Title
题目
Cross-view discrepancy-dependency network for volumetric medical imagesegmentation
跨视角差异-依赖网络用于体积医学图像分割
01
文献速递介绍
医学图像分割旨在从原始图像中分离出受试者的解剖结构(例如器官和肿瘤),并为每个像素分配语义类别,这在许多临床应用中起着至关重要的作用,如器官建模、疾病诊断和治疗规划(Shamshad 等,2023)。对于三维图像,临床医生需要逐片手动描绘感兴趣区域(VOI),这需要大量的劳动和专业知识(Qureshi 等,2023)。计算机辅助诊断(CAD)系统的目标是帮助临床医生迅速描绘出VOI(Shi 等,2022)。然而,这一任务在稳健性和准确性方面仍然面临挑战。随着CAD系统需求的快速增长,开发稳健且准确的三维医学图像分割算法变得愈加紧迫。
在过去的十年中,深度卷积神经网络(DCNNs)吸引了越来越多的关注,并推动了三维医学图像分割的进展(Xu 等,2023;Liu 等,2023)。通常,构建稳健的DCNNs需要大量的数据。但在许多实际场景中,这些模型往往面临数据稀缺的问题,主要是由于某些疾病的发病率低或获取大规模三维医学图像数据集的成本高昂(Huang 等,2023;Jiao 等,2023)。为了缓解这一问题,许多方法尝试通过基于图像块的策略处理三维图像(Çiçek 等,2016;Milletari 等,2016;Isensee 等,2021)。尽管这种策略可以捕捉局部空间信息,但由于输入的感受野有限,提取长期上下文信息变得困难。作为替代,一些研究提出通过使用从三维图像中提取的多个连续切片来训练网络(Alom 等,2018;McHugh 等,2021)。这些方法将切片图像视为独立样本,并且仅使用单视角切片图像(即轴向平面),但这不可避免地忽略了来自其他两个视角(即冠状平面和矢状平面)的空间信息以及切片之间的连续性(Dong 等,2022)。因此,更为理想的方式是基于多视角切片图像开发分割模型,通过同时考虑多个正交平面来保留全面的空间信息。
Aastract
摘要
The limited data poses a crucial challenge for deep learning-based volumetric medical image segmentation, andmany methods have tried to represent the volume by its subvolumes (i.e., multi-view slices) for alleviating thisissue. However, such methods generally sacrifice inter-slice spatial continuity. Currently, a promising avenueinvolves incorporating multi-view information into the network to enhance volume representation learning, butmost existing studies tend to overlook the discrepancy and dependency across different views, ultimately limiting the potential of multi-view representations. To this end, we propose a cross-view discrepancy-dependencynetwork (CvDd-Net) to task with volumetric medical image segmentation, which exploits multi-view slice priorto assist volume representation learning and explore view discrepancy and view dependency for performanceimprovement. Specifically, we develop a discrepancy-aware morphology reinforcement (DaMR) module toeffectively learn view-specific representation by mining morphological information (i.e., boundary and positionof object). Besides, we design a dependency-aware information aggregation (DaIA) module to adequatelyharness the multi-view slice prior, enhancing individual view representations of the volume and integratingthem based on cross-view dependency. Extensive experiments on four medical image datasets (i.e., Thyroid,Cervix, Pancreas, andGlioma) demonstrate the efficacy of the proposed method on both fully-supervised and semi-supervised tasks.
有限数据对基于深度学习的体积医学图像分割构成了一个重要挑战,许多方法尝试通过其子体积(即多视图切片)来表示体积,以缓解这一问题。然而,这些方法通常牺牲了切片间的空间连续性。目前,一个有前景的方向是将多视图信息引入网络,以增强体积表示学习,但大多数现有研究往往忽视了不同视图之间的差异性和依赖性,最终限制了多视图表示的潜力。为此,我们提出了一个跨视图差异-依赖网络(CvDd-Net),用于体积医学图像分割,它利用多视图切片先验来辅助体积表示学习,并探索视图差异和视图依赖性,以提高性能。具体来说,我们开发了一个差异感知形态强化(DaMR)模块,通过挖掘形态学信息(即边界和物体的位置)来有效地学习视图特定的表示。此外,我们设计了一个依赖感知信息聚合(DaIA)模块,充分利用多视图切片先验,增强体积的个体视图表示,并基于跨视图依赖性进行整合。在四个医学图像数据集(即甲状腺、宫颈、胰腺和胶质瘤)上的广泛实验展示了所提方法在全监督和半监督任务中的有效性。
Conclusion
结论
In this work, we propose a cross-view discrepancy-dependency network (CvDd-Net) to task with volumetric medical image segmentation,which utilizes multi-view slice prior to assist volume representationlearning and explore view discrepancy and view dependency for performance improvement. Specifically, we introduce a discrepancy-awaremorphology reinforcement (DaMR) module to exploit view discrepancyby modeling morphology information (i.e., boundary and position ofobject) for learning view-specific representation. Besides, we develop adependency-aware information aggregation (DaIA) module to exploreview dependency for aggregating multi-view information after integrating prior knowledge from slices. Extensive experiments on fourdatasets demonstrate the effectiveness of our proposed method withrelatively small size of model, especially on the small target, indicatingthe effectiveness of multi-view slice prior on assisting volume representation learning. Meanwhile, our CvDd-Net reveals higher performancewhen compared with semi-supervised methods, which also testifies thepotential of multi-view learning in the application of limited data.
在本研究中,我们提出了一种用于体积医学图像分割的跨视角差异依赖网络(CvDd-Net),该方法利用多视角切片作为先验知识来辅助体积表示学习,并通过探索视角差异和视角依赖关系来提高性能。具体而言,我们引入了一种差异感知形态强化(DaMR)模块,通过建模形态信息(即物体的边界和位置)来挖掘视角差异,从而学习视角特定的表示。此外,我们开发了一种依赖感知信息聚合(DaIA)模块,在整合切片先验知识后,通过聚合多视角信息来探索视角依赖关系。针对四个数据集的广泛实验表明,我们提出的方法在相对较小的模型规模下取得了良好的效果,特别是在小目标的分割任务中,表明多视角切片先验在辅助体积表示学习中的有效性。同时,我们的CvDd-Net在与半监督方法的比较中表现出更高的性能,这也证明了多视角学习在有限数据应用中的潜力。CRediT authorship contribution stat
Figure
图
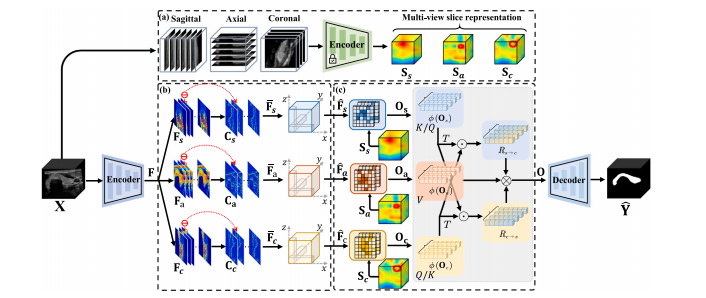
Fig. 1. The flowchart of CvDd-Net. It consists of an encoder and a decoder (color in blue) for feature extraction and prediction map generation. Besides, it also comprises: (a) aslice-based encoder (color in green) for multi-view slice representation learning, (b) a discrepancy-aware morphology reinforcement (DaMR) module for view-specific representationlearning, and © a dependency-aware information aggregation (DaIA) module for multi-view representation fusion.
图1. CvDd-Net的流程图。它由一个编码器和一个解码器(蓝色部分)组成,用于特征提取和预测图生成。此外,它还包括:(a)一个基于切片的编码器(绿色部分),用于多视角切片表示学习,(b)一个差异感知形态学增强(DaMR)模块,用于视角特定表示学习,以及(c)一个依赖感知信息聚合(DaIA)模块,用于多视角表示融合。
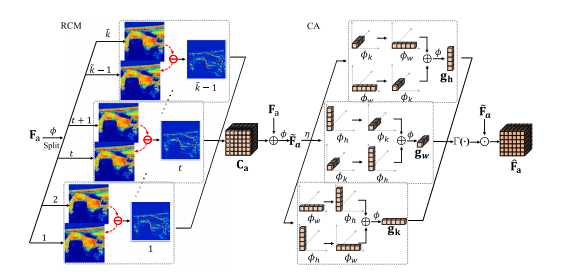
Fig. 2. Illustration of discrepancy-aware morphology reinforcement module. RCM:residual context mapping; CA: coordinate attention.
图2. 差异感知形态学增强模块的示意图。RCM:残差上下文映射;CA:坐标注意力。
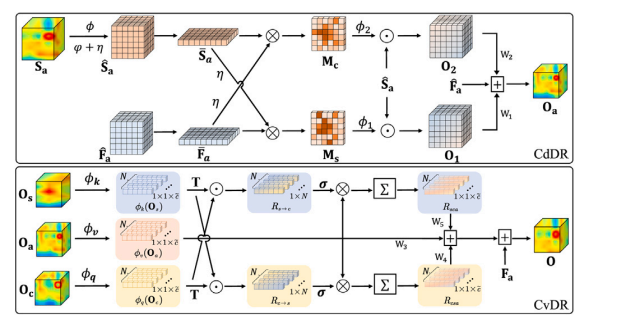
Fig. 3. Illustration of dependency-aware information aggregation module. CdDR:cross-dimension dependency-aware relation; CvDR: cross-view dependency-awarerelation
图3. 依赖感知信息聚合模块的示意图。CdDR:跨维度依赖感知关系;CvDR:跨视角依赖感知关系。
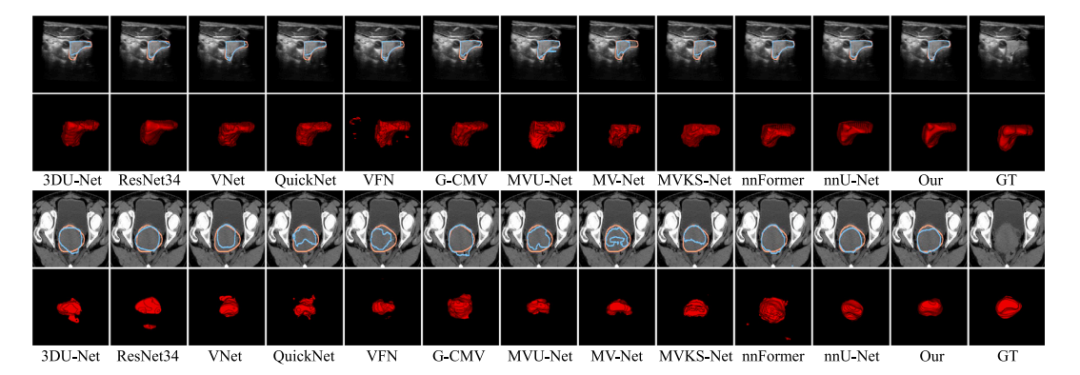
Fig. 4. Visualization results of all competing methods on some representative cases from Thyroid (top) and Cervix (bottom) datasets. The blue and orange lines in the first andthird rows represent the boundary of prediction map and ground-truth. The final column named as GT denotes the original image and its ground-truth
图4 各竞争方法在甲状腺(上)和子宫颈(下)数据集的代表性病例上的可视化结果。第一行和第三行中的蓝色和橙色线分别表示预测图和真实标注的边界。最后一列标为GT,表示原始图像及其真实标注。
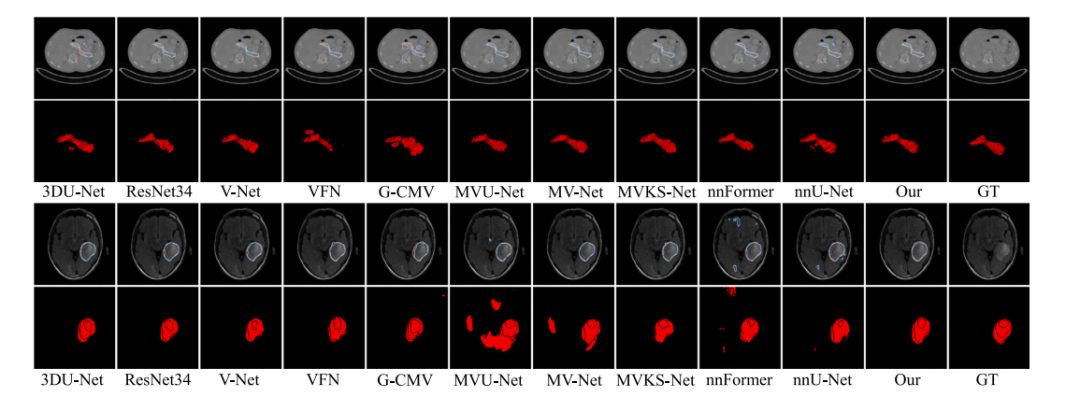
Fig. 5. Visualization results of all competing methods on some representative cases from Pancreas (top) and Glioma (bottom) datasets. The blue and orange lines in the first and third rows represent the boundary of prediction map and ground-truth. The final column named as GT denotes the original image and its ground-truth.
图5 各竞争方法在胰腺(上)和胶质瘤(下)数据集的代表性病例上的可视化结果。第一行和第三行中的蓝色和橙色线分别表示预测图和真实标注的边界。最后一列标为GT,表示原始图像及其真实标注。
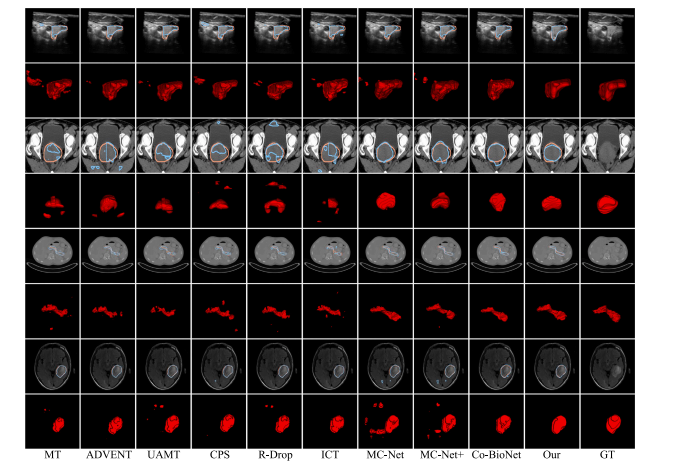
Fig. 6. Qualitative comparison of all semi-supervised methods on some representative cases from Thyroid, Cervix, Pancreas, and Glioma (from top to bottom) datasets. Thesegmentation results are from the models trained with 50% data. The blue and orange lines represent the boundary of predication and ground-truth. The final column named asGT denotes the original image and its ground-truth
图6 所有半监督方法在一些代表性病例上的定性比较,来自甲状腺、宫颈、胰腺和胶质瘤(从上到下)数据集。分割结果来自使用50%数据训练的模型。蓝色和橙色线条表示预测结果和真实标签的边界。最后一列名为GT,表示原始图像及其真实标签。
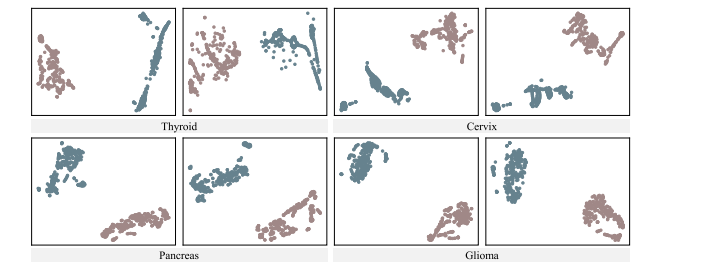
Fig. 7. Visualization of t-SNE from four datasets for validating the representation learning intuition of CvDd-Net
图7 四个数据集的t-SNE可视化,用于验证CvDd-Net的表示学习直觉。
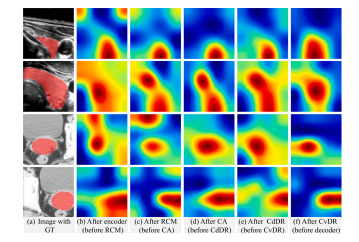
Fig. 8. Visualization of feature maps from intermediate layer of CvDd-Net. From leftto right are (a) original image with GT, feature maps (b) after encoder (before RCM),© after RCM (before CA), (d) after CA (before CdDR), (e) after CdDR (before CvDR),and (f) after CvDR (before decoder), respectively
图8 CvDd-Net中间层特征图的可视化。从左到右分别是:(a) 带有GT的原始图像,(b) 编码器后的特征图(RCM之前),© RCM后的特征图(CA之前),(d) CA后的特征图(CdDR之前),(e) CdDR后的特征图(CvDR之前),(f) CvDR后的特征图(解码器之前)。
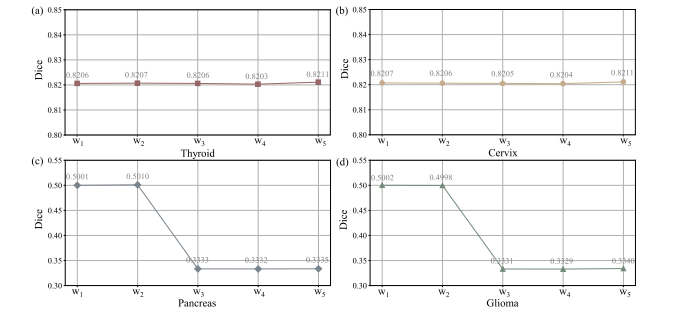
Fig. 9. Qualitative results of adaptive weights (w1 and w2 in CdDR, and w3 , w4 , and w5 in CvDR) on different datasets
图9 自适应权重(CdDR中的w1和w2,CvDR中的w3、w4和w5)在不同数据集上的定性结果。
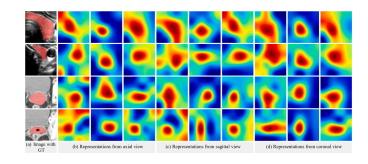
Fig. 10. Visualization of feature maps from intermediate layer of CvDd-Net that trainedwith 25% data. From left to right are (a) Image with GT, Representations from (b) axialview, © sagittal view, and (d) coronal view, respectively. Specifically, features shownthree columns from different views (from left to right) are: (1) slice representation, (2)feature map after CA (before CdDR), and (3) feature map after CdDR (before CvDR),respectively
图10 CvDd-Net使用25%数据训练后的中间层特征图可视化。从左到右分别为:(a) 带有GT的图像,表示来自 (b) 轴向视图,© 矢状视图和 (d) 冠状视图的特征。具体来说,三列不同视图的特征展示如下:(1)切片表示,(2)CA后的特征图(在CdDR之前),(3)CdDR后的特征图(在CvDR之前)。
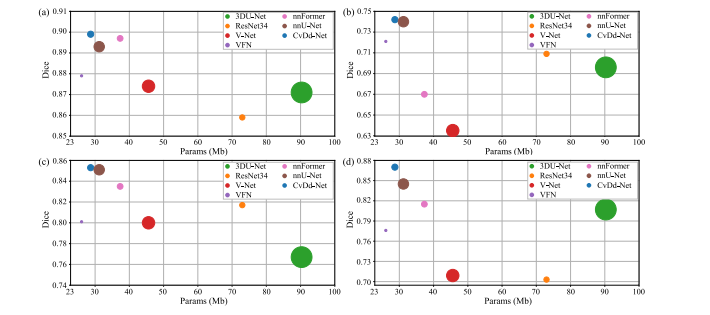
Fig. 11. Complexity analysis of different models on four datasets. The horizontal and vertical axis represent the number of model’s parameters and Dice similarity coefficient, andthe size of circle represents the FLOPs of model.
Fig. 11. 不同模型在四个数据集上的复杂度分析。横轴和纵轴分别表示模型的参数数量和Dice相似系数,圆圈的大小表示模型的FLOPs(浮点运算量)。
Table
表
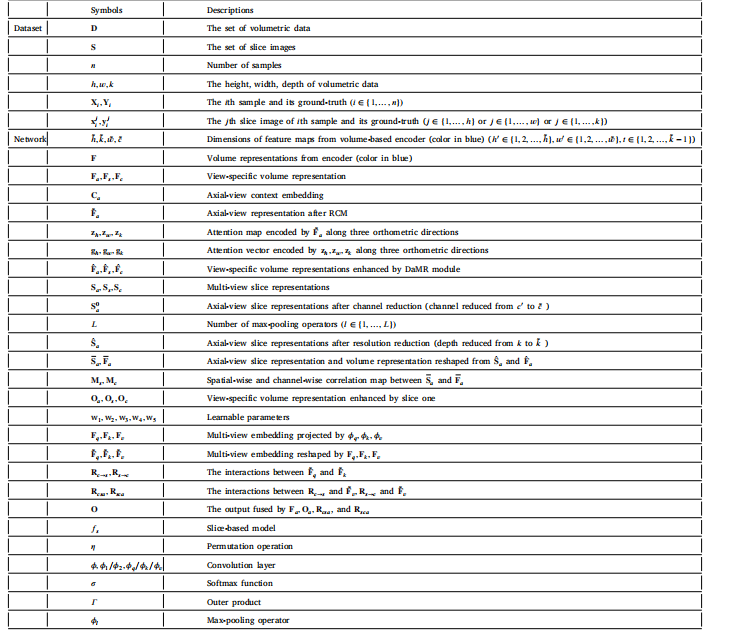
Table 1The list of symbols and their corresponding descriptions.
表1符号及其对应的描述列表。
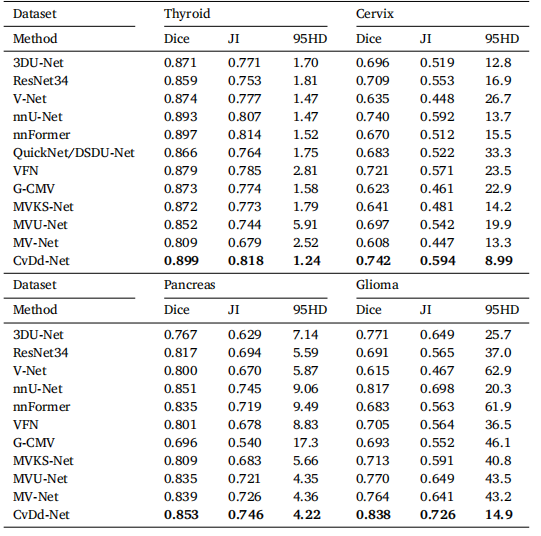
Table 2Segmentation performance of all competing methods on four datasets.
表2四个数据集上所有竞争方法的分割性能。
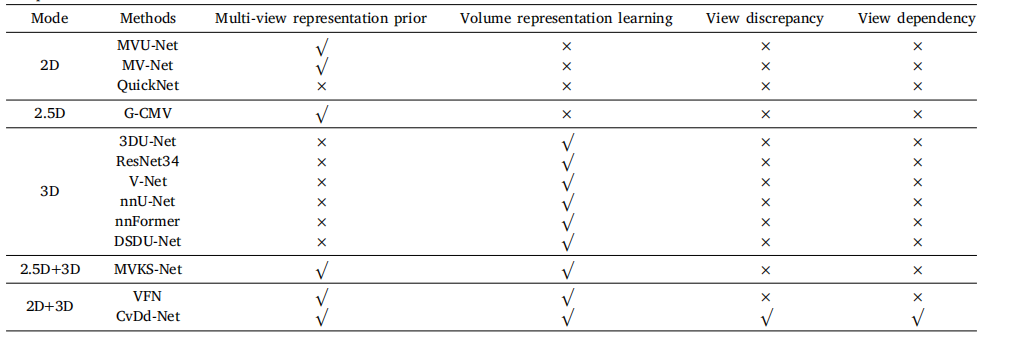
Table 3Comparison between our method and others.
表3我们方法与其他方法的比较。
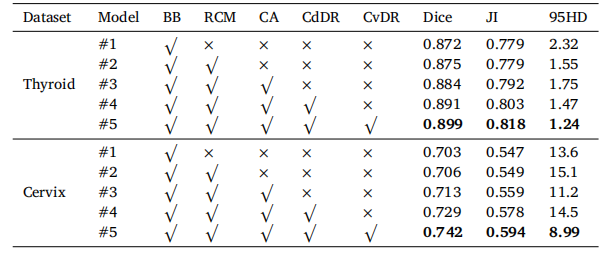
Table 4Segmentation performance of CvDd-Net with different settings, including RCM, CA,CdDR, and CvDR. BB denotes backbone.
表4CvDd-Net在不同设置下的分割性能,包括RCM、CA、CdDR和CvDR。BB表示骨干网络。
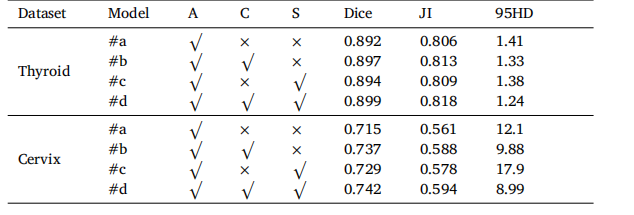
Table 5Segmentation performance of CvDd-Net with different multi-view slice representationon two datasets. ‘A’, ‘C’, and ‘S’ denote axial, coronal, and sagittal views, respectively.
表5CvDd-Net在两个数据集上使用不同的多视图切片表示的分割性能。‘A’,‘C’和‘S’分别表示轴向、冠状面和矢状面视图。
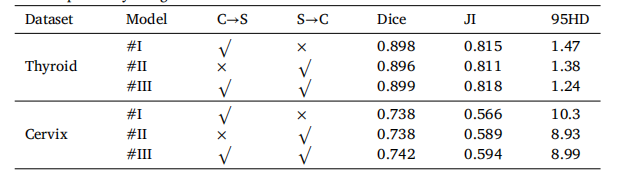
Table 6Segmentation performance of CvDd-Net with different cross-view dependency relationon two datasets. ‘C→S’ and ‘S→C’ represent the two cross-view relations to computeview dependency weights.
表6CvDd-Net在两种数据集上使用不同跨视图依赖关系的分割性能。‘C→S’和‘S→C’表示计算视图依赖权重的两种跨视图关系。
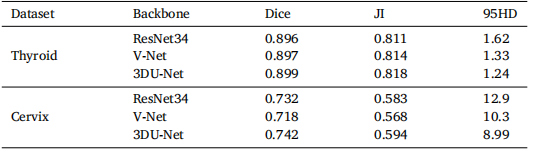
Table 7Segmentation performance of CvDd-Net with different backbones on two datasets.
表7不同后端架构的CvDd-Net在两个数据集上的分割性能。
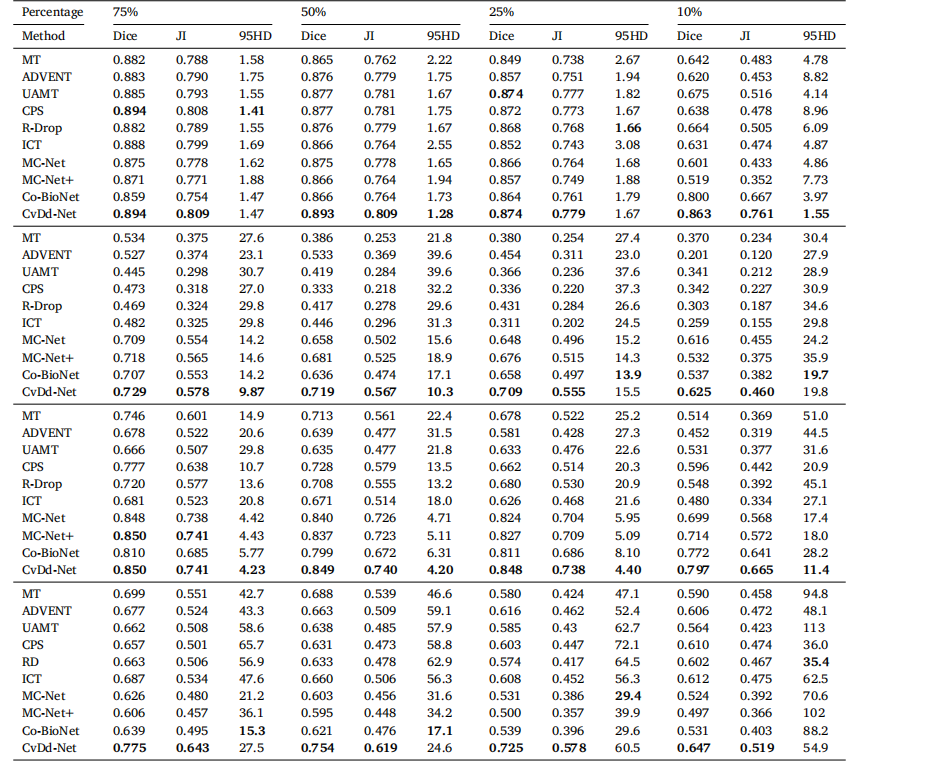
Table 8Segmentation performance of all semi-supervised methods on four datasets.
表8所有半监督方法在四个数据集上的分割性能。
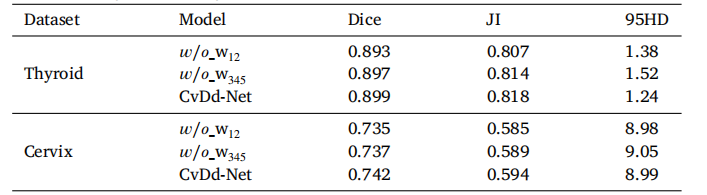
Table 9Segmentation performance of CvDd-Net with different adaptive weights (w1 and w2 inCdDR, and w3 , w4 , and w5 in CvDR) on two datasets.
表9CvDd-Net在两种数据集上使用不同自适应权重(CdDR中的w1和w2,以及CvDR中的w3、w4和w5)的分割性能。
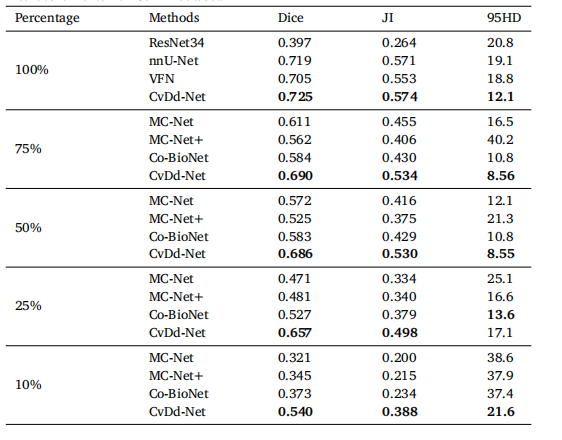
Table 10Segmentation performance of representative fully-supervised and semi-supervisedmethods on external Cervix dataset.
表10代表性全监督和半监督方法在外部宫颈数据集上的分割性能。