Title
题目
UM-Net: Rethinking ICGNet for polyp segmentation with uncertainty modeling
UM-Net:基于不确定性建模的息肉分割方法,对ICGNet的重新思考
01
文献速递介绍
结直肠癌(CRC)是男性中第三大最常见的恶性肿瘤,女性中第二大最常见的恶性肿瘤,2020年全球病例数接近100万例,占全球癌症死亡总数的9.4%(Sung等人,(2021). 约85%的结直肠癌是由结直肠息肉发展而来的,尤其是高危息肉(Strum和Williamson,2016)。幸运的是,如果能够及时发现并切除结直肠息肉,就可以有效降低结直肠癌的发病率和死亡率,5年生存率可提高至90%(Siegel等人,
(2021). 在临床实践中,高质量的结肠镜检查是筛查和去除结直肠息肉的最佳方法,可被视为金标准,可提供息肉的位置和外观。然而,该程序通常由内窥镜医生手动操作,可能会受到人为主观因素的影响,由于息肉的多样性,可能会出现漏诊。结肠镜检查中息肉的漏诊率高达21.4%(金等人,2017年)。因此,迫切需要一种自动、可靠的息肉分割方法,以协助医生在诊断过程中定位息肉区域。随着结直肠息肉在不同发育阶段结构和特征的变化(贾等人,2021年),息肉的大小、形状不规则、颜色和外观各异,这使得对其进行分析具有挑战性。此外,一些挑战可能导致分割过程中出现错误,例如存在水流、肠道内容物、模糊、气泡和亮度变化等图像伪影(吴等人,2021年)。近年来,随着医学图像分析中深度学习技术的发展,一系列息肉分割方法取得了显著的成功(周等人,2018年;Ronneberger等人,2015年;方等人,2019年;钟等人,2020年)。虽然这些方法极大地提高了分割精度,但它们仍然受到多发性息肉的类内不一致性、低对比度和息肉颜色不一致等因素的影响。因此,如何设计一种模型来解决这些问题并实现高性能的息肉分割仍然是一个极具挑战性的课题。如图1(a)中的a1所示,我们的初步工作ICGNet(Du等人,2022)通过使用反轮廓信息引导学习和局部-全局上下文信息自适应学习来解决低对比度和类内不一致性问题。然而,ICGNet的两个固有局限性给其带来了巨大的瓶颈:(1)忽视了不一致的息肉颜色。在手术场景中,由于设备、位置和息肉的性质等因素,收集到的息肉具有不一致的颜色分布(如图1(b)中的b1所示)。对于息肉分割,如果不去除这种不一致的色彩效果,就会导致色彩过拟合,干扰模型的训练。实际上,这些不一致的色彩特征远不如结构特征重要,但模型在提取特征时会同时关注无关紧要的色彩和有用的形状,并将它们结合起来。因此,有必要让模型更加关注目标结构和形状。(2)分割结果的可靠性不足。在临床实践中,临床医生试图确保他们希望做出的决策能够产生有利的结果。因此,一个值得信赖的分割模型不仅应该能够给出高精度的预测,还应该提供不确定性,以便医生能够做出更好的判断,并了解应该对结果信任多少来做出明智的决定。ICGNet的表现非常好,但它只给出预测结果,而不告诉我们结果的确定性如何(见图1-(a)中的a1,黄色的反向钩表示低不确定性,结果准确可靠,而黄色的问号表示高不确定性,结果不准确)。
Abatract
摘要
Automatic segmentation of polyps from colonoscopy images plays a critical role in the early diagnosis and treatment of colorectal cancer. Nevertheless, some bottlenecks still exist. In our previous work, we mainly focused on polyps with intra-class inconsistency and low contrast, using ICGNet to solve them. Due to the different equipment, specific locations and properties of polyps, the color distribution of the collected images is inconsistent. ICGNet was designed primarily with reverse-contour guide information and local–global context information, ignoring this inconsistent color distribution, which leads to overfitting problems and makes it difficult to focus only on beneficial image content. In addition, a trustworthy segmentation model should not only produce high-precision results but also provide a measure of uncertainty to accompany its predictions so that physicians can make informed decisions. However, ICGNet only gives the segmentation result and lacks the uncertainty measure. To cope with these novel bottlenecks, we further extend the original ICGNet to a comprehensive and effective network (UM-Net) with two main contributions that have been proved by experiments to have substantial practical value. Firstly, we employ a color transfer operation to weaken the relationship between color and polyps, making the model more concerned with the shape of the polyps. Secondly, we provide the uncertainty to represent the reliability of the segmentation results and use variance to rectify uncertainty. Our improved method is evaluated on five polyp datasets, which shows competitive results compared to other advanced methods in both learning ability and generalization capability.
从结肠镜图像中自动分割息肉在结直肠癌的早期诊断和治疗中起着关键作用。然而,仍然存在一些瓶颈问题。在我们之前的研究中,我们主要通过ICGNet解决了息肉类别内不一致性和低对比度的问题。然而,由于设备的不同以及息肉的特定位置和特性,采集图像的颜色分布往往不一致。ICGNet主要通过反向轮廓引导信息和局部-全局上下文信息设计而成,但忽略了这种颜色分布的不一致性,这导致了过拟合问题,使得模型难以仅关注有益的图像内容。
此外,一个可信的分割模型不仅需要生成高精度的结果,还需要为其预测结果提供不确定性评估,以便医生能够据此做出明智的决策。然而,ICGNet仅提供了分割结果,而缺乏不确定性评估。为了解决这些新出现的瓶颈问题,我们进一步扩展了原有的ICGNet,提出了一个全面且有效的网络(UM-Net),通过实验证明了其在实际应用中的重要价值。
我们的主要贡献有两点:我们引入了一种颜色迁移操作,以削弱颜色与息肉之间的关联,使模型更关注息肉的形状特征。
我们提供了分割结果的不确定性评估,并使用方差对不确定性进行修正。
改进后的方法在五个息肉数据集上进行了评估,与其他先进方法相比,在学习能力和泛化能力方面表现出了竞争力。
Method
方法
3.1. Problem definitionLet ?? = {( ???? , ????)}?? ??=1 represent the ?? labeled set, where each pair ( ???? , ????) consists of an image ???? ∈ R??×??×?? and its corresponding ground truth ???? ∈ {0, 1} ??×?? , where ?? × ?? are spatial dimensions and?? is the number of channels. As discussed in the introduction, the aim is to train a segmentation network ???????? under solving the polyp color and uncertainty problem to obtain good performance on the test data. In this work, given two inputs ????1 and ????2 , the color ?? of ????2 is transferred to ????1 to get the new input ????1 , which constitute the segmentation network ????????( ????1) . We also model uncertainty in the prediction results???? ??????( ????????( ????1)) , with ?? ∈ [0, 4], while minimizing the prediction bias?? ???? ( ????????( ????1) , ?? 1) .
设数据集 D={(xi,yi)}i=1N\mathcal{D} = {(x_i, y_i)}{i=1}N 表示包含 NN 个标注样本的集合,其中每对 (xi,yi)(x_i, y_i) 包括一幅图像 xi∈RH×W×Cx_i \in \mathbb{R}^{H \times W \times C} 和其对应的真实标签 yi∈{0,1}H×Wy_i \in {0, 1}^{H \times W}。这里,H×WH \times W 是空间维度,CC 是通道数。如在引言中讨论的,本研究的目标是通过解决息肉颜色和不确定性问题,训练一个分割网络 Fseg\mathcal{F}{seg},以在测试数据上取得良好的性能。在本研究中,给定两个输入图像 x1x_1 和 x2x_2,将 x2x_2 的颜色 cc 转移到 x1x_1 上,生成新的输入图像 x1\hat{x}1,并利用其作为分割网络 Fseg(x1)\mathcal{F}{seg}(\hat{x}1) 的输入。此外,我们对预测结果 U(Fseg(x1))\mathcal{U}(\mathcal{F}{seg}(\hat{x}1)) 建立不确定性模型,其中 U\mathcal{U} 的范围为 0,4。同时,目标是最小化预测偏差 Lseg(Fseg(x1),y1)\mathcal{L}{seg}(\mathcal{F}{seg}(\hat{x}1), y_1)。
Conclusion
结论
In this paper, we propose a novel automatic segmentation framework, namely UM-Net, to comprehensively solve the challenging tasks of polyp segmentation from colonoscopic images. The proposed UMNet firstly deals with the inconsistent polyp color by color transfer operation in the input part of the network. Then in the network feature extraction part, the RCG module effectively addresses low contrast and missed diagnosis, and the ALGM module help identifies various scales of the polyp. Finally, we provide uncertainty for the segmentation masks to represent the reliability of the results. Extensive experimentson five polyp segmentation datasets demonstrate the superiority of the proposed method compared with state-of-the-art approaches in both learning ability and generalization capability. Furthermore, our proposed approach is a generic technique that can be flexibly extended to other medical image segmentation tasks where blurry boundary, diverse scales and inconsistent image color are the main challenges.
在本文中,我们提出了一种新颖的自动分割框架——UM-Net,用于全面解决从结肠镜图像中分割息肉这一具有挑战性的任务。UM-Net首先通过网络输入部分的颜色迁移操作处理息肉颜色不一致的问题。然后,在网络特征提取部分,RCG模块有效解决了低对比度和漏诊的问题,ALGM模块则有助于识别不同尺度的息肉。最后,我们为分割掩模提供了不确定性评估,以表示结果的可靠性。在五个息肉分割数据集上的大量实验表明,所提出的方法在学习能力和泛化能力方面均优于现有的最先进方法。此外,我们提出的方法是一种通用技术,可以灵活扩展到其他医学图像分割任务,尤其是在边界模糊、尺度多样和图像颜色不一致是主要挑战的情况下。
Results
结果
In this section, we perform the learning ability of our approach on two datasets, and the quantitative results are shown in Tables 2and 3. Compared with ICGNet, UM-Net has improved the Dice and mIoU metrics from 87.93%, 89.56% to 89.26%, and 90.33% respectively on the EndoScene dataset, and from 92.35%, 91.99% to 93.04%, and 92.54% respectively on the Kvasir-SEG dataset. Similarly, ourmethod is superior to other advanced approaches and achieves the best performance, further demonstrating good model learning ability. In addition, we also conduct the complexity analysis comparing our method with other advanced methods. The indicators we compare include floating point operations (FLOPs), network parameters (Params), and frames per second (FPS). On the EndoScene dataset, the FLOPs, Params, and FPS of the UM-Net are 16.87G, 22.75M, and 46 respectively, meanwhile achieving 15.62G, 22.75M, and 50 on the Kvasir-SEG dataset. Although Polyp-PVT obtains the minimum value in FLOPs, our method only increases 8.28G, and 7.66G on the two datasets, respectively. In terms of Params, our model has fewer network parameters than most advanced methods. Since the accuracy of polypsegmentation is crucial for physicians to produce accurate diagnostic results, we pay more attention to the accuracy of segmentation with little difference in model computational complexity. Therefore, UM-Net is still considered to be the optimal model with reasonable efficiency. It is worth noting that the inference speed of our model can reach an average of 48 FPS, which can be used as an auxiliary system for diagnosis to satisfy real-time prediction.
在本节中,我们对两组数据集进行了评估以验证我们方法的学习能力,量化结果如表 2 和表 3 所示。与ICGNet相比,UM-Net在EndoScene数据集上的Dice和mIoU指标分别从87.93%、89.56%提高到89.26%、90.33%;在Kvasir-SEG数据集上,这两个指标分别从92.35%、91.99%提高到93.04%、92.54%。同样,我们的方法在其他先进方法中表现出更优越的性能,进一步证明了模型优秀的学习能力。此外,我们还进行了复杂性分析,将我们的方法与其他先进方法进行了比较。对比指标包括浮点运算量(FLOPs)、网络参数数量(Params)和每秒帧数(FPS)。在EndoScene数据集上,UM-Net的FLOPs为16.87G,Params为22.75M,FPS为46;而在Kvasir-SEG数据集上,FLOPs为15.62G,Params为22.75M,FPS为50。尽管Polyp-PVT在FLOPs上取得了最低值,我们的方法仅在这两个数据集上分别增加了8.28G和7.66G。在Params方面,我们的模型网络参数比大多数先进方法更少。
由于息肉分割的精确性对于医生提供准确的诊断结果至关重要,我们更关注分割的准确性,而对模型计算复杂度的轻微差异影响较少。因此,UM-Net仍然被认为是具有合理效率的最优模型。值得注意的是,我们的模型推理速度平均可达48 FPS,可作为诊断辅助系统,满足实时预测需求。
Figure
图
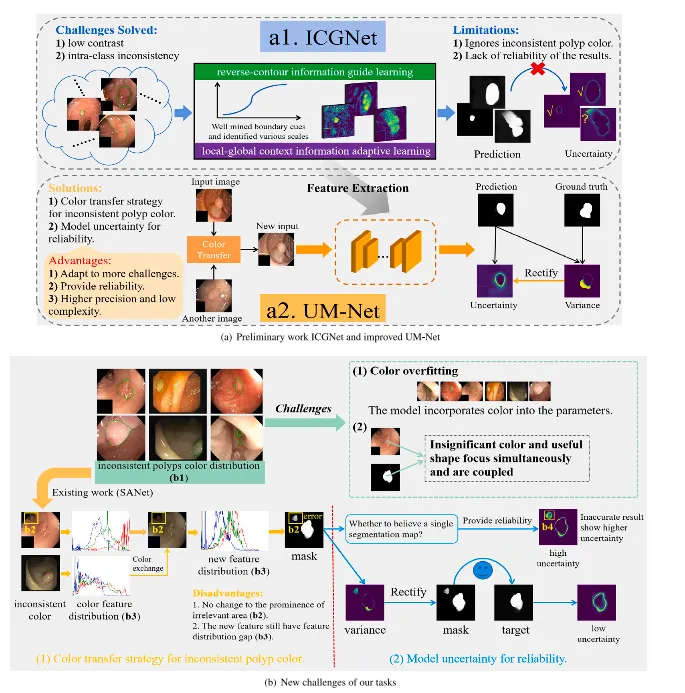
Fig. 1. Challenges and method of our framework to handle the polyps segmentation via using the colonoscopy images. From (a) to (b), they are preliminary work ICGNet and improved method UM-Net, the new challenges of our tasks, respectively.
图 1. 我们框架在处理基于结肠镜图像的息肉分割任务中的挑战与方法。从(a)到(b),分别展示了前期工作ICGNet、改进方法UM-Net,以及任务中面临的新挑战。
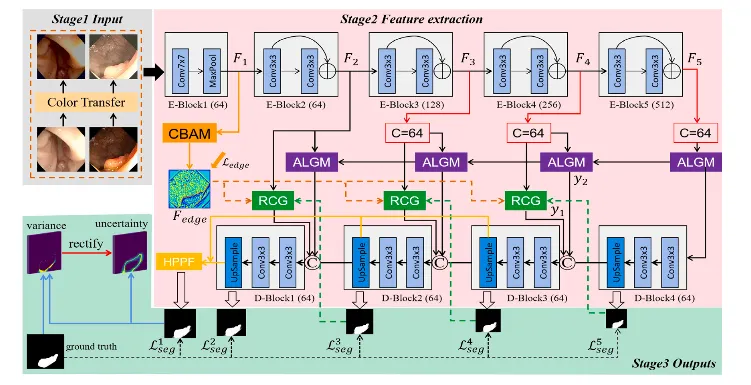
Fig. 2. Overview of the improved UM-Net. It segments the polyps and consists of three stages. Stage1 Input: By using the new polyp images after the color transfer operation as input. Stage2 Feature extraction. Stage3 Outputs: Output segmentation mask as well as corresponding uncertainty. Specifically, the RCG, ALGM, and HPPF modules refer to ICGNet (Du et al., 2022).
图 2. 改进的UM-Net概述。该网络用于分割息肉,共包括三个阶段。
阶段1 输入:以经过颜色迁移操作后的新息肉图像作为输入。 阶段2 特征提取。 阶段3 输出:生成分割掩模以及对应的不确定性输出。
具体而言,RCG、ALGM和HPPF模块参考了ICGNet (Du等, 2022)。
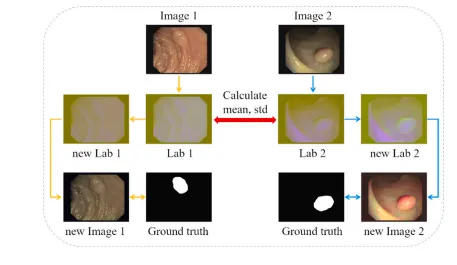
Fig. 3. One iteration of the color transfer operation.
图 3. 一次颜色迁移操作的迭代过程。
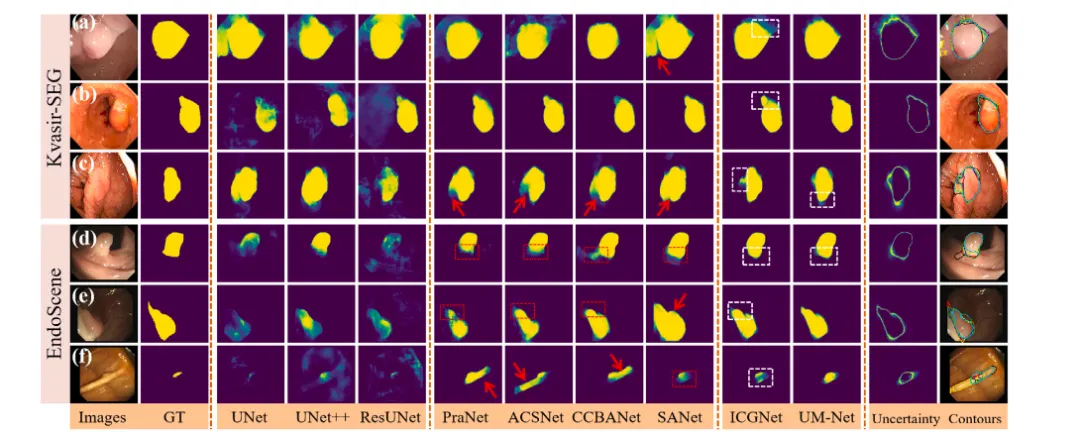
Fig. 4. Qualitative results of different methods on Kvasir-SEG and EndoScene datasets. The segmentation results are converted to contours and shown in the last column (ground truth in red, PraNet in cyan, ACSNet in yellow, CCBANet in black, SANet in white, ICGNet in blue, UM-Net in green). In addition, the red dashed boxes indicate the missed diagnosis area, the red arrows indicate areas that are larger than the ground truth, and the white dashed boxes show the difference between ICGNet and UM-Net predictions.
图 4. 不同方法在Kvasir-SEG和EndoScene数据集上的定性结果。分割结果被转换为轮廓并显示在最后一列(真实值为红色,PraNet为青色,ACSNet为黄色,CCBANet为黑色,SANet为白色,ICGNet为蓝色,UM-Net为绿色)。此外,红色虚线框表示漏诊区域,红色箭头表示分割区域大于真实值的区域,白色虚线框显示ICGNet和UM-Net预测结果之间的差异。
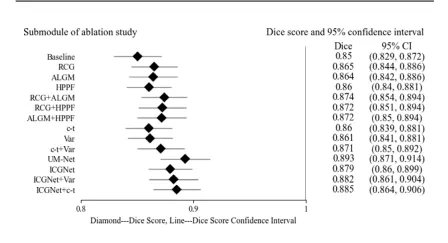
Fig. 5. Forest plot of ablation study on the EndoScene test set. Listed on the left side are the submodules of the ablation study. On the right side are the submodules corresponding Dice scores and 95% confidence intervals, and in the middle are their visual results, where diamond represents the Dice score of each submodule, and the horizontal line connecting the diamond represents the upper and lower limits of the score confidence interval.
图 5. EndoScene测试集上消融研究的森林图。左侧列出了消融研究的子模块,右侧显示了子模块对应的Dice分数及其95%置信区间,中间为其可视化结果。图中菱形表示每个子模块的Dice分数,连接菱形的水平线表示分数置信区间的上下限。
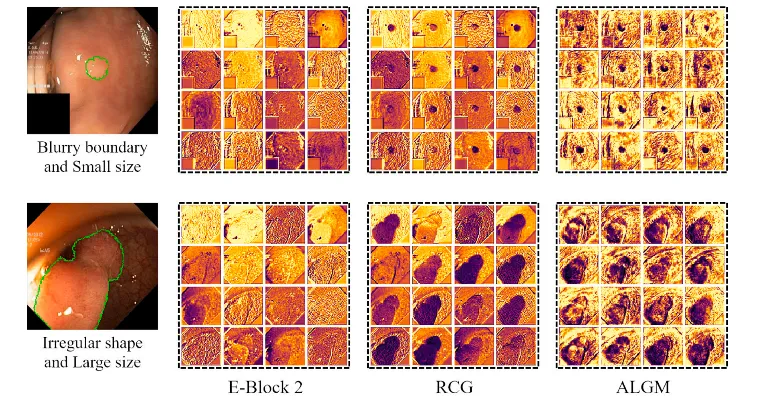
Fig. 6. Feature visualization examples of the UM-Net’s second layer. From left to right are input images (the green curve represents the outline of ground truth), the E-Block 2 feature, the RCG module feature, and the ALGM module feature, respectively. After applying two modules, the network well captured missing object parts and details near the boundary, and achieved feature representation.
图 6. UM-Net第二层特征可视化示例。从左到右分别为输入图像(绿色曲线表示真实值的轮廓)、E-Block 2特征、RCG模块特征和ALGM模块特征。通过应用这两个模块,网络能够很好地捕捉到缺失的目标部分以及边界附近的细节,从而实现了良好的特征表示。
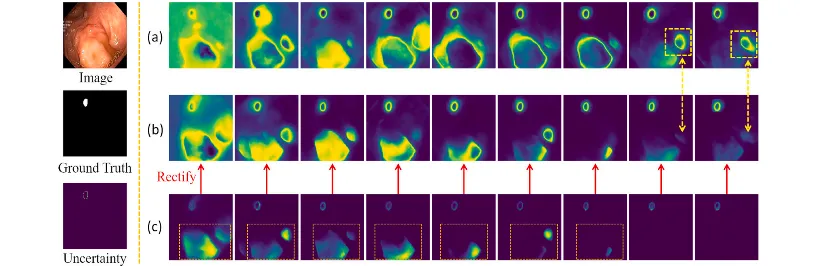
Fig. 7. Shows the variation of UM-Net modeling uncertainty as the number of training iterations continues to increase. From top to bottom on the left are the input images, the ground truth, and the corresponding uncertainty. Row (a) denotes the uncertainty output without variance rectification. Row (b) denotes the uncertainty results of variance rectification. Row © denotes the variance calculated between the prediction masks and the ground truth.
图 7. 展示了随着训练迭代次数的增加,UM-Net对不确定性建模的变化情况。左侧从上到下依次为输入图像、真实值以及对应的不确定性。
(a) 行表示未经过方差修正的不确定性输出结果。 (b) 行表示经过方差修正的不确定性结果。 © 行表示预测掩模与真实值之间计算的方差。
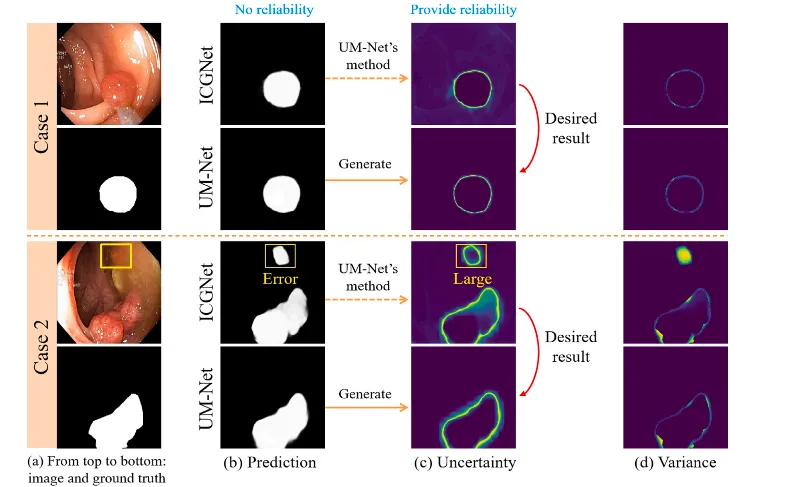
Fig. 8. Provide an evaluation of the reliability degree of the result of two cases in the test set. For each case, from left to right, the first column is the input image and its corresponding ground truth. The second column displays the prediction for the ICGNet and UM-Net. The third column displays the uncertainty map associated with the prediction for both models. The last column displays the variance.
图 8. 对测试集中两个案例的结果可靠性进行评估。对于每个案例,从左到右:第一列为输入图像及其对应的真实值;第二列显示ICGNet和UM-Net的预测结果;第三列显示两个模型预测结果相关的不确定性图;最后一列显示方差。
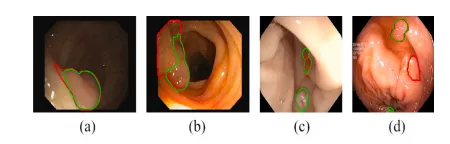
Fig. 9. Failure cases in EndoScene (a, b) and Kvasir-SEG (c, d) datasets. Green and red contours outline our prediction and ground truth of the polyp boundary.
图 9. EndoScene数据集(a, b)和Kvasir-SEG数据集(c, d)中的失败案例。绿色轮廓表示我们的预测结果,红色轮廓表示息肉边界的真实值。
Table
表
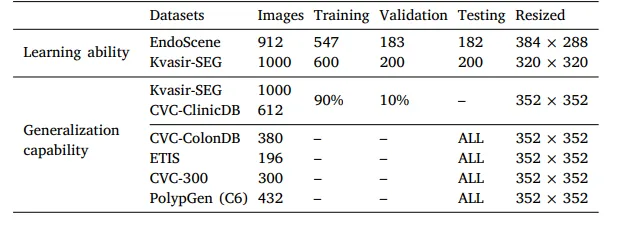
Table 1Details of the datasets for training, validation and testing.
表 1用于训练、验证和测试的数据集详细信息。
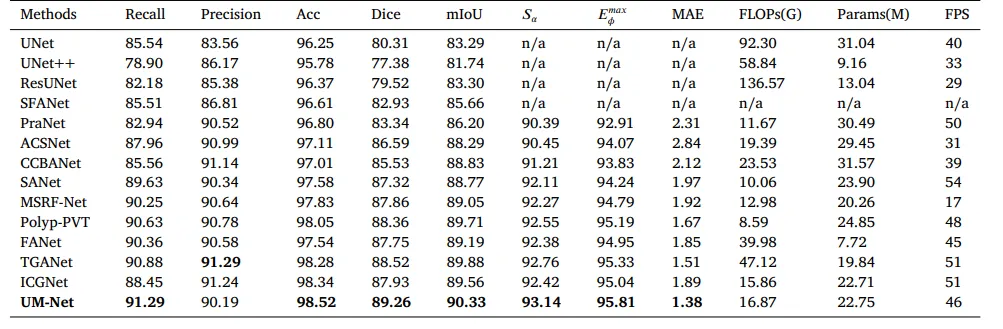
Table 2Quantitative results of the EndoScene test datasets. ‘n/a’ denotes that the results are not available.
表 2EndoScene测试数据集的量化结果。“n/a”表示结果不可用。
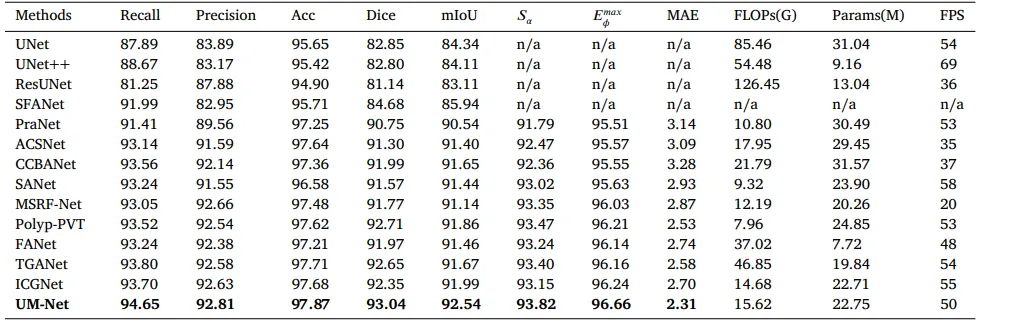
Table 3Quantitative results of the Kvasir-SEG test datasets. ‘n/a’ denotes that the results are not available.
表 3Kvasir-SEG测试数据集的量化结果。“n/a”表示结果不可用。
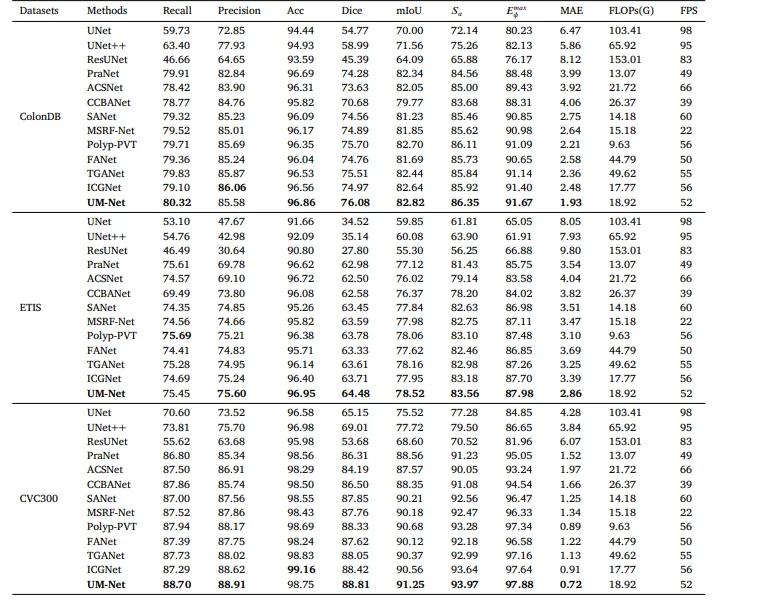
Table 4Quantitative results of the test datasets ColonDB, ETIS and CVC300.
表 4测试数据集ColonDB、ETIS和CVC300的量化结果。
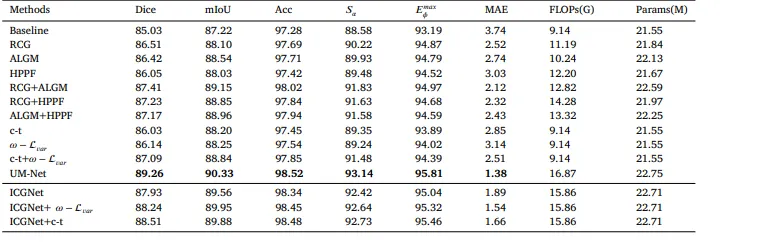
Table 5The quantitative evaluation of the ablation studies on the EndoScene test set.
表 5EndoScene测试集上消融研究的量化评估结果。
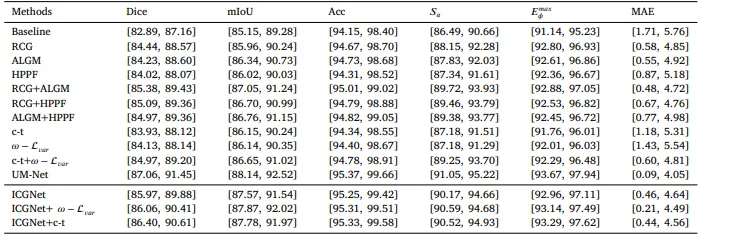
Table 695% confidence intervals for all metrics.
表 6所有指标的95%置信区间。
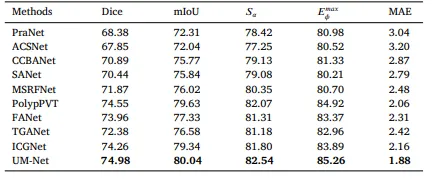
Table 7Quantitative results for a subset (C6) of the PolypGen dataset.
表 7PolypGen 数据集子集 (C6) 的量化结果。
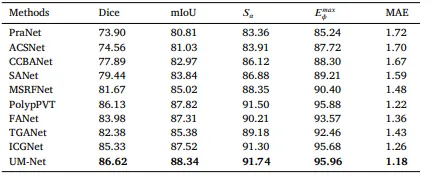
Table 8Quantitative results on CVC-300-TV dataset.
表 8CVC-300-TV数据集的量化结果。