Title
题目
Labeled-to-unlabeled distribution alignment for partially-supervised multi-organ medical image segmentation
部分监督的多器官医学图像分割中的标注与未标注分布对齐
01
文献速递介绍
多器官医学图像分割(Mo-MedISeg)是医学图像分析领域的一个基础性但具有挑战性的研究任务。它涉及将每个图像像素分配一个语义器官标签,如“肝脏”、“肾脏”、“脾脏”或“胰腺”。任何未定义的器官区域被视为“背景”类别(Cerrolaza等,2019)。随着卷积神经网络(CNNs)(He等,2016)和视觉变换器(Vision Transformers)(Dosovitskiy等,2020;Zhang等,2020b,2024)等深度图像处理技术的发展,Mo-MedISeg已获得显著的研究关注,并广泛应用于各种实际应用中,包括诊断干预(Kumar等,2019)、治疗规划(Chu等,2013),以及计算机断层扫描(CT)图像(Gibson等,2018)和X射线图像(Gómez等,2020)等多种场景。然而,训练一个完全监督的深度语义图像分割模型具有挑战性,因为它通常需要大量像素级标注样本(Wei等,2016;Zhang等,2020a)。对于Mo-MedISeg任务来说,这一挑战更加艰巨,因为获取准确且密集的多器官标注既繁琐又耗时,并且需要稀缺的专家知识。因此,大多数基准公开数据集,如LiTS(Bilic等,2023)和KiTS(Heller等,2019),仅提供单个器官的标注,其他与任务无关的器官被标记为背景。与完全标注的多器官数据集相比,部分标注的多器官数据集更为容易获得。
为了减轻收集完整标注的负担,部分监督学习(PSL)被用来从多个部分标注的数据集中学习Mo-MedISeg模型(Zhou等,2019;Zhang等,2021a;Liu和Zheng,2022)。在这种设置下,每个数据集为单个器官类提供标签,直到涵盖所有感兴趣的前景类别。这种策略避免了需要一个密集标注的数据集,并且可以合并来自不同机构、标注不同器官类型的数据集,尤其是当不同医院关注不同器官时。使用PSL进行Mo-MedISeg的一大挑战是如何在不复杂化多器官分割模型的情况下,利用有限的标注像素和大量未标注像素。每个部分标注的数据集使用二进制图来标注特定器官,指示像素是否属于感兴趣的器官。其他前景器官和背景类别没有提供标签,导致训练集包括每个图像中的标注和未标注像素。先前的方法通常仅从标注像素中学习(Dmitriev和Kaufman,2019;Zhou等,2019;Zhang等,2021a)。
Aastract
摘要
Partially-supervised multi-organ medical image segmentation aims to develop a unified semantic segmentation model by utilizing multiple partially-labeled datasets, with each dataset providing labels for a single class of organs. However, the limited availability of labeled foreground organs and the absence of supervision to distinguish unlabeled foreground organs from the background pose a significant challenge, which leads to a distribution mismatch between labeled and unlabeled pixels. Although existing pseudo-labeling methods can be employed to learn from both labeled and unlabeled pixels, they are prone to performance degradation in this task, as they rely on the assumption that labeled and unlabeled pixels have the same distribution. In this paper, to address the problem of distribution mismatch, we propose a labeled-to-unlabeled distribution alignment (LTUDA) framework that aligns feature distributions and enhances discriminative capability. Specifically, we introduce a cross-set data augmentation strategy, which performs region-level mixing between labeled and unlabeled organs to reduce distribution discrepancy and enrich the training set. Besides, we propose a prototype-based distribution alignment method that implicitly reduces intra-class variation and increases the separation between the unlabeled foreground and background. This can be achieved by encouraging consistency between the outputs of two prototype classifiers and a linear classifier. Extensive experimental results on the AbdomenCT-1K dataset and a union of four benchmark datasets (including LiTS, MSD-Spleen, KiTS, and NIH82) demonstrate that our method outperforms the state-of-the-art partially-supervised methods by a considerable margin, and even surpasses the fully-supervised methods.
部分监督的多器官医学图像分割旨在利用多个部分标注的数据集开发一个统一的语义分割模型,每个数据集为单一类器官提供标签。然而,标注前景器官的数量有限,并且缺乏监督来区分未标注的前景器官和背景,这导致了标注和未标注像素之间的分布不匹配问题。尽管现有的伪标签方法可以用于从标注和未标注像素中学习,但它们在此任务中容易出现性能下降,因为它们依赖于标注和未标注像素具有相同分布的假设。为了解决分布不匹配的问题,本文提出了一种标注到未标注分布对齐(LTUDA)框架,通过对齐特征分布并增强判别能力来克服这一挑战。具体来说,我们提出了一种跨集数据增强策略,该策略通过在标注和未标注器官之间进行区域级混合,以减少分布差异并丰富训练集。此外,我们还提出了一种基于原型的分布对齐方法,通过鼓励两个原型分类器和一个线性分类器之间的输出一致性,隐式地减少类内变异并增加未标注前景和背景之间的分离度。我们在AbdomenCT-1K数据集和四个基准数据集的联合数据集(包括LiTS、MSD-Spleen、KiTS和NIH82)上的大量实验结果表明,我们的方法显著超越了现有的部分监督方法,甚至超越了完全监督方法。
Method
方法
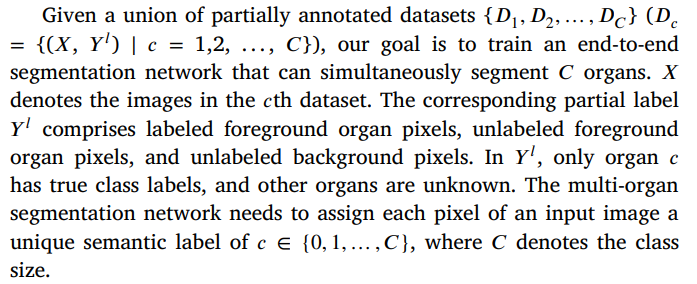
给定一个部分标注数据集的集合 {��1, ��2, …, ����}(���� = {(��, �� ��) ∣ �� = 1,2, …, ��}),我们的目标是训练一个端到端的分割网络,可以同时分割 �� 个器官。这里,�� 表示第 �� 个数据集中的图像。对应的部分标注 �� �� 包括标注的前景器官像素、未标注的前景器官像素和未标注的背景像素。在 �� �� 中,只有器官 �� 拥有真实的类别标签,而其他器官的类别未知。
多器官分割网络需要为输入图像的每个像素分配一个唯一的语义标签 �� ∈ {0, 1, …, ��},其中 �� 表示类别的总数。
Conclusion
结论
Partially-supervised segmentation is confronted with the challenge of feature distribution mismatch between the labeled and unlabeled pixels. To address this issue, this paper proposes a new framework called LTUDA for partially supervised multi-organ segmentation. Our approach comprises two key components. Firstly, we design a crossset data augmentation strategy that generates interpolated samples between the labeled and unlabeled pixels, thereby reducing feature discrepancy. Furthermore, we propose a prototype-based distribution alignment module to align the distributions of labeled and unlabeled pixels. This module eliminates confusion between unlabeled foreground and background by encouraging consistency between labeled prototype and unlabeled prototype classifier. Experimental results on both a toy dataset and a large-scale partially labeled dataset demonstrate the effectiveness of our method. Our proposed method has the potential to contribute to the development of foundation models. Recent foundation models (Liu et al., 2023;Ye et al., 2023) have focused on leveraging large-scale and diverse partially annotated datasets to segment different types of organs and tumors, thereby fully exploiting existing publicly available datasets from different modalities (e.g., CT, MRI, PET). While these models have achieved promising results, they typically do not leverage unlabeled pixels or consider the domain shift between partially-labeled datasets from different institutions. This heterogeneity in training data leads to a more pronounced distributional deviation between labeled and unlabeled pixels, posing a greater challenge. Our proposed labeled-tounlabeled distribution framework specifically addresses this challenge and thus can help unlock the potential of public datasets. Looking ahead, one promising future direction is to apply partially supervised segmentation methods to more realistic clinical scenarios (Yao et al.,2021). Our approach demonstrates the feasibility of training multiorgan segmentation models using small-sized partially labeled datasets. This convenience enables the customization and development of multiorgan segmentation models based on the clinical requirements of medical professionals. If the annotations provided by the public datasetslack specific anatomical structures of interest, experts would only need to provide annotations of a small number of missing classes to develop multi-organ segmentation models.
部分监督分割面临标注和未标注像素之间的特征分布不匹配问题。为了解决这一问题,本文提出了一种新的框架,称为LTUDA,用于部分监督的多器官分割。我们的方法包括两个关键组件。首先,我们设计了一种跨集数据增强策略,通过在标注和未标注像素之间生成插值样本,从而减少特征差异。其次,我们提出了一个基于原型的分布对齐模块,用于对标注和未标注像素的分布进行对齐。该模块通过鼓励标注原型和未标注原型分类器之间的一致性,消除了未标注前景和背景之间的混淆。通过在玩具数据集和大规模部分标注数据集上的实验结果验证了我们方法的有效性。
我们提出的方法有潜力为基础模型的发展做出贡献。最近的基础模型(Liu et al., 2023; Ye et al., 2023)专注于利用大规模且多样化的部分标注数据集,分割不同类型的器官和肿瘤,从而充分利用现有的公开数据集(如CT、MRI、PET)。尽管这些模型取得了令人鼓舞的结果,但它们通常没有利用未标注像素,也没有考虑来自不同机构的部分标注数据集之间的领域偏移。训练数据中的这种异质性导致标注和未标注像素之间的分布偏差更加明显,提出了更大的挑战。我们提出的标注到未标注分布对齐框架专门解决了这个挑战,因此可以帮助充分挖掘公共数据集的潜力。
展望未来,一个有前景的方向是将部分监督分割方法应用于更为现实的临床场景(Yao et al., 2021)。我们的方法展示了使用小规模部分标注数据集训练多器官分割模型的可行性。这种便利性使得可以根据医学专业人员的临床需求定制和开发多器官分割模型。如果公共数据集中提供的标注缺少特定的解剖结构,专家只需提供少量缺失类别的标注即可开发多器官分割模型。
Figure
图
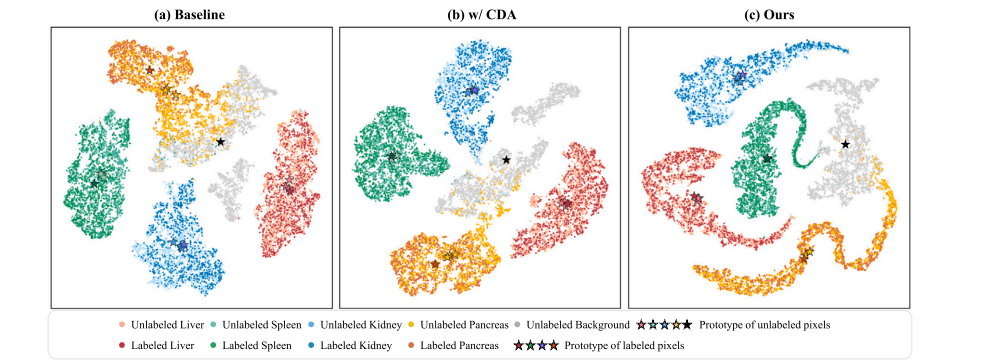
Fig. 1. Comparisons of t-SNE feature visualization on the toy dataset consisting of four partially-labeled sub-datasets. The feature distribution of labeled and unlabeled pixels for different classes is visualized. For each foreground category, only one sub-dataset provides a labeled set, while the other three provide unlabeled sets. Since each sub-dataset does not provide the true label of the background, the background is completely unlabeled. We have superimposed the feature centers of the labeled set and unlabeled set, i.e., labeled prototypes and unlabeled prototypes, of each foreground category on the feature distribution. Additionally, we visualized the feature center of the background classes across all subsets. (a) Baseline model (trained on labeled pixels). The labeled prototype and unlabeled prototypes of the foreground classes are not aligned. (b) Baseline model with cross-set data augmentation (CDA). The CDA strategy effectively reduces the distributional discrepancy between labeled and unlabeled pixels for the foreground classes. © Our proposed method. The labeled prototype and unlabeled prototypes of each foreground class almost overlap.
图1. 在由四个部分标注子数据集组成的玩具数据集上进行t-SNE特征可视化比较。展示了不同类别的标注像素和未标注像素的特征分布。对于每个前景类别,只有一个子数据集提供标注集,而其他三个子数据集提供未标注集。由于每个子数据集未提供背景的真实标签,因此背景完全未标注。我们在特征分布上叠加了每个前景类别的标注集和未标注集的特征中心,即标注原型和未标注原型。此外,我们还可视化了所有子集中的背景类的特征中心。(a)基线模型(仅在标注像素上训练)。前景类别的标注原型和未标注原型未对齐。(b)带有跨集数据增强(CDA)的基线模型。CDA策略有效地减少了前景类别标注和未标注像素之间的分布差异。(c)我们提出的方法。每个前景类别的标注原型和未标注原型几乎重叠。
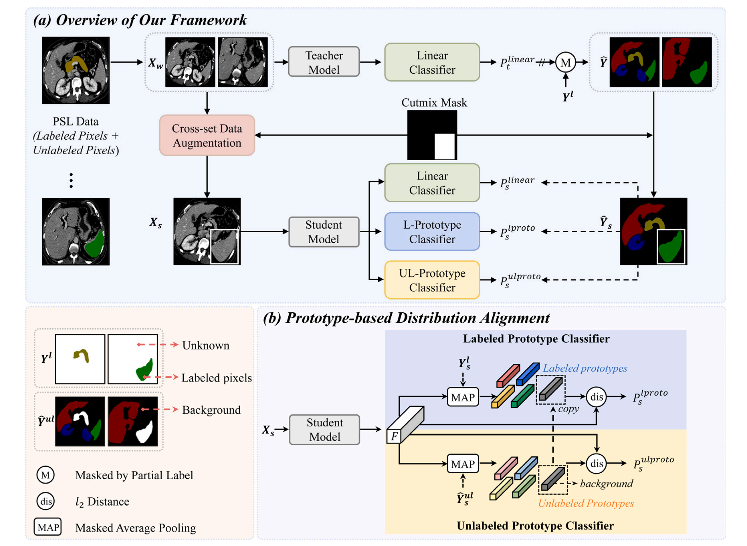
Fig. 2. (a) The overall framework of the proposed LTUDA method, which consists of cross-set data augmentation and prototype-based distribution alignment. (b) Details of the prototype-based distribution alignment module. Our method is built on the popular teacher–student framework and applies weak (rotation and scaling) and strong augmentation (cross-set region-level mixing) to the input images of the teacher and student models, respectively. The linear classifier refers to the linear threshold-based classifier described in Eq. (2) of Section 3.1. Two prototype classifiers are introduced in the student model, and the predictions of the teacher model and partial labels are combined as pseudo-labels to supervise the outputs of the three classifiers in the student model. The term ‘‘copy’’ denotes that the labeled prototype of the background class is set to be equal to the unlabeled prototype
图2. (a) 提出的LTUDA方法的整体框架,包括跨集数据增强和基于原型的分布对齐。(b) 基于原型的分布对齐模块的详细信息。我们的方法基于流行的教师-学生框架,并分别对教师和学生模型的输入图像应用弱增强(旋转和缩放)和强增强(跨集区域级混合)。线性分类器指的是第3.1节公式(2)中描述的基于线性阈值的分类器。在学生模型中引入了两个原型分类器,并将教师模型的预测和部分标签结合为伪标签,监督学生模型中三个分类器的输出。术语“copy”表示背景类别的标注原型被设定为等于未标注原型。
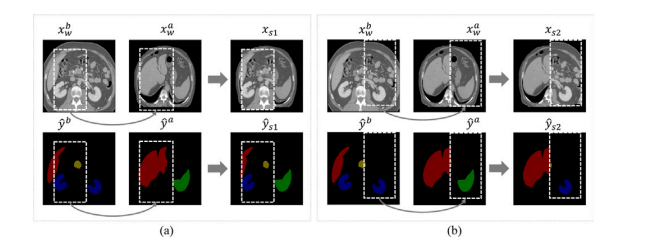

图3. 由CutMix生成的不同强度增强图像的可视化。(a)和(b)将?? ?? ?? 中裁剪的补丁粘贴到?? ?? ?? 中的相同位置。(a)和(b)的框坐标和大小不同。
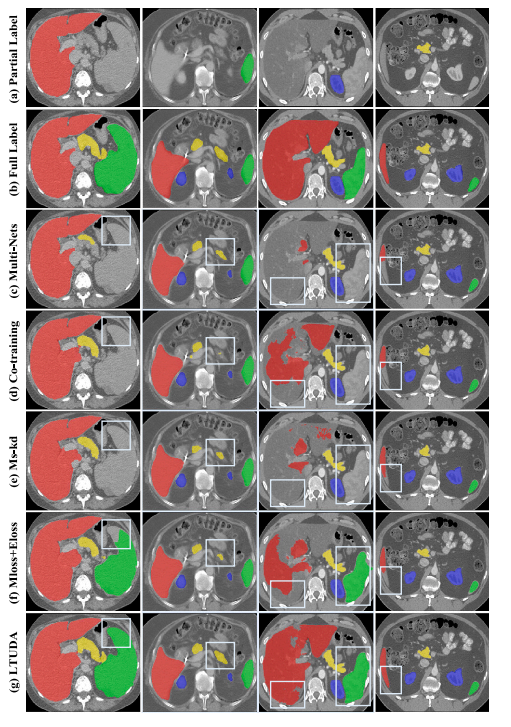
Fig. 4. Visualizations of LSPL. Examples are from the LiTS, MSD-Spleen, KiTS, and NIH82 datasets, respectively, arranged from left to right. (a) Single-organ annotations originally provided by the four benchmark datasets. (b) Full annotations of four organs. © to (g) are the segmentation results of different methods. The white frame highlights the better predictions of our method.
图4. LSPL的可视化。示例来自LiTS、MSD-Spleen、KiTS和NIH82数据集,按从左到右的顺序排列。(a) 四个基准数据集最初提供的单器官标注。(b) 四个器官的完整标注。© 到 (g) 是不同方法的分割结果。白色框突出显示我们方法的更好预测。
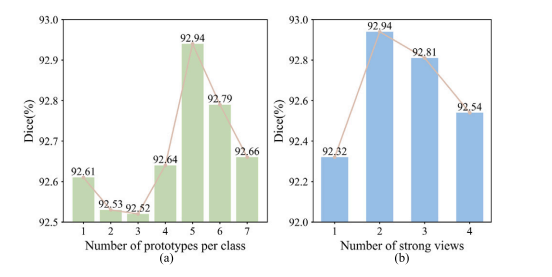
Fig. 5. Ablation results. (a) The number of prototypes per class. (b) The number of strong views.
图5. 消融结果。(a) 每个类别的原型数量。(b) 强增强视图的数量。
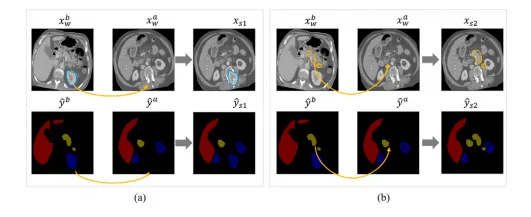
Fig. 6. Visualizations of differently strongly augmented images generated by CarveMix. (a) and (b) paste the cropped ROI from ?? ?? ?? to the same position in ?? ?? ?? . (a) and (b) crop the ROI of different foreground organs.
图6. 由CarveMix生成的不同强度增强图像的可视化。(a)和(b)将来自?? ?? ?? 的裁剪区域(ROI)粘贴到?? ?? ?? 中的相同位置。(a)和(b)裁剪了不同前景器官的ROI。
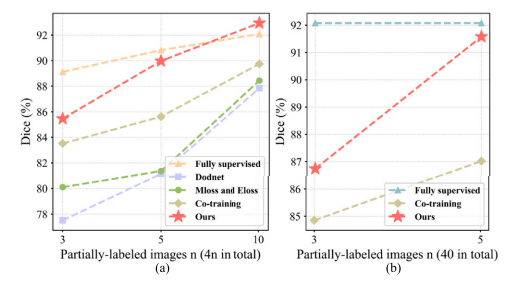
Fig. 7. (a) The performance of learning from partially labeled data under different labeled data sizes. (b) The performance of learning from partially labeled data and unlabeled data under different labeled data sizes.
图7. (a) 在不同标注数据大小下,从部分标注数据中学习的性能。(b) 在不同标注数据大小下,从部分标注数据和未标注数据中学习的性能。
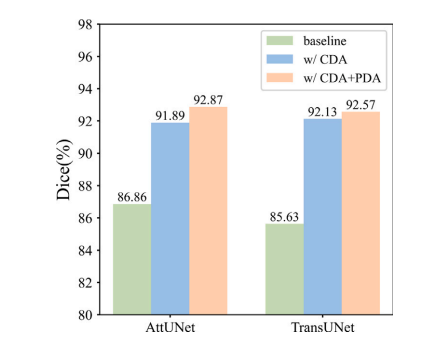
Fig. 8. Ablation results of different segmentation backbones.
图8. 不同分割骨干网络的消融结果。
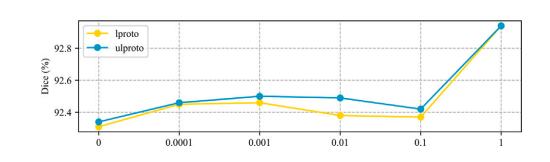

图9. L???????????? 和 L?????????????? 权重参数的消融研究。
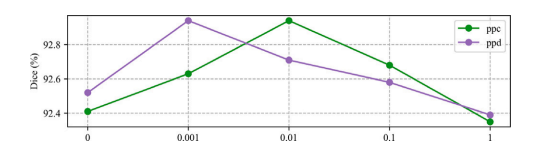

图10. L?????? 和 L?????? 权重参数的消融研究。
Table
表
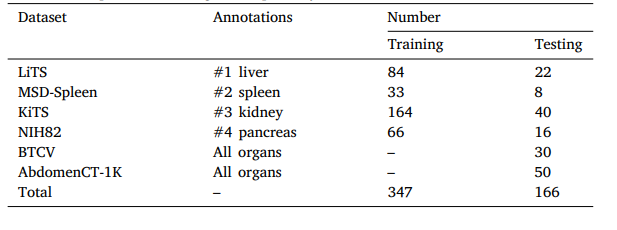
Table 1A brief description of the large-scale partially-labeled dataset.
表1:大规模部分标注数据集的简要描述。
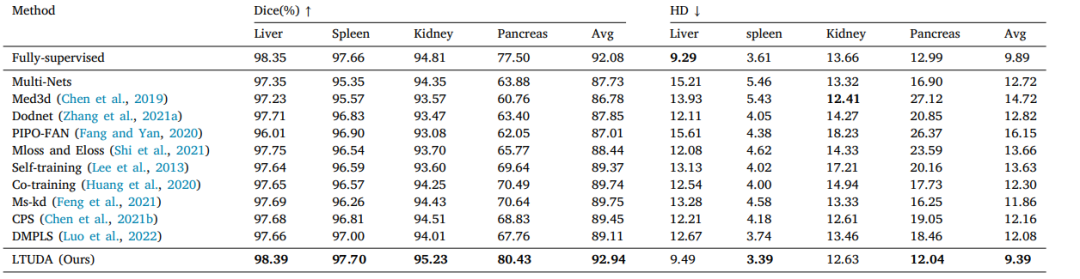
Table 2Quantitative results of partially-supervised multi-organ segmentation on a toy dataset.
表2:在玩具数据集上进行部分监督多器官分割的定量结果。
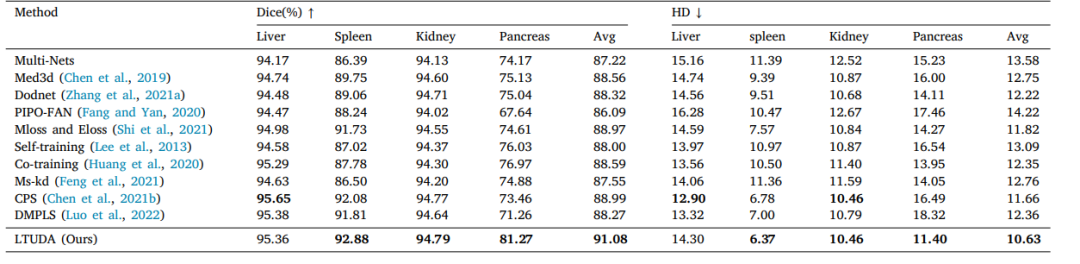
Table 3Quantitative results of partially-supervised multi-organ segmentation on four partially labeled datasets (LSPL dataset).
表3:在四个部分标注数据集(LSPL数据集)上进行部分监督多器官分割的定量结果。
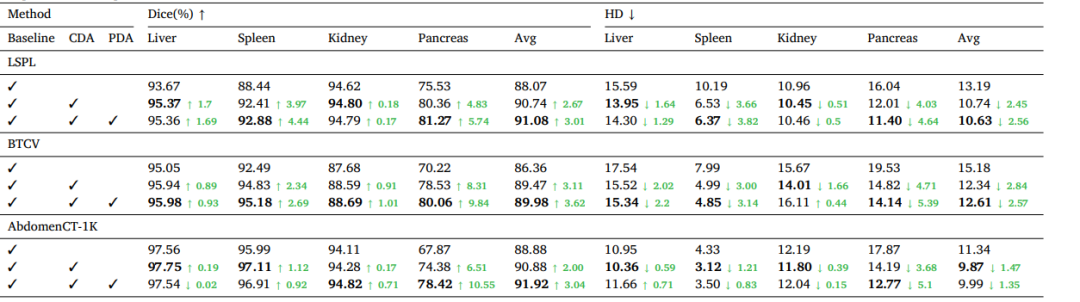
Table 4Ablation study of key components on LSPL dataset. CDA denotes cross-set data augmentation, and PDA denotes prototype-based distribution alignment. Green numbers indicate the performance improvement over the baseline.
表4:在LSPL数据集上关键组件的消融实验。CDA表示跨集数据增强,PDA表示基于原型的分布对齐。绿色数字表示相对于基线的性能提升。
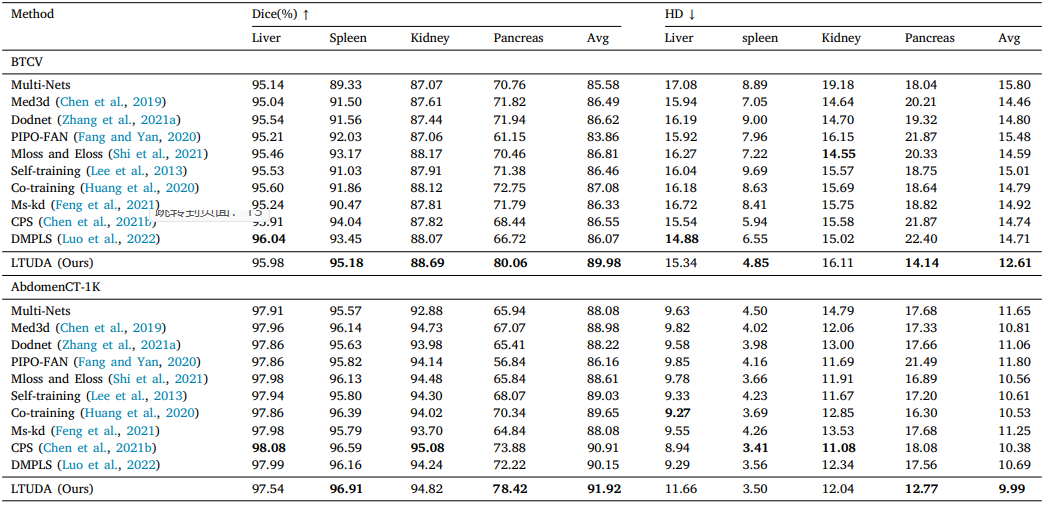
Table 5Generalization performance of different methods on two multi-organ datasets.
表5:不同方法在两个多器官数据集上的泛化性能。

Table 6Ablation study of key components on the toy dataset. CDA denotes cross-set data augmentation, and PDA denotes prototype-based distribution alignment. Green numbers indicate the performance improvement over the baseline.
表6:在玩具数据集上关键组件的消融实验。CDA表示跨集数据增强,PDA表示基于原型的分布对齐。绿色数字表示相对于基线的性能提升。

Table 7Ablation results of different data augmentation methods.
表7:不同数据增强方法的消融结果。

Table 8Comparison of different paste position strategies for CutMix.
表8:不同CutMix粘贴位置策略的比较。

Table 9Intra-class and inter-class variance for different methods.
表9:不同方法的类内和类间方差。