架构的自注意力机制是其独特特性之一,能够更好地理解图像的上下文信息,为其与CNNs的竞争提供了优势。然而,Transformer是否也有潜力在医学图像分析领域带来革命性变化尚不清楚(Matsoukas等,2021;Li等,2023;Parvaiz等,2023;Shamshad等,2023;Azad等,2024)。
胃肠道内镜图像分析是医学领域一个快速发展的分支,为基于深度学习的计算机辅助检测和诊断(CADe/CADx)工具提供了广阔的应用机会。内镜设备图像质量的提高使得内镜图像的视觉检查成为胃肠道内镜的重要组成部分。内镜技术的使用为胃肠道疾病(如癌症、溃疡和炎症性疾病)的无创诊断和治疗提供了可能。然而,由于正常组织和异常组织之间的微小差异,以及内镜医师间一致性较低,内镜图像分析面临着显著的挑战。因此,已经开发出多种CADe和CADx技术,以帮助内镜医师诊断和治疗胃肠道并发症。
尽管如此,内镜图像质量(Image Quality, IQ)可能受到运动/失焦模糊、过度/不足曝光等伪影的显著影响,这些伪影通常由内镜的操作不当引起。此外,内镜图像外观(Image Appearance, IA)取决于内镜采集设备,并受后期处理和压缩步骤的影响。一些研究表明,深度学习技术的性能依赖于图像的质量和外观,即使是轻微的图像降质也可能导致性能的显著下降(Hendrycks和Dietterich, 2019;Pei等,2021)。
Aastract
摘要
Gastrointestinal endoscopic image analysis presents significant challenges, such as considerable variations inquality due to the challenging in-body imaging environment, the often-subtle nature of abnormalities with lowinterobserver agreement, and the need for real-time processing. These challenges pose strong requirementson the performance, generalization, robustness and complexity of deep learning-based techniques in suchsafety–critical applications. While Convolutional Neural Networks (CNNs) have been the go-to architecturefor endoscopic image analysis, recent successes of the Transformer architecture in computer vision raise thepossibility to update this conclusion. To this end, we evaluate and compare clinically relevant performance,generalization and robustness of state-of-the-art CNNs and Transformers for neoplasia detection in Barrett’sesophagus. We have trained and validated several top-performing CNNs and Transformers on a total of10,208 images (2,079 patients), and tested on a total of 7,118 images (998 patients) across multiple testsets, including a high-quality test set, two internal and two external generalization test sets, and a robustnesstest set. Furthermore, to expand the scope of the study, we have conducted the performance and robustnesscomparisons for colonic polyp segmentation (Kvasir-SEG) and angiodysplasia detection (Giana). The resultsobtained for featured models across a wide range of training set sizes demonstrate that Transformersachieve comparable performance as CNNs on various applications, show comparable or slightly improvedgeneralization capabilities and offer equally strong resilience and robustness against common image corruptionsand perturbations. These findings confirm the viability of the Transformer architecture, particularly suitedto the dynamic nature of endoscopic video analysis, characterized by fluctuating image quality, appearanceand equipment configurations in transition from hospital to hospital.
胃肠道内镜图像分析面临许多重大挑战,例如由于体内成像环境复杂导致的图像质量差异显著、病变往往较为隐匿且观察者间一致性较低,以及对实时处理的需求。这些挑战对基于深度学习的技术在性能、泛化能力、鲁棒性和复杂性方面提出了较高要求,因为这是一个安全性至关重要的应用领域。尽管卷积神经网络(Convolutional Neural Networks, CNNs)一直是内镜图像分析的首选架构,但近年来Transformer架构在计算机视觉领域的成功为这一结论的更新提供了可能性。
为此,我们评估并比较了最先进的CNN和Transformer在Barrett食管瘤变检测中的临床相关性能、泛化能力和鲁棒性。我们在总计10,208张图像(来自2,079名患者)上训练和验证了几种表现突出的CNN和Transformer模型,并在多个测试集上进行了测试,这些测试集包括一个高质量测试集、两个内部泛化测试集、两个外部泛化测试集以及一个鲁棒性测试集。此外,为了扩展研究范围,我们还在结肠息肉分割(Kvasir-SEG)和血管发育不良检测(Giana)任务上进行了性能和鲁棒性对比。
研究结果表明,在各种训练集规模下,Transformer在多个应用中的性能与CNN相当,在泛化能力方面表现出相当或略有提升,并且在面对常见图像损坏和扰动时表现出同样强的鲁棒性。这些发现证实了Transformer架构的可行性,尤其适用于内镜视频分析的动态特性,该特性包括图像质量、外观和设备配置在不同医院间的波动变化。
Method
方法
This study aims to offer valuable insights into the comparative efficacy of CNNs and Transformers for gastrointestinal endoscopic imageanalysis, in terms of the required performance, data efficiency, robustness and generalization capabilities. Several methods and materials areconsidered and described in the upcoming subsections. Firstly, Section 3.1 provides a detailed description of the datasets. Subsequently,in Section 3.2, we discuss the data pre-processing techniques, followedby the introduction of the employed network architectures, while anexplanation of the rationale for selection is addressed in Section 3.3.Lastly, the training details and performance evaluation are discussedin Sections 3.4 and 3.5, respectively.
本研究旨在从性能要求、数据效率、鲁棒性和泛化能力等方面,为胃肠道内镜图像分析中CNN(卷积神经网络)和Transformer的比较效果提供有价值的见解。研究中考虑并描述了多种方法和材料,具体内容将在后续小节中详述。首先,第3.1节详细介绍了所使用的数据集。随后,第3.2节讨论了数据预处理技术,第3.3节介绍了所采用的网络架构,并解释了选择这些架构的依据。最后,第3.4节和第3.5节分别讨论了训练细节和性能评估方法。
Conclusion
结论
A. Motivation: The rapid progress of machine learning solutions hasresulted in the emergence of the Vision Transformer (ViT) and itsderived instances fitting to the field of Computer Vision (CV), whichhave challenged the state-of-the-art Convolutional Neural Networks(CNNs) in various CV tasks. However, the potential of Transformersin medical image analysis, and specifically, gastrointestinal endoscopicimage analysis, is not yet clear. Endoscopic image analysis is an important field within medical image analysis, where deep learning-basedComputer-Aided Detection and Diagnosis (CADe/CADx) tools can playa significant role. Nevertheless, endoscopic image analysis poses severalchallenges due to subtle differences between normal and abnormaltissue, low-interobserver agreement among endoscopists and imagequality and image appearance fluctuations. These challenges and fluctuations have a proven and significant impact on the performanceof such deep learning-based techniques, posing requirements on theperformance, robustness and generalization capabilities. Therefore, theefficacy of deep learning-based CADe/CADx systems in safety–criticalapplications, including gastrointestinal cancer screening, depends ontheir performance, robustness and generalization capabilities, whilethey also offer real-time processing speed. This arises the inquiryabout the suitability of Transformers for the purpose of gastrointestinalendoscopic image analysis, compared to the state-of-the-art CNNs.
A. 研究动机:机器学习解决方案的快速发展催生了视觉Transformer(Vision Transformer, ViT)及其衍生模型在计算机视觉(CV)领域的应用,并在多个CV任务中对最先进的卷积神经网络(Convolutional Neural Networks, CNNs)发起了挑战。然而,Transformer在医学图像分析,特别是胃肠道内镜图像分析中的潜力尚不清楚。
内镜图像分析是医学图像分析中的一个重要领域,基于深度学习的计算机辅助检测和诊断(CADe/CADx)工具在该领域中可以发挥重要作用。然而,由于正常组织与异常组织之间的微小差异、内镜医师之间低一致性、以及图像质量和图像外观的波动,内镜图像分析面临诸多挑战。这些挑战和波动已被证明对深度学习技术的性能具有显著影响,对性能、鲁棒性和泛化能力提出了更高要求。因此,在包括胃肠道癌症筛查在内的安全性至关重要的应用中,深度学习CADe/CADx系统的效果取决于其性能、鲁棒性和泛化能力,同时还需提供实时处理速度。这引发了关于Transformer是否适合用于胃肠道内镜图像分析,与最先进的CNN相比其表现如何的探讨。
Figure
图
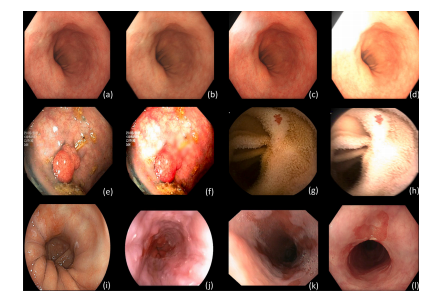
Fig. 1. Variational samples of the different test sets. (a) Barrett’s Test: high-quality subtle neoplasia case; (b) Barrett’s Test-C: corrupted hue and motion blur; © Barrett’s Test-C:overexposure and corrupted saturation; (d) Barrett’s Test-C: overexposure, motion blur, corrupted hue and brightness; (e) Kvasir-SEG: colonic polyp; (f) Kvasir-SEG-C: defocus blur,corrupted contrast, hue and brightness; (g) Giana: angiodysplasia case; (h) Giana-C: motion-blur, overexposure, corrupted saturation, brightness and hue; (i) ARGOS: high-qualitysubtle neoplasia case of different scope manufacturer; (j) BORN: low-quality image of different scope manufacturer; (k) QRT: low-quality image (blur and presence of bubbles); (l)CRT: NDBE case with visible abnormality
图1. 不同测试集的变异样本。 (a) Barrett’s Test:高质量的细微瘤变病例; (b) Barrett’s Test-C:色调损坏和运动模糊; © Barrett’s Test-C:过度曝光和饱和度损坏; (d) Barrett’s Test-C:过度曝光、运动模糊、色调和亮度损坏; (e) Kvasir-SEG:结肠息肉; (f) Kvasir-SEG-C:失焦模糊、对比度、色调和亮度损坏; (g) Giana:血管发育不良病例; (h) Giana-C:运动模糊、过度曝光、饱和度、亮度和色调损坏; (i) ARGOS:不同内镜制造商的高质量细微瘤变病例; (j) BORN:不同内镜制造商的低质量图像; (k) QRT:低质量图像(模糊且有气泡); (l) CRT:带有可见异常的非肠上皮化生(NDBE)病例。
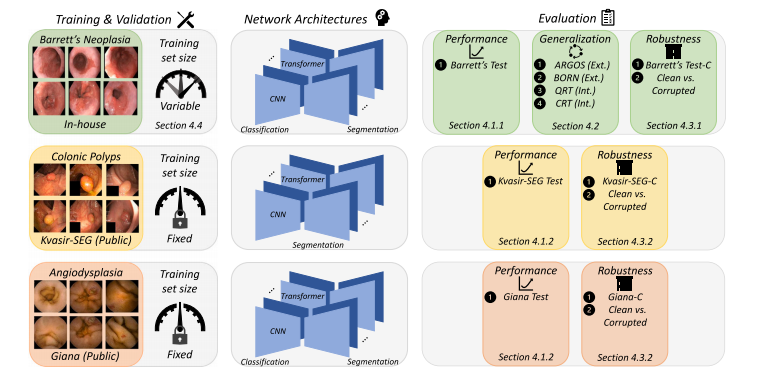
Fig. 2. Graphical illustration of the experimental setup for each individual gastrointestinal endoscopic application. For each set of experiments, the section numbers are indicated in which the experimental results are presented and discussed.
图2. 每个胃肠道内镜应用的实验设置图示。对于每组实验,标注了实验结果展示和讨论所在的章节编号。
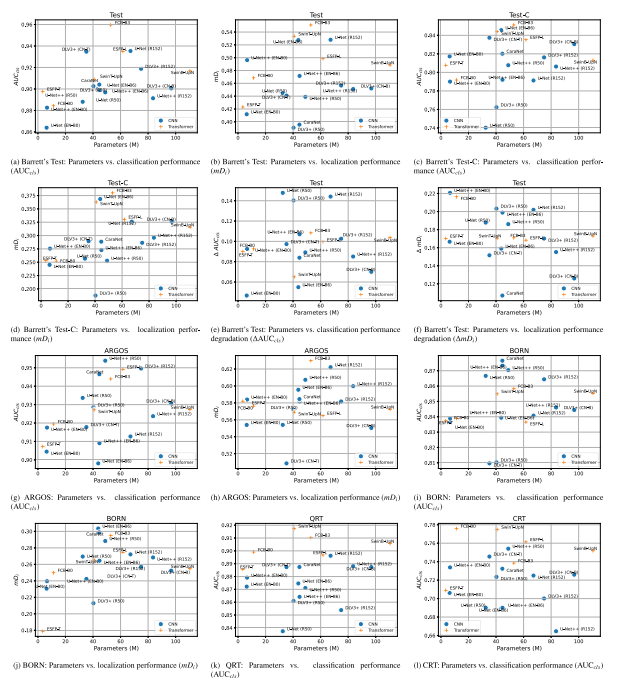
Fig. 3. Scatter plots illustrate the mean bootstrap classification performance (AUC𝑐𝑙𝑠 ) or the mean bootstrap localization performance (𝑚𝐷**𝑖 ) values together with the number ofparameters on the Barrett’s Test, ARGOS, BORN, QRT, CRT, and the corrupted Barrett’s Test-C sets. Furthermore, scatter plots depict the mean bootstrap performance degradation(𝛥𝑚𝐷**𝑖 and 𝛥AUC𝑐𝑙𝑠 ) between the clean and corrupted version of the respective set. The use of four different datasets indicates the generalization capabilities of the networkarchitectures, and the use of a corrupted dataset provides a clear view on the robustness of the network architectures.
图3. 散点图展示了Barrett’s测试集、ARGOS、BORN、QRT、CRT以及损坏的Barrett’s Test-C数据集上的平均自举分类性能(AUCcls)或平均自举定位性能(mDi)值与参数数量之间的关系。此外,散点图还显示了对应数据集中干净版本与损坏版本之间性能下降(ΔmDi 和 ΔAUCcls*)的平均自举值。通过使用四个不同数据集,展示了网络架构的泛化能力;而损坏数据集的使用则提供了对网络架构鲁棒性的一种清晰观察。
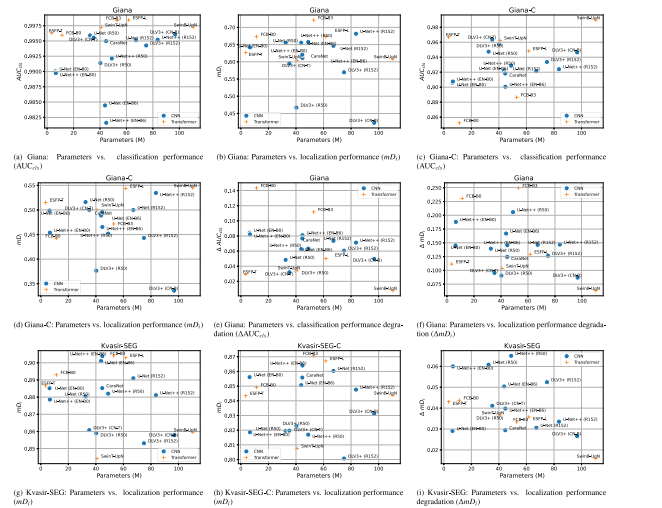
Fig. 4. Scatter plots illustrate the mean bootstrap classification performance (AUC𝑐𝑙𝑠 ) or the mean bootstrap localization performance (𝑚𝐷𝑖 ) values together with the number ofparameters on the publicly available Giana and Kvasir-SEG datasets, and the corrupted Giana-C and Kvasir-SEG-C sets. Furthermore, scatter plots depict the mean performancebootstrap degradation (𝛥𝑚𝐷**𝑖 and 𝛥AUC𝑐𝑙𝑠 ) between the clean and corrupted version of respective sets. The use of corrupted datasets provides a clear view on the robustness ofvarious network architectures.
图4. 散点图展示了公共的Giana和Kvasir-SEG数据集,以及损坏的Giana-C和Kvasir-SEG-C数据集上的平均自举分类性能(AUCcls)或平均自举定位性能(mDi)值与参数数量之间的关系。此外,散点图还显示了对应数据集中干净版本与损坏版本之间性能下降(ΔmDi 和 ΔAUCcls)的平均自举值。损坏数据集的使用清晰展示了不同网络架构的鲁棒性。
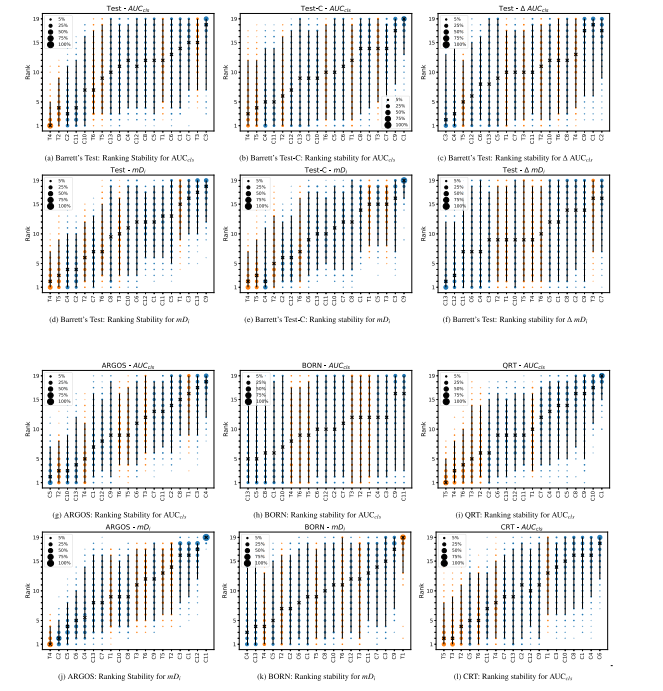
Fig. 5. Blob plots illustrating the ranking stability based on bootstrap sampling (1000 samples) for classification performance (AUC𝑐𝑙𝑠 ) and localization performance (𝑚𝐷**𝑖 ) on theBarrett’s Test, ARGOS, BORN, QRT, CRT and corrupted Barrett’s Test-C sets as well as the performance degradation between the clean and corrupted version of the Barrett’s Testset (𝛥 AUC𝑐𝑙𝑠/𝛥 𝑚𝐷**𝑖 ). CNNs and Transformers are color coded and code names, specified in Table 3, are used for each specific network architecture. The area of each blob isproportional to the relative frequency the architecture achieved that specific rank. The median rank for each architecture is indicated by a black cross. 95% bootstrap intervalsacross bootstrap samples (ranging from 2.5th to the 97.5th percentile of the bootstrap distribution) are indicated by black lines. It should be noted that the models are orderedfrom left to right, with the best model at the left side and the worst at the right, determined by the mean-rank score across bootstrap samples. A lower rank on the y-axis indicatesbetter performance.
图5. 使用自举采样(1000次样本)计算的分类性能(AUCcls)和定位性能(mDi)在Barrett’s测试集、ARGOS、BORN、QRT、CRT以及损坏的Barrett’s Test-C数据集上的排名稳定性Blob图,同时展示了Barrett’s测试集干净版本与损坏版本之间性能下降(ΔAUCcls/ΔmDi)的排名稳定性。CNN和Transformer通过颜色编码区分,特定网络架构的代码名称使用表3中的标注。每个Blob的面积与架构达到特定排名的相对频率成比例。每种架构的中位排名用黑色叉标出,自举样本的95%置信区间(从2.5百分位到97.5百分位的自举分布范围)用黑色线条表示。需要注意的是,模型从左到右排序,左侧为最佳模型,右侧为最差模型,排序依据自举样本的平均排名分数。y*-轴上的排名越低,表示性能越好。
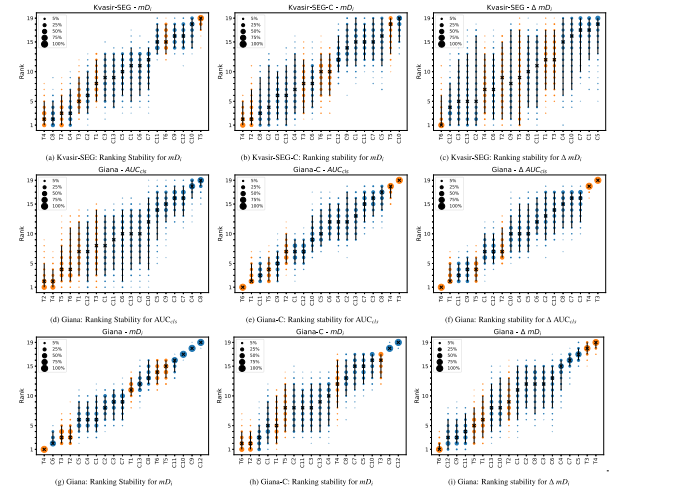
Fig. 6. Blob plots illustrating the ranking stability based on bootstrap sampling (1000 samples) for classification performance (AUC𝑐𝑙𝑠 ) and localization performance (𝑚𝐷𝑖 ) on theKvasir-SEG, Giana and corrupted Kvasir-SEG-C and Giana-C sets as well as the performance degradation between the clean and corrupted version of the respective sets (𝛥 AUC𝑐𝑙𝑠/𝛥𝑚𝐷*𝑖 ). CNNs and Transformers are color coded and code names, specified in Table 3, are used for each specific network architecture. The area of each blob is proportional to therelative frequency the architecture achieved that specific rank. The median rank for each architecture is indicated by a black cross. 95% bootstrap intervals across bootstrap samples(ranging from 2.5th to the 97.5th percentile of the bootstrap distribution) are indicated by black lines. It should be noted that the models are ordered from left to right, with thebest model at the left side and the worst at the right, determined by the mean-rank score across bootstrap samples. A lower rank on the y-axis indicates better performance
图6. 使用自举采样(1000次样本)计算的分类性能(AUCcls)和定位性能(mDi)在Kvasir-SEG、Giana以及损坏的Kvasir-SEG-C和Giana-C数据集上的排名稳定性Blob图,同时展示了各数据集中干净版本与损坏版本之间性能下降(ΔAUCcls/ΔmDi)的排名稳定性。CNN和Transformer通过颜色编码区分,特定网络架构的代码名称使用表3中的标注。每个Blob的面积与架构达到特定排名的相对频率成比例。每种架构的中位排名用黑色叉标出,自举样本的95%置信区间(从2.5百分位到97.5百分位的自举分布范围)用黑色线条表示。需要注意的是,模型从左到右排序,左侧为最佳模型,右侧为最差模型,排序依据自举样本的平均排名分数。y*-轴上的排名越低,表示性能越好。
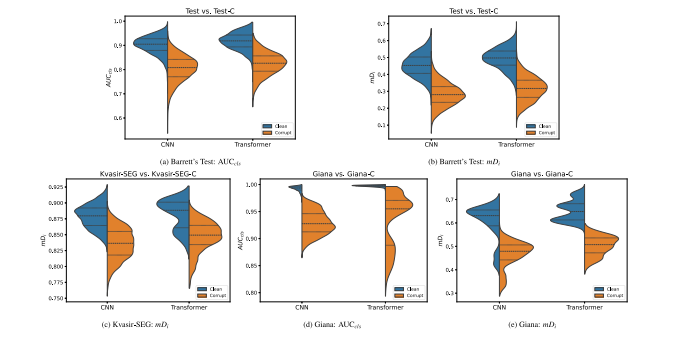
Fig. 7. Violin plots indicating the distribution based on 1000 bootstrap samples of AUC𝑐𝑙𝑠 and/or 𝑚𝐷𝑖 for both the CNN and Transformer architecture types, on the Barrett’s Test,Kvasir-SEG, Giana, corrupt Barrett’s Test-C, corrupt Kvasir-SEG-C and corrupt Giana-C sets. Each half of a violin visualizes the distribution of the respective performance metricvalues and is supplemented with the mean and inter-quartile range
图7. 小提琴图展示了基于1000次自举样本的AUCcls和/或mDi分布,用于CNN和Transformer两种架构类型,在Barrett’s测试集、Kvasir-SEG、Giana、损坏的Barrett’s Test-C、损坏的Kvasir-SEG-C和损坏的Giana-C数据集上的性能表现。每个小提琴图的一半表示相应性能指标值的分布,并补充显示了均值和四分位间距。
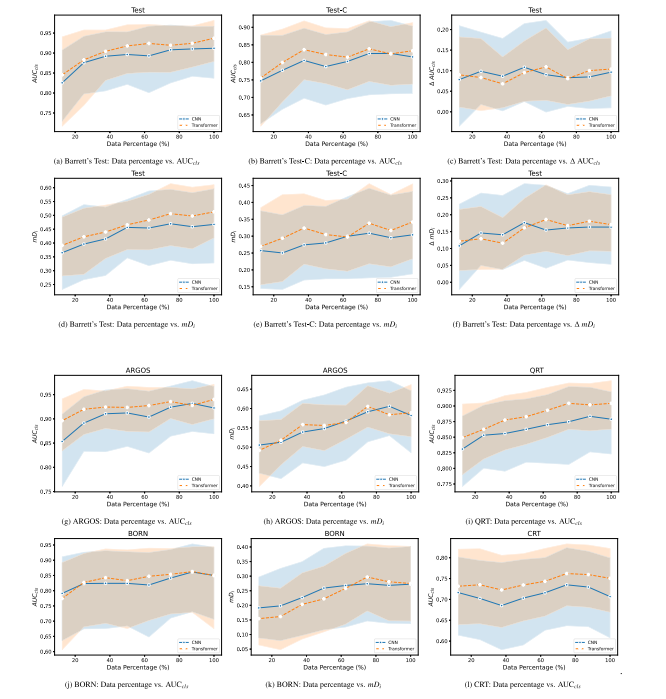
Fig. 8. Line plots indicating the mean bootstrap AUC𝑐𝑙𝑠 and/or 𝑚𝐷𝑖 values on the ARGOS, BORN, QRT, CRT, Barrett’s Test and corrupted Barrett’s Test-C sets. Plots are a functionof the data percentage of the total Barrett’s training set size. Furthermore, line plots are presented with the mean bootstrap performance degradation (𝛥 𝑚𝐷𝑖 and 𝛥 AUC𝑐𝑙𝑠 ) betweenthe clean and corrupted version of the Barrett’s Test Set. The line plots are supplemented with error bands indicating the 95%CI of the bootstrap samples.
图8. 折线图展示了基于自举样本的平均AUCcls和/或mDi值在ARGOS、BORN、QRT、CRT、Barrett’s测试集及损坏的Barrett’s Test-C数据集上的表现。图表以Barrett’s训练集总数据量百分比为函数。此外,还展示了Barrett’s测试集干净版本与损坏版本之间性能下降(ΔmDi 和 ΔAUCcls*)的折线图。折线图补充了表示自举样本95%置信区间的误差带。
Table
表
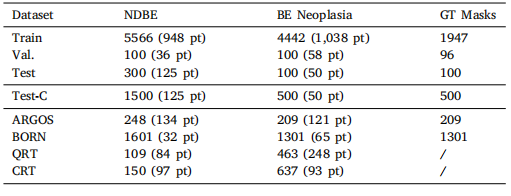
Table 1Description of datasets used for algorithm development and evaluation for the Barrett’sneoplasia detection task. Number of images with corresponding number of patients forthe algorithm development sets, Barrett’s Test Set (Test), corrupted Barrett’s Test-C Set(Test-C) and the Generalization test sets
表1 Barrett瘤变检测任务中用于算法开发和评估的数据集描述。包括算法开发数据集、Barrett’s测试集(Test)、损坏的Barrett’s测试集(Test-C)以及泛化测试集的图像数量及对应患者数量的详细信息。

Table 2Amount of images and GT masks, subdivided over each split, for the public Kvasir-SEG and Giana datasets and correspondingcorrupted sets
表2 公共Kvasir-SEG和Giana数据集及其对应的损坏数据集在每个划分中的图像和GT(Ground Truth)掩膜数量。
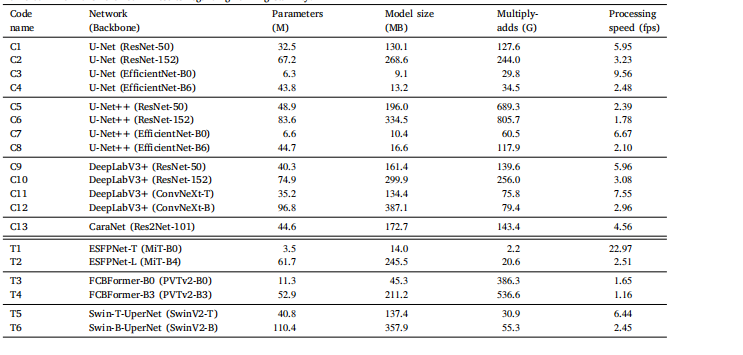
Table 3Complexity comparison of used architectures, in terms of parameters, model size, multiply-add operations and processing speed (measured ona 12-core Ryzen 9 5900X CPU, displayed value is the average of 5 runs of each 500 forward passes). Each network is associated with a codename to which it is referred in results regarding ranking stability.
表3 所用架构的复杂性比较,包括参数数量、模型大小、乘加运算次数以及处理速度(在12核Ryzen 9 5900X CPU上测量,显示的值为每个网络进行500次前向传播的平均值,共运行5次)。每个网络均分配了一个代号,用于结果中排名稳定性的相关讨论。
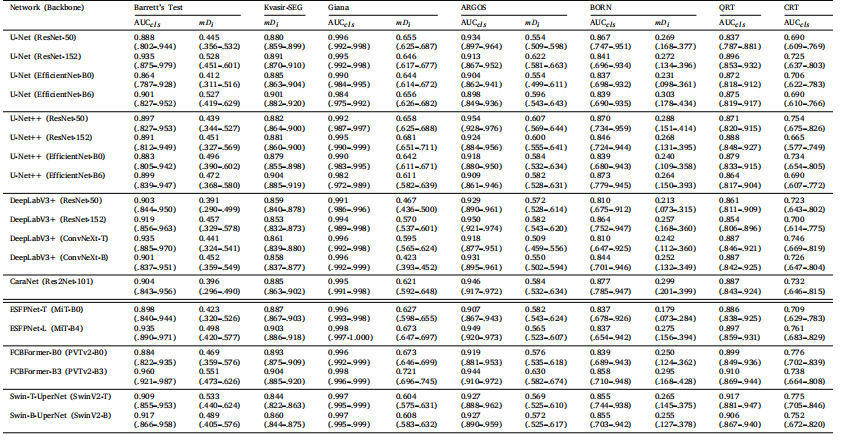
Table 4Performance on the Barrett’s Test Set, Kvasir-SEG test set and Giana test set. Generalization performance on ARGOS, BORN, QRT and CRT sets. Results are presented as Mean(95% CI), resulting from 1000 bootstrap samples.
表4 Barrett’s测试集、Kvasir-SEG测试集和Giana测试集上的性能表现,以及在ARGOS、BORN、QRT和CRT测试集上的泛化性能。结果以均值(95%置信区间)表示,基于1000次自举样本计算得出。
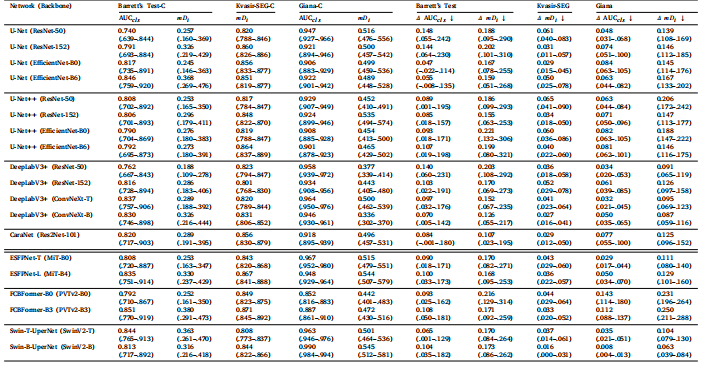
Table 5Robustness performance on the corrupted Barrett’s Test-C Set, Kvasir-SEG-C test set and Giana-C test set, and the robustness performance degradation between clean and corruptedversions of respective sets. Results are presented as Mean (95% CI), resulting from 1000 bootstrap samples
表5在损坏的Barrett’s Test-C测试集、Kvasir-SEG-C测试集和Giana-C测试集上的鲁棒性性能,以及各数据集干净版本与损坏版本之间的鲁棒性性能下降。结果以均值(95%置信区间)表示,基于1000次自举样本计算得出。