Title
题目
Semi-supervised ViT knowledge distillation network with style transfer normalization for colorectal liver metastases survival prediction
半监督ViT知识蒸馏网络与风格迁移标准化在结直肠肝转移生存预测中的应用
01
文献速递介绍
结直肠肝转移(CLM)是一个常见且致命的疾病,癌细胞从结肠或直肠扩散至肝脏(Xi和Xu,2021)。CLM的治疗选择需要对患者的癌症进行彻底的理解和表征,以确定预后并选择适当的治疗方案。尽管传统的分期方法,如临床风险评分(CRS)(Fong等,1999)或肿瘤退化等级(TRG)(Mandard等,1994),已被开发出来以将患者分类为低风险和高风险组,这些组对治疗的反应有所不同,但即使在单一的CRS或TRG内,患者的预后结果也存在显著差异。尽管这些方法至关重要,但它们仍然受到主观性、时间消耗和对广泛专业知识要求的限制。因此,需要更准确和客观的患者风险分类,以改善患者管理和疾病预后。
近年来,利用机器学习(ML)技术从组织病理图像中提供独特的预后信息,已成为研究的热点,这些信息可以补充最新的临床推荐。然而,尽管已有一些研究工作(Van der Laak等,2021),从机器学习中获得预后特征的见解仍然具有挑战性。如果能够可靠地检测和展示所学习的特征具有独立的预后价值,可能会使识别潜在的创新特征和建立可解释的信息成为可能,这对于基于AI的临床决策支持至关重要。此外,在临床背景下进行数据标注对训练机器学习模型至关重要,但这一过程仍然是时间消耗且昂贵的任务(Boehm等,2022)。
基于深度学习的先前努力将临床预后的预测分为两大类。第一类方法使用专门工具(如CellProfiler)(McQuin等,2018),集中精力从组织切片中提取预定义的形态学特征,然后使用统计学或机器学习方法确定哪些预定义特征与生存期或复发相关。第二类方法通过使用弱监督深度学习方法,避免了预定义特征的提取,直接从全切片图像(WSI)预测生存期(Wulczyn等,2020)。然而,要在临床环境中适用,这些应用中使用的组织病理切片必须经过适当的标准化。由于病理学家使用多种染色技术来可视化某些组织特征,而不同染色技术中的解决方案和程序可能会因中心而异,因此切片的染色标准化是必要的,特别是在训练用于图像分类或分割的深度神经网络时,这样可以使模型具有更好的跨数据集的迁移能力(Ciompi等,2017;Tschuchnig等,2020)。
Aastract
摘要
Colorectal liver metastases (CLM) affect almost half of all colon cancer patients and the response to systemicchemotherapy plays a crucial role in patient survival. While oncologists typically use tumor grading scores,such as tumor regression grade (TRG), to establish an accurate prognosis on patient outcomes, includingoverall survival (OS) and time-to-recurrence (TTR), these traditional methods have several limitations. Theyare subjective, time-consuming, and require extensive expertise, which limits their scalability and reliability.Additionally, existing approaches for prognosis prediction using machine learning mostly rely on radiologicalimaging data, but recently histological images have been shown to be relevant for survival predictions byallowing to fully capture the complex microenvironmental and cellular characteristics of the tumor. To addressthese limitations, we propose an end-to-end approach for automated prognosis prediction using histologyslides stained with Hematoxylin and Eosin (H&E) and Hematoxylin Phloxine Saffron (HPS). We first employ aGenerative Adversarial Network (GAN) for slide normalization to reduce staining variations and improve theoverall quality of the images that are used as input to our prediction pipeline. We propose a semi-supervisedmodel to perform tissue classification from sparse annotations, producing segmentation and feature maps.Specifically, we use an attention-based approach that weighs the importance of different slide regions inproducing the final classification results. Finally, we exploit the extracted features for the metastatic nodulesand surrounding tissue to train a prognosis model. In parallel, we train a vision Transformer model in aknowledge distillation framework to replicate and enhance the performance of the prognosis prediction. Weevaluate our approach on an in-house clinical dataset of 258 CLM patients, achieving superior performancecompared to other comparative models with a c-index of 0.804 (0.014) for OS and 0.735 (0.016) for TTR, aswell as on two public datasets. The proposed approach achieves an accuracy of 86.9% to 90.3% in predictingTRG dichotomization. For the 3-class TRG classification task, the proposed approach yields an accuracy of78.5% to 82.1%, outperforming the comparative methods. Our proposed pipeline can provide automatedprognosis for pathologists and oncologists, and can greatly promote precision medicine progress in managingCLM patients.
结直肠肝转移(CLM)几乎影响所有结肠癌患者的一半,而系统性化疗的反应在患者生存中起着至关重要的作用。虽然肿瘤学家通常使用肿瘤分级评分,如肿瘤退行性变分级(TRG),来建立对患者预后的准确预测,包括总体生存期(OS)和复发时间(TTR),但这些传统方法存在一些局限性。它们是主观的、耗时的,并且需要大量的专业知识,这限制了它们的可扩展性和可靠性。此外,现有的基于机器学习的预后预测方法大多依赖于放射学影像数据,但最近的研究表明,组织学图像对于生存预测也具有重要意义,因为它们能够全面捕捉肿瘤的复杂微环境和细胞特征。为了解决这些局限性,我们提出了一种基于组织学切片的自动化预后预测端到端方法,使用了Hematoxylin and Eosin (H&E)和Hematoxylin Phloxine Saffron (HPS)染色切片。
我们首先采用生成对抗网络(GAN)进行切片标准化,以减少染色变异并改善输入到预测管道中的图像的整体质量。我们提出了一种半监督模型,通过稀疏标注执行组织分类,生成分割图和特征图。具体来说,我们使用基于注意力的方法,权衡不同切片区域在最终分类结果中的重要性。最后,我们利用提取的转移性结节及其周围组织的特征,训练预后模型。与此同时,我们在知识蒸馏框架下训练了一个视觉变换器(Vision Transformer,ViT)模型,以复制并增强预后预测的性能。
我们在一个包含258例CLM患者的院内临床数据集上评估了我们的方法,并与其他比较模型进行了比较,获得了优越的表现,OS的C-index为0.804(0.014),TTR为0.735(0.016);同时也在两个公共数据集上进行了评估。该方法在预测TRG二分类任务中实现了86.9%至90.3%的准确率。在3类TRG分类任务中,该方法的准确率为78.5%至82.1%,优于比较方法。我们提出的管道可以为病理学家和肿瘤学家提供自动化的预后预测,极大推动了个性化医学在CLM患者管理中的进展。
Method
方法
In this section, we present our overall framework illustrated inFig. 1. We first describe the dataset and data preparation procedures inSection 3.1. Subsequently, we present our end-to-end pipeline startingwith the normalization method based on GAN to normalize H&E andHPS slides in Section 3.2, Then, we present in Section 3.3 the Semisupervised ViT knowledge distillation network that achieves the prognosis prediction, including overall survival (OS), time-to-recurrence(TTR), and TRG. Section 3.4 presents the experimental setup.The proposed framework is designed to operate in an end-to-endmanner, encompassing both the stain normalization process and thesubsequent steps leading to clinical outcome prediction. This holisticapproach allows our model to process raw WSIs through various stagesof analysis seamlessly. Starting from the stain normalization to addressvariability across H&E and HPS stained slides, the pipeline advancesthrough semi-supervised learning for tissue classification, and culminates in the final prediction of clinical outcomes such as OS and TTR.This end-to-end capability of our approach underscores the efficiency inautomating the analysis of histopathological data, significantly streamlining the process from raw data input to actionable clinical insightswithout the necessity for manual intervention between stages.
在本节中,我们展示了我们的整体框架,如图1所示。首先,在第3.1节中描述了数据集和数据准备过程。随后,在第3.2节中介绍了基于生成对抗网络(GAN)的标准化方法,用于标准化H&E和HPS切片。接着,在第3.3节中,我们展示了半监督的ViT知识蒸馏网络,该网络用于实现预后预测,包括总体生存期(OS)、复发时间(TTR)和肿瘤退化评分(TRG)。第3.4节介绍了实验设置。所提出的框架设计为端到端的工作方式,涵盖了从染色标准化过程到临床结果预测的各个步骤。这一整体方法使我们的模型能够无缝处理原始的全切片图像(WSIs)并通过多个分析阶段。首先通过染色标准化来解决H&E和HPS染色切片之间的变异性,然后通过半监督学习进行组织分类,最后预测临床结果,如OS和TTR。我们方法的端到端能力突出了其在自动化组织病理学数据分析中的效率,显著简化了从原始数据输入到可操作的临床见解的整个过程,且无需在各个阶段之间进行人工干预。
Conclusion
结论
To conclude, the proposed end-to-end approach for prognosis prediction, based on machine learning of WSI features and semi-supervisedtissue classification, and knowledge distillation with Vision Transformer (ViT) achieved promising results in predicting patient prognosisfor colorectal liver metastasis. Moreover, the model was able to predictTRG values with a high degree of accuracy, indicating its potential usein guiding treatment decisions. The proposed approach could provideautomated prognosis information for pathologists and oncologists, andcould greatly promote precision medicine progress in managing CLMpatients. Future research will focus on evaluating the performanceof the proposed pipeline on larger datasets and on its clinical implementation. Additionally, further analysis into the cellular level of theWSI could help extract features related to the cellular distribution andimmunological infiltration that have been shown to be related to theprognosis.
总之,基于WSI特征和半监督组织分类的机器学习以及基于Vision Transformer(ViT)的知识蒸馏的端到端预后预测方法,在结直肠肝转移患者的预后预测中取得了良好的结果。此外,该模型能够高精度预测TRG值,表明其在指导治疗决策方面具有潜在的应用价值。该方法可以为病理学家和肿瘤学家提供自动化的预后信息,并能够在CLM患者管理中大力推动精准医学的发展。未来的研究将重点评估该方法在更大数据集上的表现及其临床应用。此外,进一步分析WSI的细胞层次可能有助于提取与细胞分布和免疫浸润相关的特征,这些特征已被证明与预后相关。
Results
结果
In order to evaluate the performance of our normalization model,we compared it to two other commonly used methods for staining normalization: Macenko (Macenko et al., 2009) and Reinhard (Reinhardet al., 2001). As shown in Table 2, we also selected two evaluationtechniques that are used to assess the quality of the image normalization process: the Structure Similarity Index Matrix (SSIM) (Wanget al., 2004) and the Pearson correlation coefficient (PCC) (Rodgers andNicewander, 1988).On one hand, SSIM is used to measure the structural information,luminance, and contrast between the source and processed image andits index denotes the reference metric:SSIM(𝑥, 𝑦) = (2𝜇𝑥𝜇𝑦 + 𝑐1𝜇𝑥 2 + 𝜇𝑦 2 + 𝑐1) (2𝜎𝑥𝑦 + 𝑐2𝜎𝑥 2 + 𝜎𝑦 2 + 𝑐2)(11)
On the other hand, PCC measures the linear correlation between thetwo images and its range from 0 to 1. A value of 0 indicates that thereis no similarity between the two images:
PCC(𝑥, 𝑦) =∑𝑖( 𝑥𝑖 − 𝜇𝑥) (𝑦𝑖 − 𝜇𝑦)√∑𝑖( 𝑥𝑖 − 𝜇𝑥)2√∑ 𝑖𝑦𝑖 − 𝜇𝑦 )2(12)
The proposed model yielded significant improvement in both metrics. Sample results of the normalized slide obtained with the GANmodel are shown in Fig. 4.
为了评估我们提出的正规化模型的性能,我们将其与另外两种常用的染色正规化方法进行比较:Macenko(Macenko et al., 2009)和Reinhard(Reinhard et al., 2001)。如表2所示,我们还选择了两种评估技术,用于评估图像正规化过程的质量:结构相似性指数矩阵(SSIM)(Wang et al., 2004)和皮尔逊相关系数(PCC)(Rodgers and Nicewander, 1988)。
在一方面,SSIM(结构相似性指数)用于衡量源图像与处理后图像之间的结构信息、亮度和对比度,其指标定义为参考度量:SSIM(x,y)=(2μxμy+c1)(μx2+μy2+c1)×(2σxy+c2)(σx2+σy2+c2)\text{SSIM}(x, y) = \frac{(2\mu_x \mu_y + c_1)}{(\mu_x2 + \mu_y2 + c_1)} \times \frac{(2\sigma{xy} + c_2)}{(\sigma_x2 + \sigma_y2 + c_2)}其中,μx\mu_x 和 μy\mu_y 分别为图像 xx 和 yy 的均值,σx2\sigma_x2 和 σy2\sigma_y2 为它们的方差,σxy\sigma{xy} 为它们的协方差,c1c_1 和 c2c_2 是稳定常数。另一方面,PCC(皮尔逊相关系数)用于测量两个图像之间的线性相关性,其范围从0到1。值为0表示两个图像之间没有相似性:
PCC(x,y)=∑i(xi−μx(yi−μy)∑i(xi−μx)2∑i(yi−μy)2\text{PCC}(x, y) = \frac{\sum_i (x_i - \mu_x)(y_i - \mu_y)}{\sqrt{\sum_i (x_i - \mu_x)2} \sqrt{\sum_i (y_i - \mu_y)2}}其中,xix_i 和 yiy_i 为图像 xx 和 yy 的像素值,μx\mu_x 和 μy\mu_y 为它们的均值。
所提出的模型在这两个评估标准上均显示出显著的改善。图4展示了使用GAN模型获得的正规化切片的样本结果。
Figure
图
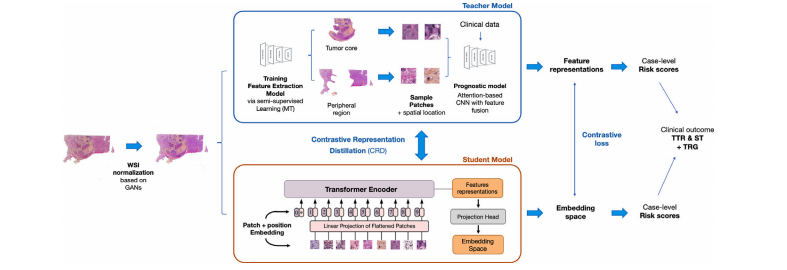
Fig. 1. Overview of the semi-supervised ViT knowledge distillation network. First, the WSIs are normalized using the GAN model. Then, a Mean Teacher (MT) approach is trainedto extract key features related to prognostic from the selected ROIs: tumor core and peripheral region. This part of the model is capable of doing the tissue classification taskand will generate the classification maps. Using the extracted features from sample patches from the tumor core and the peripheral region, we train the Prognosis model throughan attention mechanism with clinical data. In parallel, Contrastive Representation Distillation (CRD) uses a c
Fig. 1. 半监督ViT知识蒸馏网络概述。首先,使用GAN模型对全切片图像(WSIs)进行标准化。然后,训练一个Mean Teacher(MT)方法,提取与预后相关的关键特征,来自选择的感兴趣区域(ROIs):肿瘤核心区和外围区域。该模型部分能够执行组织分类任务,并生成分类图。通过使用从肿瘤核心和外围区域样本补丁中提取的特征,我们通过注意力机制和临床数据训练预后模型。与此同时,Contrastive Representation Distillation(CRD)使用对比学习方法…
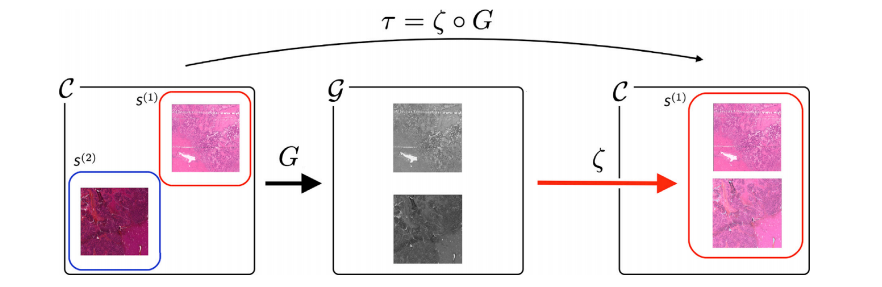
Fig. 2. Overview of the stain-style transfer model. The model 𝜏 is composed of two transformations: Gray-normalization 𝐺 and style-generator 𝜁. 𝐺 standardizes each stain-style,H&E and HPS, and 𝜁 colorizes gray images following the stain-style chosen as reference, in this case H&E.
Fig. 2. 染色风格转移模型概述。模型𝜏由两个变换组成:灰度标准化𝐺和风格生成器𝜁。𝐺对每种染色风格(H&E和HPS)进行标准化,𝜁根据选择的参考染色风格(此处为H&E)将灰度图像进行上色。
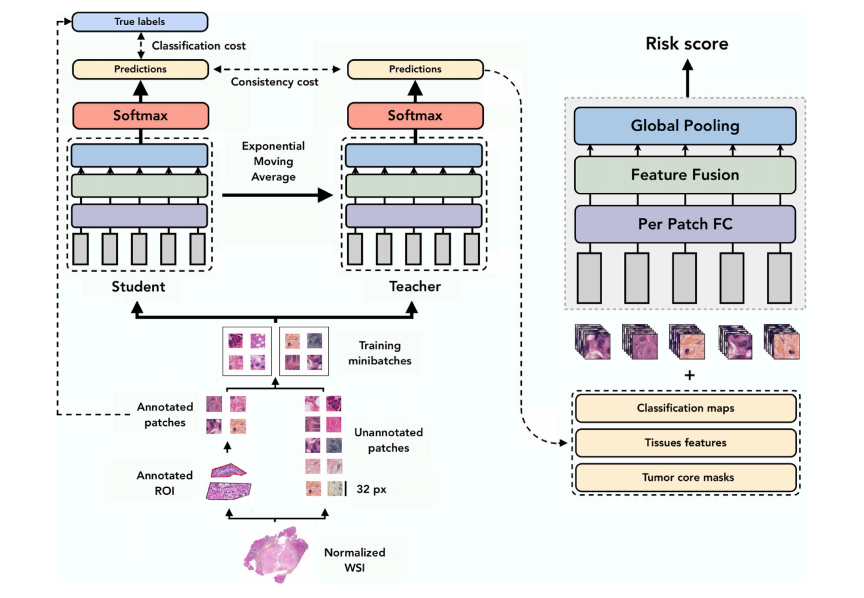
Fig. 3. Illustration of the Mean Teacher Approach for tissue classification in the context of survival analysis on input patches from histopathology slides. The student and teacher models are jointly trained using exponential moving average (EMA) to generate 𝑝1 and 𝑝2 tissue class probability distributions, respectively. The loss function is defined as the cross-entropy between both predictions, promoting consistency between the student and teacher models. The teacher model consists of per-patch fully connected layers, followed by feature fusion and global pooling to obtain a risk score. As an output, the model produces risk scores related to survival and TRG of the CLM lesion.
Fig. 3. 生存分析中组织分类的均值教师方法说明,输入来自组织病理学切片的样本块。学生和教师模型通过指数移动平均(EMA)共同训练,分别生成𝑝1和𝑝2组织类别概率分布。损失函数定义为两个预测之间的交叉熵,促进学生和教师模型之间的一致性。教师模型由每个切片的全连接层组成,接着进行特征融合和全局池化以获得风险评分。模型的输出为与CLM病变的生存和肿瘤退化评分(TRG)相关的风险评分。
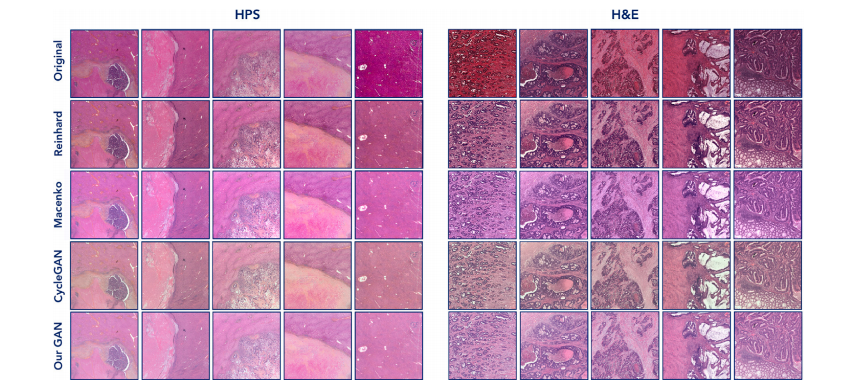
Fig. 4. Sample results of normalized slides with the proposed GAN model and the comparative methods. We can see a homogeneity in the color distribution in the obtained slidesdespite variability in the original slides, that can be either HPS or H&E stained
图4 使用所提出的GAN模型和比较方法进行正规化切片的样本结果。可以看到,尽管原始切片(无论是HPS染色还是H&E染色)存在一定的变异性,但在获得的切片中,颜色分布表现出了一致性。
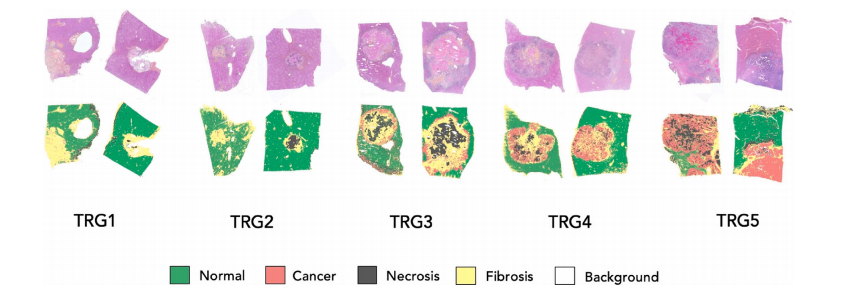
Fig. 5. Sample results for the classification task. In the first row, we show two normalized slides for each TRG score. In the second row, the correspondent classification map isgenerated with the SST model.
图5 分类任务的样本结果。第一行显示了每个TRG评分的两个正规化切片。第二行显示了使用SST模型生成的对应分类图。
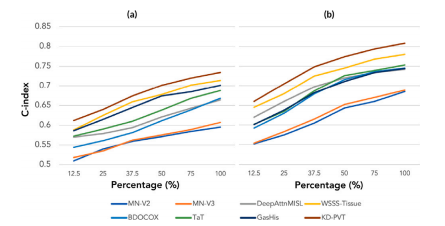
Fig. 6. Model performances for survival and TTR. It is represented in c-index curvesfor (a) TTR, and (b) OS prediction. We can observe that the proposed model yieldsimproved accuracy to the comparative models for both prediction tasks.
图6 生存期和复发时间预测模型表现。以c-index曲线表示(a)复发时间(TTR)和(b)总体生存期(OS)预测。可以观察到,提出的模型在这两个预测任务中相较于比较模型具有更高的准确性。
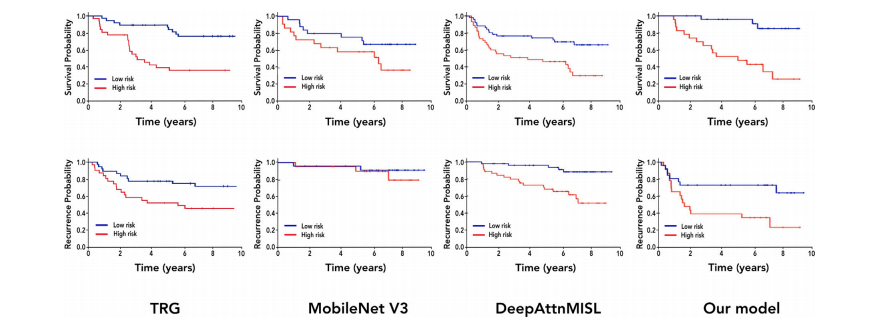
Fig. 7. Kaplan–Meier curves for OS prediction (first row) and TTR (second row). From left to right: TRG stratification (1–2 vs 3–5), MobileNetV3, DeepAttnMISL, and our model(SSL + KD)
图 7. OS 预测(第一行)和 TTR(第二行)的 Kaplan-Meier 曲线。从左到右依次为:TRG 分层(1-2 vs 3-5),MobileNetV3,DeepAttnMISL 和我们的模型(SSL + KD)。
Table
表
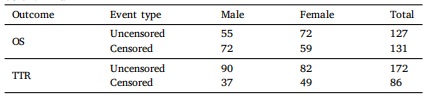
Table 1Distribution of uncensored (deaths and recurrences) and censored cases by gender forOS and TTR
表1按性别分组的未被截断(死亡和复发)和被截断病例在OS和TTR中的分布
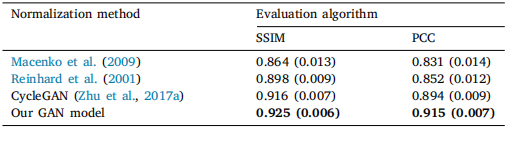
Table 2Normalization performance of the proposed model compared to Macenko, Reinhard, and CycleGAN approaches using the SSIM and PCC evaluation measures
Table 2 正规化模型性能比较,使用SSIM和PCC评估指标,与Macenko、Reinhard和CycleGAN方法进行对比。
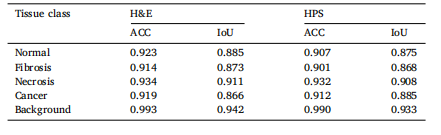
Table 3Class-wise accuracy, performance results for H&E and HPS slide acquisition methods,and IoU.
表3 各类准确率、H&E 和 HPS 切片采集方法的性能结果,以及 IoU。
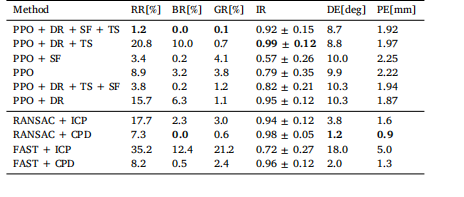
Table 4Summary of evaluation results on synthetic US reconstruction dataset. The values areaveraged over the 5 human datasets from the ITIS virtual population. Bold numbersare the best column-wise values
表 4 合成 US 重建数据集的评估结果总结。数值是对来自 ITIS 虚拟人群的 5 个人体数据集进行平均的结果。加粗的数字是每列的最佳值。
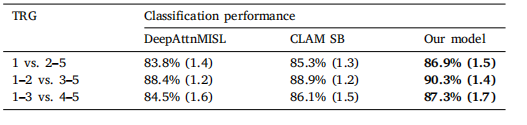
Table 5TRG prediction performance for our approach, compared to DeepAttnMISL and CLAMSB models, in 3 different TRG dichotomizations. Statistically significant results areshown in bold
表 5 我们的方法在 3 种不同的 TRG 二分类中的 TRG 预测性能,与 DeepAttnMISL 和 CLAMSB 模型进行比较。统计显著的结果用粗体表示。
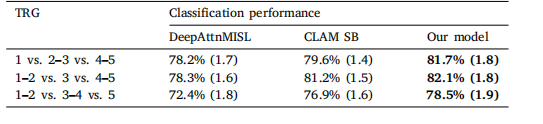
Table 6TRG prediction performance for our approach, compared to DeepAttnMISL and CLAMSB models, in 3 different TRG classifications. Statistically significant results shown inbold
表 6 我们的方法在 3 种不同的 TRG 分类中的 TRG 预测性能,与 DeepAttnMISL 和 CLAMSB 模型进行比较。统计显著的结果用粗体表示。
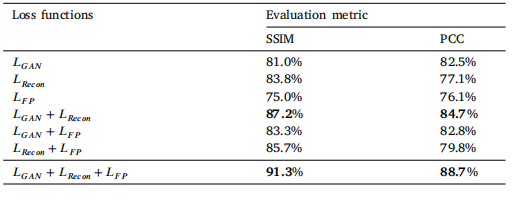
Table 7Ablation studies results for the WSI normalization. Top results are indicated in bold.
表 7 WSI 归一化的消融研究结果。最好的结果用粗体表示。
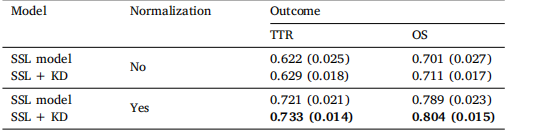
Table 8 Model performance (c-index) with and without the normalization step
表 8 模型性能(c-index)有无归一化步骤的比较
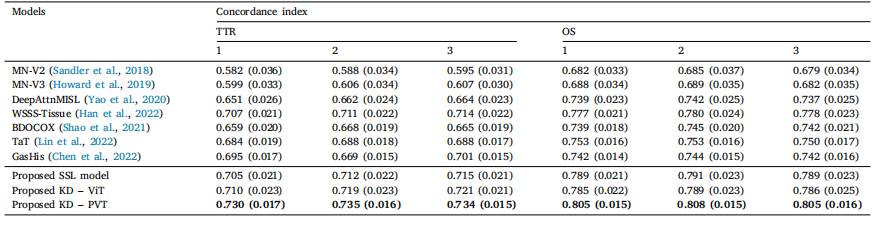
Table 9Model performances expressed in concordance index comparing different models on TTR and OS prediction using various tumor aggregation strategies: 1. max pooling, 2. meanpooling, and 3. weighted average pooling. Top result is shown in bold.
表 9 使用不同肿瘤聚合策略(1. 最大池化,2. 均值池化,3. 加权平均池化)比较不同模型在TTR和OS预测中的性能,结果以一致性指数(C-index)表示。最佳结果以粗体显示。
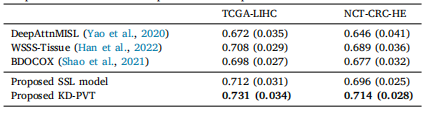
Table 10Comparison of survival models performances on public datasets.
Table 10 公共数据集上生存模型性能的比较