Title
题目
RFMiD: Retinal Image Analysis for multi-Disease Detection challenge
RFMiD:多疾病检测的视网膜图像分析挑战
01
文献速递介绍
眼部疾病的普遍性与上升趋势
根据世界卫生组织 (WHO) 2019 年《全球视觉报告》,目前全球约有 22 亿人存在视力损害,其中至少有 10 亿病例本可以预防或尚未得到治疗。这些超过 10 亿的人生活在可治疗或可预防的病况中,仅仅因为无法获得必要的眼科护理。由于人口快速增长、老龄化和生活方式的改变,未来全球对眼科护理的需求预计将大幅增加。此外,视力损害因生产力损失造成了巨大的全球经济负担(WHO,2019)。眼底影像的临床价值彩色眼底摄影通过使用配备低功率显微镜的专用相机,非侵入性地捕捉眼睛内部表面的图像。眼底影像被广泛用于临床筛查、检测疾病的存在以及监测其随时间的变化。在眼科护理领域,世界面临着诸多挑战,包括预防、治疗和康复服务覆盖率和质量的不平等。通过详细的临床观察,眼底特征不仅可以提供眼部疾病的信息,还可用于识别多种长期病症的早期指标,如糖尿病、中风、高血压、动脉硬化、心血管疾病、神经退行性疾病、肾病以及脂肪肝病(MacGillivray et al., 2014;Yang et al., 2015;Chang et al., 2016)。因此,视网膜不仅仅是反映眼健康的器官,其评估对判断全身性健康问题也具有重要意义。通过眼科筛查以及及时的检测和治疗,可以避免视力损害和身体其他部位的损伤。自动化视网膜图像分析的进展自动化视网膜图像分析技术可用于早期检测和诊断眼部病理,从而避免视力损害,减少眼科医生和验光师的手动工作量,并节约诊断成本和时间。在多数发达国家,眼科医生和验光师与人口的比例约为 1:10,000;而在发展中国家,这一比例为 1:600,000,在农村地区甚至每位眼科医生需服务数百万人(Holden 和 Resnikoff,2002)。专业人员的短缺是发展中国家视力问题高发的主要原因。因此,转向自动化计算机筛查系统可以帮助检测视网膜的主要组成部分,分类正常、异常和未知的眼底图像,从而显著提高疾病在早期阶段的检测和治疗率。近年来,计算能力和人工智能技术的进步为生物医学科学家提供了满足临床需求的新工具(Shortliffe 和 Blois, 2006;Patton et al., 2006)。为了满足这一需求,原始眼底图像及其相关标注成为研究界开发、验证和比较技术的重要基础。在多疾病检测中,标注是基于临床记录和视野对特定图像赋予的标签。这一需求推动了研究组开发并共享眼底图像数据库。在过去的几十年中,通过糖尿病视网膜病变(DR)筛查项目收集的一些数据集包括 IDRiD (Porwal et al., 2020, 2018a)、Messidor (Decencière et al., 2014) 和 EyePACS (Cuadros 和 Bresnick, 2009)。此外,代表严重病理如青光眼的数据集包括 Drishti-GS (Sivaswamy et al., 2015)、RIM-ONE (Fumero et al., 2011) 和 Refuge (Orlando et al., 2020);代表老年黄斑变性(AMD)的数据集包括 ARIA (Farnell et al., 2008)、STARE (Hoover et al., 2000) 和 Age-Related Eye Disease Study Research Group (2001)。
多疾病检测的必要性与数据集限制参与 DR 筛查项目的患者可能同时患有青光眼和 AMD 等其他疾病,因此多疾病检测至关重要。当前可用于多疾病检测的数据集较少,例如公开的 ODIR 数据集(ODIR-2019, 2019)。但该数据集的异常标注仅限于患者级别,而非眼睛级别;并且它仅关注 8 类标签(大多数异常被归类为“其他”类别进行评估)。现有的多疾病检测数据集要么是私有的,要么仅涵盖最常见的病理。然而,诸如中央视网膜动脉阻塞或前部缺血性视神经病变等威胁视力的罕见病理的检测和诊断同样重要。由于缺乏表示罕见病理的数据集,尚未开发出用于自动检测罕见病理的方法。因此,自动筛查系统通常忽略这些在常规临床检查中可能出现的罕见病理,这限制了眼科医生对自动筛查系统的采用。RFMiD 数据集与 RIADD 挑战赛为了解决这些问题,我们引入了一个新的数据集——视网膜眼底多疾病图像数据集(Retinal Fundus Multi-Disease Image Dataset, RFMiD)(Pachade et al., 2021b)。此外,该数据集被用作 2021 年 国际生物医学成像研讨会(ISBI 2021)上组织的 “多疾病检测的视网膜图像分析”挑战赛(RIADD)的基础数据集。RFMiD 数据集提供了 3200 张彩色眼底图像,包含代表正常或异常类别的图像级别标签,并由视网膜专家标注了 45 种不同的疾病/异常。此次挑战赛汇集了生物医学和计算机视觉研究人员,旨在开发具有通用性的视网膜筛查技术,与以往专注于特定疾病检测/分类的研究方法不同。在算法开发的初始阶段,参赛者获得了带有标注的训练数据集。随后,参与最终阶段的团队根据其算法在验证数据集上的表现入围。最终,获胜者根据算法在测试数据集上的表现进行评估。算法的表现通过其结果与标注真值的接近程度来衡量。挑战赛分为两个子任务:疾病筛查和疾病/病理分类。挑战赛的独特贡献以下是此次挑战赛的主要贡献:
聚焦罕见病理:挑战赛通过提供涵盖更广泛病理的数据集,填补了自动检测罕见病理方法的研究空白。提升模型通用性:挑战赛鼓励开发适用于更广范围病理的解决方案,促进了多功能、鲁棒筛查系统的发展,与以往专注于特定疾病的研究方法形成鲜明对比。应对数据问题:挑战赛解决了多疾病检测中数据稀缺和类别不平衡的问题,通过大量实践提示,为研究人员和从业者提供了宝贵的知识。推动新解决方案:挑战赛催生了多种新的多疾病检测解决方案,凸显了协作努力的有效性,以及此次挑战在推进该领域技术发展中的重要性。
文章结构本文的余下部分安排如下:第 2 节 简要回顾多疾病检测的视网膜图像分析;第 3 节 详细介绍 RFMiD 数据集;第 4 节 描述挑战赛的组织情况;第 5 节 总结表现最佳的参赛方案;第 6 节 介绍挑战赛中使用的性能评估方法;第 7 节 展示两个子任务的参赛结果和团队比较;第 8 节 提供讨论和结论。
Abatract
摘要
In the last decades, many publicly available large fundus image datasets have been collected for diabeticretinopathy, glaucoma, and age-related macular degeneration, and a few other frequent pathologies. Thesepublicly available datasets were used to develop a computer-aided disease diagnosis system by training deeplearning models to detect these frequent pathologies. One challenge limiting the adoption of a such systemby the ophthalmologist is, computer-aided disease diagnosis system ignores sight-threatening rare pathologiessuch as central retinal artery occlusion or anterior ischemic optic neuropathy and others that ophthalmologistscurrently detect. Aiming to advance the state-of-the-art in automatic ocular disease classification of frequentdiseases along with the rare pathologies, a grand challenge on ‘‘Retinal Image Analysis for multi-DiseaseDetection’’ was organized in conjunction with the IEEE International Symposium on Biomedical Imaging (ISBI -2021). This paper, reports the challenge organization, dataset, top-performing participants solutions, evaluationmeasures, and results based on a new ‘‘Retinal Fundus Multi-disease Image Dataset’’ (RFMiD). There were twoprincipal sub-challenges: disease screening (i.e. presence versus absence of pathology — a binary classificationproblem) and disease/pathology classification (a 28-class multi-label classification problem). It received apositive response from the scientific community with 74 submissions by individuals/teams that effectivelyentered in this challenge. The top-performing methodologies utilized a blend of data-preprocessing, dataaugmentation, pre-trained model, and model ensembling. This multi-disease (frequent and rare pathologies)detection will enable the development of generalizable models for screening the retina, unlike the previousefforts that focused on the detection of specific diseases.
近年来,为糖尿病视网膜病变、青光眼和老年黄斑变性等常见疾病,以及其他一些常见病理,已经收集了许多公开可用的大型眼底图像数据集。这些公开数据集被用来通过训练深度学习模型开发计算机辅助疾病诊断系统,以检测这些常见病理。然而,限制眼科医生采用此类系统的一个挑战是,这些计算机辅助疾病诊断系统忽略了威胁视力的罕见病理,例如中央视网膜动脉阻塞或前部缺血性视神经病变等,而这些病理目前是眼科医生能够检测到的。为了推动在自动眼病分类领域对常见疾病和罕见病理的研究进展,在2021年IEEE国际生物医学成像研讨会(ISBI 2021)上举办了一项名为“多疾病检测的视网膜图像分析” (Retinal Image Analysis for multi-Disease Detection) 的重大挑战赛。本文汇报了此次挑战赛的组织情况、数据集、表现最佳的参赛方案、评估方法及基于全新“视网膜眼底多疾病图像数据集”(RFMiD)的结果。该挑战赛设有两个主要子挑战:疾病筛查(即病理的存在与否——一个二分类问题)和疾病/病理分类(一个28类的多标签分类问题)。挑战赛受到了科研社区的积极响应,共收到来自个人或团队的74份参赛提交,成功参与了此次挑战。表现最佳的方法采用了数据预处理、数据增强、预训练模型以及模型集成的组合。这种针对多疾病(包括常见和罕见病理)的检测方法,有助于开发可推广的视网膜筛查模型,与以往专注于特定疾病检测的研究方法不同。
Conclusion
结论
In this paper, we have presented the details of the RIADD challenge,including detailed information about the dataset, the challenge organization, all the top-performing competing solutions, used evaluationmetrics, and final individual and averaged results for both sub-tasks,i.e., disease screening and multi-disease classification.The top performing methods for multi-disease detection demonstrated that the problem of multi-disease detection, limited data, andclass imbalance can be handled using a substantial number of commontips: (1) appropriate data pre-processing, (2) data augmentation tohandle the problem of data scarcity and class imbalance problem,(3) transfer learning using the pre-trained model, (4) modification ofloss function, (5) model ensembling. Additionally, to improve modelperformance, many teams used the EfficientNet framework as the backbone model for the network, it learns gradient features by uniformlyscaling all dimensions of depth/width/resolution using a compoundcoefficient. For both the sub-challenges, the best performing proposed network by team ‘‘KAMATALAB’’ along with these common tips,adopted post-processing steps to avoid over-fitting. As this is the firstpublicly available multi-disease dataset, most of the teams did not usean external dataset. The only team that used external unlabeled datasetis ‘‘IGSTfencing’’, it used ODIR-2019 (ODIR, 2019) dataset and got 2ndand 6th position in sub-challenge 1 and sub-challenge 2, respectively.Teams are observed (from Tables 6 and 7) to perform poorly in themulti-disease classification task as compared to the disease screeningtask that reduced the overall final score. The major reason seems to bethe difficulty in rare pathology classification which in turn decreasesthe overall performance of the multi-disease classification task. Thesame can also be seen from the box plot (Fig. 4), showing the distribution of ‘‘Final Score’’ in sub-challenge-2 across the top performing eightteams for all 28 disease categories on test set. We observe that detectionperformance generally increases with the size of disease categories.Other factors probably come into play, such as the subtlety of diseases:optic disc pallor (ODP) is frequent but subtle, retinitis pigmentosa(RP) is rare but generally obvious. The ‘Other’ category, which isboth small and varied, is particularly challenging. We note that the8 teams have similar scores for frequent categories: the performancedifference between teams largely comes from rare categories, wherethe variability between teams is high.
本文详细介绍了RIADD挑战赛的相关内容,包括数据集的详细信息、挑战赛的组织、所有表现最佳的参赛方案、使用的评估指标,以及两个子任务(即疾病筛查和多疾病分类)的最终个体及平均结果。多疾病检测的最佳方法表明,面对多疾病检测、数据稀缺和类别不平衡问题时,可以通过一些常见的技巧来解决:1.适当的数据预处理,2.数据增强以应对数据稀缺和类别不平衡问题,3.使用预训练模型进行迁移学习,4.修改损失函数,5.模型集成。此外,为了提高模型性能,许多团队使用了EfficientNet框架作为网络的骨干模型,它通过统一缩放深度、宽度和分辨率来学习梯度特征,使用复合系数。对于两个子挑战,表现最佳的团队“KAMATALAB”采用了这些常见技巧,并在此基础上加入了后处理步骤,以避免过拟合。由于这是第一个公开的多疾病数据集,大部分队伍并未使用外部数据集。唯一使用了外部未标记数据集的团队是“IGSTfencing”,他们使用了ODIR-2019(ODIR,2019)数据集,并在子挑战1和子挑战2中分别获得了第2和第6的位置。从表6和表7中可以看到,队伍在多疾病分类任务中的表现较差,相比之下,疾病筛查任务的表现更好,这降低了总体的最终得分。主要原因似乎在于稀有病理分类的困难,这反过来影响了多疾病分类任务的整体表现。从箱线图(图4)中也可以看出,展示了测试集中前8支队伍在子挑战2中28个疾病类别的“最终得分”分布。我们观察到,随着疾病类别规模的增加,检测性能通常会有所提升。其他可能的因素包括疾病的细微差异:视盘苍白(ODP)频繁但细微,色素性视网膜炎(RP)罕见但通常显著。“其他”类别既小又多样,尤其具有挑战性。我们注意到,8支队伍在频繁类别的得分相似,队伍间的表现差异主要出现在稀有类别,稀有类别之间的差异较大。
Results
结果
This section reports and discusses the results of the challenge.Performance of all competing solutions on both sub-challenge including their leaderboard rank. The final rank on the leaderboard wasdetermined by using performance in both sub-challenges as follows:Final Score = 0.5(Sub-challenge-1 Score) + 0.5(Sub-challenge-2Score)This section presents the performance of the proposed model byall participating teams for disease screening and classification tasks.Online result submission and automated evaluation system was builtand presented on our website for the participants to submit and evaluate their results. Evaluated results are made publicly available on theleaderboard of our website. All the results submitted by competingteams were analyzed using the evaluation measures given in Section 6.This measure generated the AUC score for sub-challenge-1 and AUCand mAP score for sub-challenge-2. We got total 74 submissions byindividuals/teams for the challenge. Amongst them, the top performing8 teams were invited to participate in the final online challenge.Table 6 summarizes the best submission performance of the top 8teams on the validation set. The column ‘‘Disease Risk Score’’ showsthe performance of the individual solution for disease screening task(sub-challenge 1) and the column ‘‘Multi-Disease Average Score’’ givesthe performance for the disease classification task (sub-challenge 2).The column ‘‘Final Score’’ is an aggregate performance of the individualsolution on sub-challenge 1 and sub-challenge 2. The final placementfor each subtask is determined using the ‘‘Final Score’’ from the leaderboard. Furthermore, we conducted an analysis for both tasks (SC1:sub-challenge-1 and SC2: sub-challenge-2), evaluating F1 score andSpecificity. The performance of top 8 teams is reported in the sametable. The ‘‘Final Score’’ of all the participating individual/ teamsin decreasing order of their performance in sub-challenge-1 and subchallenge-2 on validation set is shown in Figs. 2 and 3 respectively.Fig. 4 shows the distribution of ‘‘Final Score’’ in sub-challenge-2 acrossthe top performing eight teams for all 28 disease categories on testset. The disease categories are ranked by decreasing order of theirfrequency of appearance in the RFMiD dataset.
本部分报告并讨论了该挑战赛的结果。包括两个子挑战中所有参赛方案的表现及其排行榜排名。排行榜的最终排名由两个子挑战的表现决定,计算公式如下:最终得分 = 0.5 ×(子挑战1得分)+ 0.5 ×(子挑战2得分)本部分呈现了各参赛队伍在疾病筛查和分类任务中的模型表现。我们为参与者搭建了在线结果提交和自动评估系统,供其提交和评估结果。评估结果已在我们的网站排行榜上公开可见。所有参赛队伍提交的结果均按照第6节中给出的评估指标进行了分析。这些指标生成了子挑战1的AUC(曲线下面积)得分,以及子挑战2的AUC和mAP(平均精度均值)得分。
此次挑战赛共收到来自个人或团队的74次提交。其中,表现最优秀的8支队伍受邀参加了最终的在线挑战赛。表6总结了这8支队伍在验证集上的最佳提交表现。“疾病风险得分”列展示了各参赛方案在疾病筛查任务(子挑战1)中的表现,而“多疾病平均得分”列则反映了疾病分类任务(子挑战2)的表现。“最终得分”为参赛方案在子挑战1和子挑战2中的综合表现。排行榜的最终排名基于排行榜上的“最终得分”确定。此外,我们对两个任务(子挑战1:SC1和子挑战2:SC2)进行了分析,评估了F1得分和特异性。表中还报告了前8支队伍的表现。图2和图3分别展示了所有参赛个人/团队在验证集中子挑战1和子挑战2中“最终得分”的降序排列。图4则展示了前8支表现最佳队伍在测试集中针对所有28个疾病类别的子挑战2“最终得分”的分布情况。疾病类别按照其在RFMiD数据集中出现频率的降序排列。
Figure
图
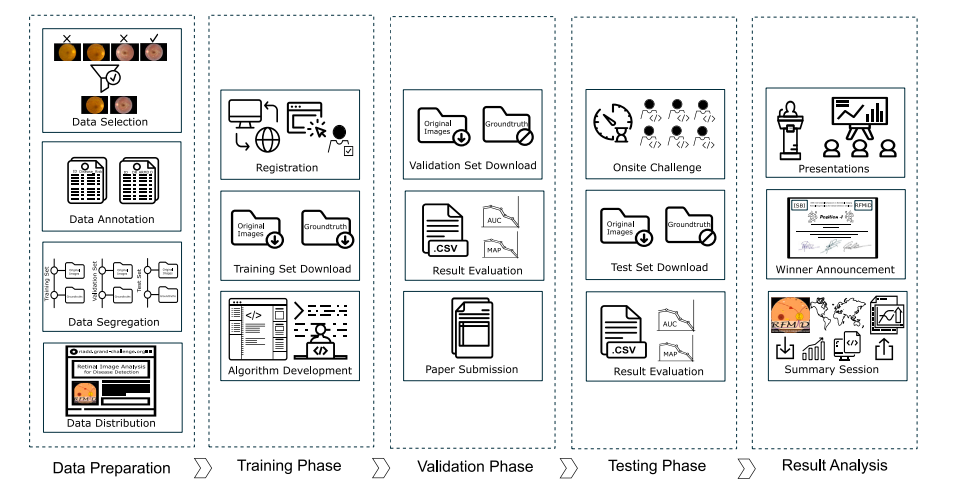
Fig. 1. Workflow of the ISBI - 2021: Retinal Image Analysis for multi-Disease Detection (RIADD) Challenge.
图 1. ISBI - 2021:多疾病检测的视网膜图像分析 (RIADD) 挑战赛工作流程。
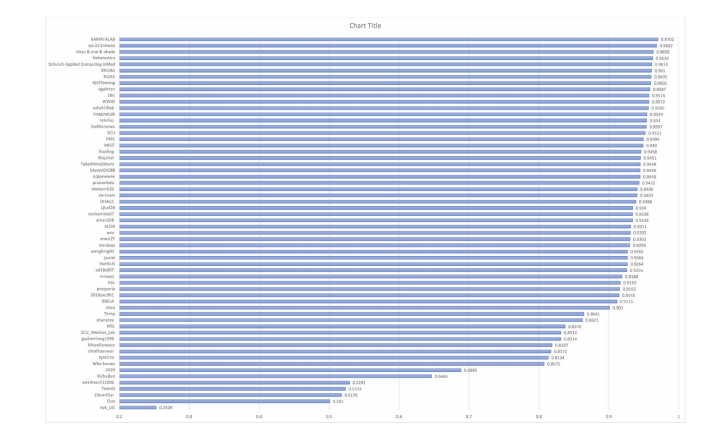
Fig. 2. The performance (Final Score) of all the participating individual/ teams in sub-challenge-1 on validation set.
图2. 所有参赛个人/团队在子挑战1中在验证集上的表现(最终得分)。
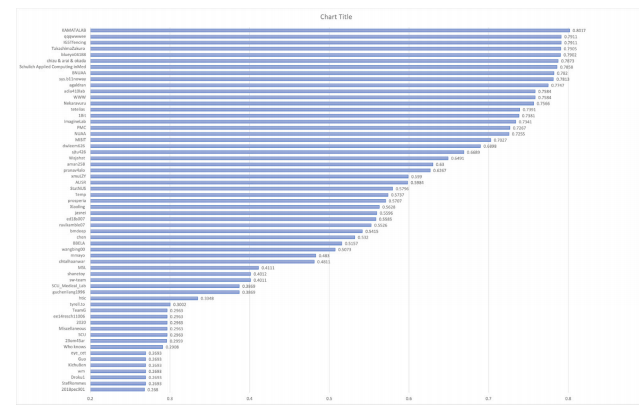
Fig. 3. The performance (Final Score) of all the participating individual/ teams in sub-challenge-2 on validation set.
图 3. 所有参赛个人/团队在验证集子挑战 2 中的表现(最终得分)。
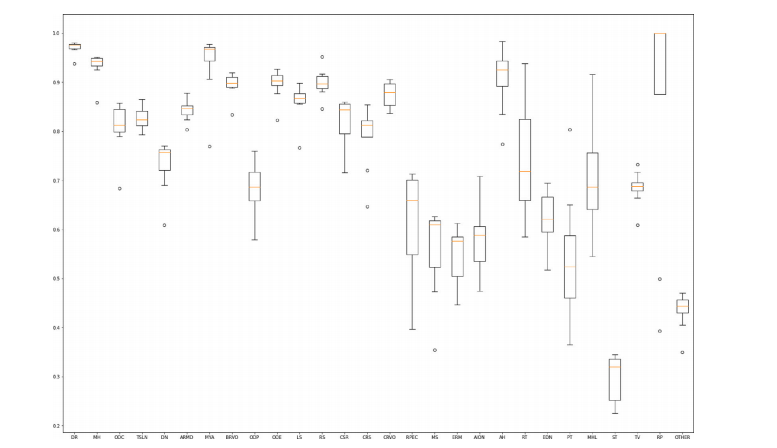
Fig. 4. Box plot showing the distribution of ‘‘Final Score’’ in sub-challenge-2 across the top performing eight teams for all 28 disease categories on test set. The disease categoriesare ranked by decreasing order of their frequency of appearance in the RFMiD dataset
图 4. 箱线图显示了测试集中前 8 支团队在子挑战 2 中针对 28 种疾病类别的“最终得分”分布。疾病类别按照其在 RFMiD 数据集中出现频率的降序排列。
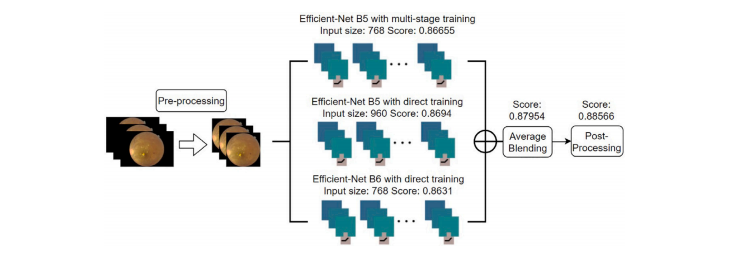
Fig. A.5. The overall structure of the proposed system for multi-disease detection
图 A.5. 提出系统的多疾病检测总体结构。
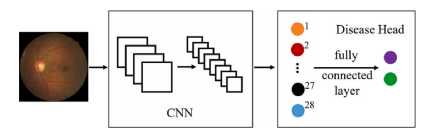
Fig. A.6. The BNUAA designed module.
图 A.6. BNUAA 设计的模块。
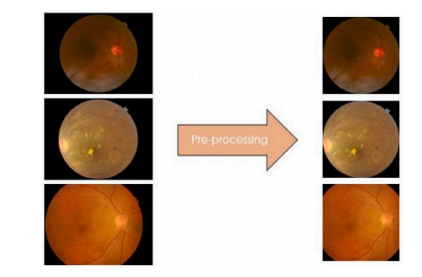
Fig. A.7. Pre-processing for three digital fundus camera images.
图 A.7. 三种数字眼底相机图像的预处理流程。
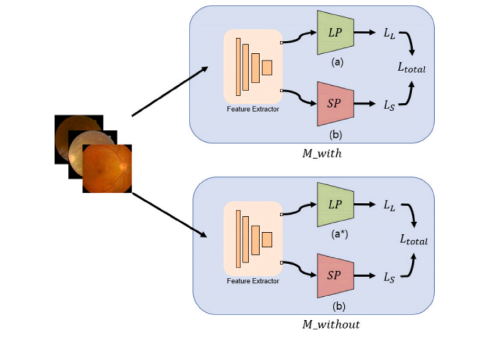
Fig. A.8. 𝐿𝑃 is the classifier for large positive class and 𝑆𝑃 is the classifier for smallpositive class. 𝑀𝑤𝑖𝑡ℎ is composed of 13 class (a), and 𝑀𝑤𝑖𝑡ℎ𝑜𝑢𝑡 is composed of 12class (a).
图 A.8. 𝐿𝑃 是用于大正类的分类器,𝑆𝑃 是用于小正类的分类器。𝑀𝑤𝑖𝑡ℎ 由 13 个类别组成 (a),而 𝑀𝑤𝑖𝑡ℎ𝑜𝑢𝑡 由 12 个类别组成 (a)。
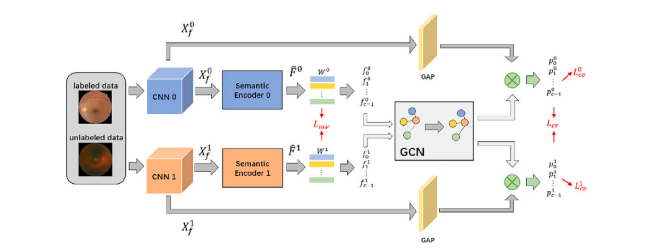
Fig. A.9. The pipeline of proposed semi-supervised siamese graph convolutional network (SS-GCN) framework. Both labeled and unlabeled images are encoded with the semanticencoder to obtain semantic features. Then, the multi-view GCN incorporates the features to model relations between each category to construct the final classifier. Finally, thefeature vector after GAP is fed into the classifier to get the final results. 𝐿𝑚𝑣, 𝐿𝑐𝑒 , and 𝐿𝑐𝑟 are terms of the loss function to regularize different parts of SS-GCN
图 A.9. 提出的半监督孪生图卷积网络(SS-GCN)框架的流程图。标注和未标注的图像均通过语义编码器进行编码以获得语义特征。然后,多视图 GCN 融合特征以建模各类别之间的关系,从而构建最终分类器。最后,经过全局平均池化(GAP)后的特征向量被输入分类器以获得最终结果。𝐿𝑚𝑣、𝐿𝑐𝑒 和 𝐿𝑐𝑟 是用于正则化 SS-GCN 不同部分的损失函数项。
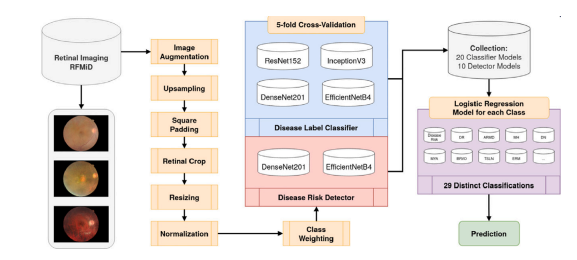
Fig. A.10. Flowchart diagram of the implemented pipeline from Müller et al. for retinal multi-disease detection.
图 A.10. Müller 等人提出的视网膜多疾病检测实现流程图。
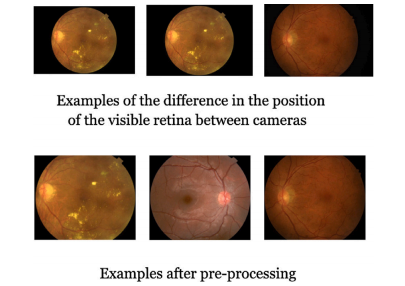
Fig. A.11. Example of fundus images and results of pre-processing on them.
图 A.11. 眼底图像示例及其预处理结果。
Table
表

Table 1The details of the camera model, field of view (FOV), resolution, and number of images included in thedataset.
Table 1: 数据集相机模型、视场(FOV)、分辨率和包含的图像数量的详细信息
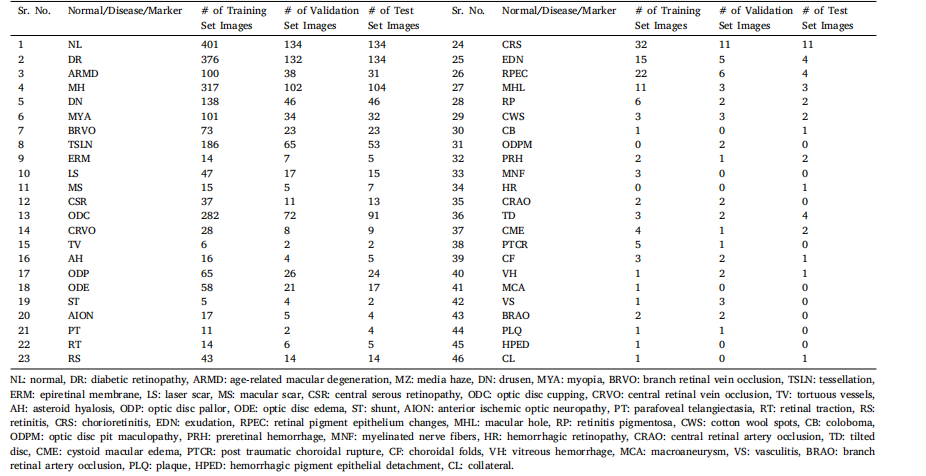
Table 2Summary of data augmentation, normalization and pre-processing in the competing solutions. Where, RF, RR, RS, RT, RC represent random flip, rotation, scaling, translation andcrop respectively.
表2 各参赛解决方案中数据增强、归一化和预处理的总结。其中,RF、RR、RS、RT 和 RC 分别代表随机翻转、旋转、缩放、平移和裁剪。
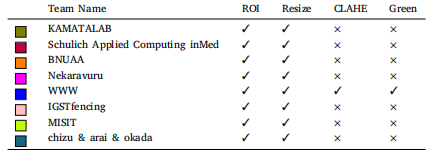
Table 3Summary of data pre-processing used by all the top performing teams. Where ROI isextraction which cuts out the redundant black edge from fundus images; Resize is if theimages are resized before giving to the network; CLAHE is if contrast limited adaptivehistogram equalization (CLAHE) is applied to images; Green is if the green channel isextracted and given to the network.
表 3 顶尖团队使用的数据预处理总结。其中,ROI 指提取视网膜图像的感兴趣区域,去除多余的黑边;Resize 表示是否在输入网络之前对图像进行了调整大小;CLAHE 表示是否对图像应用了对比度受限的自适应直方图均衡化;Green 表示是否提取了绿色通道并输入到网络中。
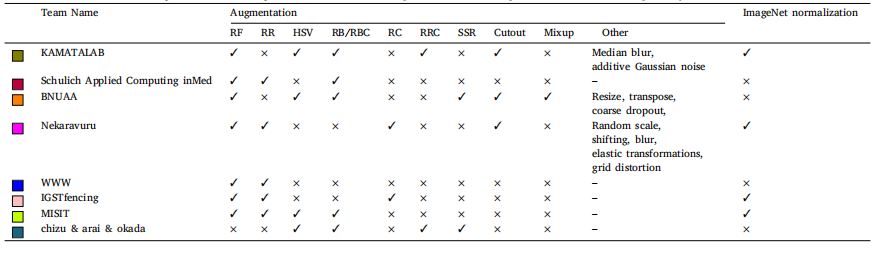
Table 4Summary of data augmentation and normalization used by all the top performing teams. Where RF, RR, HSV, RB/RBC, RC, RRC, and SSR represents random flip, random rotation,hue saturation value, random brightness/ random brightness contrast, random crop, random resized crop, and shift scale rotate respectively
表 4 顶尖团队使用的数据增强和归一化方法总结。其中,RF、RR、HSV、RB/RBC、RC、RRC 和 SSR 分别代表随机翻转、随机旋转、色相-饱和度-亮度(Hue Saturation Value)、随机亮度/随机亮度对比、随机裁剪、随机调整大小裁剪以及移动-缩放-旋转。
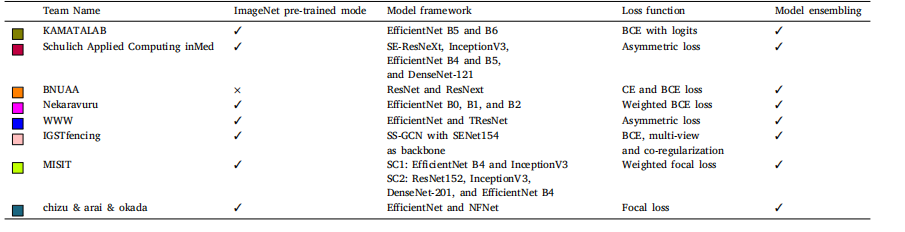
Table 5Summary of pre-training model used and deep learning model with loss functions and model ensembling. Where BCE is binary cross-entropy, CE is cross-entropy, SC1 is sub-challenge1, and SC2 is sub-challenge 2
表 5 预训练模型、深度学习模型及其损失函数与模型集成方法总结。其中,BCE 表示二元交叉熵(Binary Cross-Entropy),CE 表示交叉熵(Cross-Entropy),SC1 表示子挑战 1,SC2 表示子挑战 2。
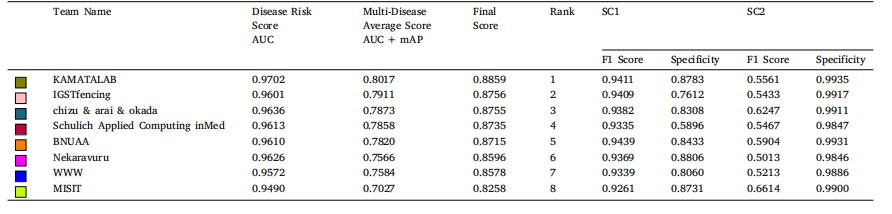
Table 6The best submission performance of top 8 teams in sub-challenge 1 (Disease Risk Score) and sub-challenge 2 (Multi-Disease Average Score) on validation set. AUC: area under thereceiver operator curve, mAP: mean average precision, SC1: sub-challenge-1 and SC2: sub-challenge-2. Average valuesare reported for SC2.
表 6 前 8 支团队在验证集上子挑战 1(疾病风险评分)和子挑战 2(多疾病平均评分)的最佳提交表现。AUC 表示接收者操作特征曲线下面积(Area Under the Receiver Operator Curve),mAP 表示平均精度均值(Mean Average Precision),SC1 表示子挑战 1,SC2 表示子挑战 2。子挑战 2 的结果以平均值报告。
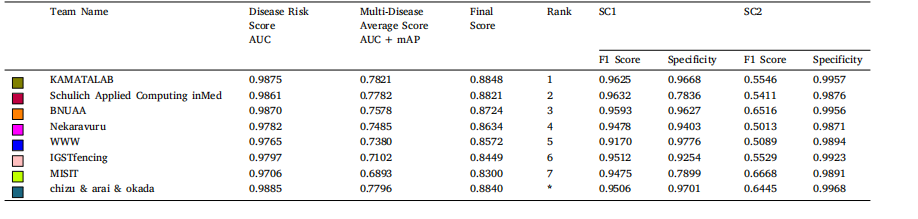
Table 7The performance of top 8 teams in sub-challenge 1 (Disease Risk Score) and sub-challenge 2 (Multi-Disease Average Score) on test set. AUC: area under the receiver operatorcurve, mAP: mean average precision, SC1: sub-challenge-1 and SC2: sub-challenge-2. Average values are reported for SC2.
表 7 前 8 支团队在测试集上子挑战 1(疾病风险评分)和子挑战 2(多疾病平均评分)的表现。AUC 表示接收者操作特征曲线下面积(Area Under the Receiver Operator Curve),mAP 表示平均精度均值(Mean Average Precision),SC1 表示子挑战 1,SC2 表示子挑战 2。子挑战 2 的结果以平均值报告。
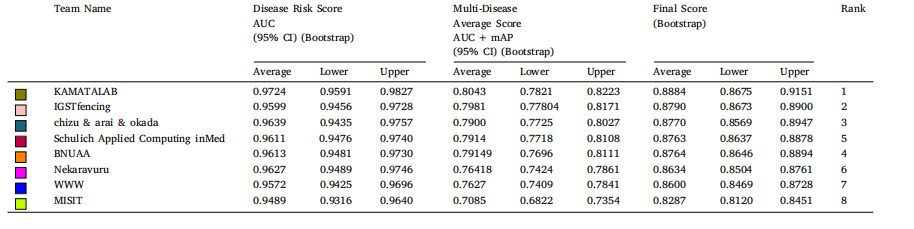
Table 8Bootstrap confidence intervals for best submission performance of top 8 teams in sub-challenge 1 (Disease Risk Score) and sub-challenge 2 (Multi-Disease Average Score) onvalidation set. AUC: area under the receiver operator curve, mAP: mean average precision, CI: confidence interval.
表 8 前 8 支团队在验证集上子挑战 1(疾病风险评分)和子挑战 2(多疾病平均评分)的最佳提交表现的自举置信区间。AUC 表示接收者操作特征曲线下面积(Area Under the Receiver Operator Curve),mAP 表示平均精度均值(Mean Average Precision),CI 表示置信区间(Confidence Interval)。
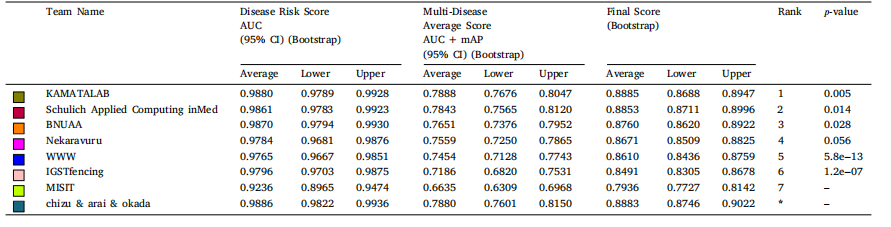
Table 9Bootstrap confidence intervals of top 8 teams in sub-challenge 1 (Disease Risk Score) and sub-challenge 2 (Multi- Disease Average Score) on test set. AUC: area under the receiveroperator curve, mAP: mean average precision, CI: confidence interval, 𝑝-value: performance of Wilcoxon signed-rank tests.
表 9 前 8 支团队在测试集上子挑战 1(疾病风险评分)和子挑战 2(多疾病平均评分)的自举置信区间。AUC 表示接收者操作特征曲线下面积(Area Under the Receiver Operator Curve),mAP 表示平均精度均值(Mean Average Precision),CI 表示置信区间(Confidence Interval),p-值表示 Wilcoxon 符号秩检验的性能。

Table 10The performance of Averaging Ensemble and Weighted Averaging Ensemble for the top-performing methods on test set. AUC: area under the receiveroperator curve, mAP: mean average precision, M1: KAMATALAB, M2: Schulich Applied Computing inMed, M3: BNUAA.
表 10 测试集上顶尖方法的平均集成和加权平均集成的表现。AUC 表示接收者操作特征曲线下面积(Area Under the Receiver Operator Curve),mAP 表示平均精度均值(Mean Average Precision),M1 表示 KAMATALAB,M2 表示 Schulich Applied Computing in Med,M3 表示 BNUAA。
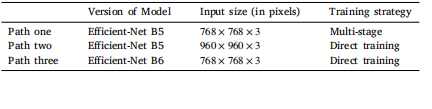
Table A.11The details of three paths with various image sizes, version of models, and trainingstrategies.
表 A.11 三种路径的详细信息,包括不同的图像尺寸、模型版本和训练策略。
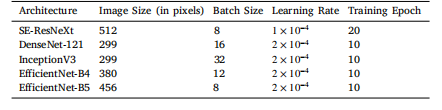
Table A.12Hyperparameters and image input sizes for the 5 different architectures
表 A.12 五种不同架构的超参数和图像输入尺寸。