Title
题目
OCTA-500: A retinal dataset for optical coherence tomography angiographystudy
OCTA-500:用于光学相干断层扫描血管造影研究的视网膜数据集
01
文献速递介绍
光学相干断层扫描(OCT)是视网膜成像领域最重要的进展之一,能够以微米级分辨率非侵入性地捕捉视网膜的三维结构数据(Huang等, 1991)。OCT广泛用于观察视网膜的横截面结构和监测液体渗漏(Sakata等, 2009)。然而,OCT的局限在于它无法直接提供血流信息。在OCT平台的基础上,OCT血管造影(OCTA)被开发为一种新的成像技术,用于提供视网膜血管和微血管系统的功能信息。
OCTA通过干涉法测量反射或反向散射光的振幅和延迟,以获取视网膜血管造影体积数据(Kashani等, 2017)。OCTA体积数据可以从不同的视网膜层投影,以单独显示相应的视网膜网状结构。这一独特的观察视角提高了我们对视网膜血管病理生理学的理解。自2014年首款商业产品推出以来,OCTA在临床应用中迅速展示了其在年龄相关性黄斑变性、糖尿病视网膜病变、脉络膜新生血管、青光眼及其他眼病中的卓越性(Kashani等, 2017; Spaide等, 2018; Lains等, 2021)。近年来,OCTA还被用于研究神经系统疾病(如阿尔茨海默病、帕金森病和亨廷顿病)中视网膜血流的功能性变化(Jiang等, 2018; Zabel等, 2019; Kwapong等, 2018; Robbins等, 2022; Di Maio等, 2021)。
视网膜血管的OCTA定量分析对于标准化临床结果的客观解释至关重要(Yao等, 2020)。已建立的定量指标包括血管密度、血管直径指数、血管长度分数、血管分形维度、中心凹无血管区(FAZ)面积和中心凹无血管区周长,用于OCTA的客观评估(Wang等, 2021)。
Aastract
摘要
Optical coherence tomography angiography (OCTA) is a novel imaging modality that has been widely utilizedin ophthalmology and neuroscience studies to observe retinal vessels and microvascular systems. However,publicly available OCTA datasets remain scarce. In this paper, we introduce the largest and most comprehensiveOCTA dataset dubbed OCTA-500, which contains OCTA imaging under two fields of view (FOVs) from500 subjects. The dataset provides rich images and annotations including two modalities (OCT/OCTAvolumes), six types of projections, four types of text labels (age/gender/eye/disease) and seventypesofsegmentationlabels(large vessel/capillary/artery/vein/2D FAZ/3D FAZ/retinal layers). Then, we propose amulti-object segmentation task called CAVF, which integrates capillary segmentation, artery segmentation,vein segmentation, and FAZ segmentation under a unified framework. In addition, we optimize the 3D-to-2Dimage projection network (IPN) to IPN-V2 to serve as one of the segmentation baselines. Experimental resultsdemonstrate that IPN-V2 achieves an about 10% mIoU improvement over IPN on CAVF task. Finally, wefurther study the impact of several dataset characteristics: the training set size, the model input (OCT/OCTA,3D volume/2D projection), the baseline networks, and the diseases.
光学相干断层扫描血管造影(OCTA)是一种新型成像技术,已广泛应用于眼科和神经科学研究中,用于观察视网膜血管和微血管系统。然而,公开可用的OCTA数据集仍然稀缺。本文介绍了迄今为止最大且最全面的OCTA数据集,称为OCTA-500,该数据集包含来自500名受试者的两种视野(FOVs)下的OCTA成像。数据集提供了丰富的图像和注释,包括两种模态(OCT/OCTA体积)、六种投影类型、四种文本标签(年龄/性别/眼别/疾病)以及七种分割标签(大血管/毛细血管/动脉/静脉/2D FAZ/3D FAZ/视网膜层)。然后,我们提出了一个称为CAVF的多目标分割任务,将毛细血管分割、动脉分割、静脉分割和FAZ分割集成在一个统一框架下。此外,我们将3D到2D图像投影网络(IPN)优化为IPN-V2,作为分割基线之一。实验结果表明,IPN-V2在CAVF任务上比IPN的mIoU提高了约10%。最后,我们进一步研究了数据集特征的几个影响因素,包括训练集规模、模型输入(OCT/OCTA、3D体积/2D投影)、基线网络和疾病。
Conclusion
结论
We have introduced the new OCTA-500 dataset, which containsOCTA imaging from 500 subjects and provides a rich set of imagesand annotations. Based on the provided segmentation annotations, wehave proposed a new CAVF segmentation task that integrates arterysegmentation, vein segmentation, capillary segmentation, and FAZ segmentation under a unified framework. Focusing on the proposed CAVFtask, we optimized the 3D-to-2D network IPN to IPN-V2 to serve asone of the baselines. We have explored the effect of several datasetcharacteristics on the CAVF task: the training set size, the model input,the baselines, and the diseases.The experiments show that data are a driving factor for the segmentation performance in the proposed task. Our dataset has been ata reasonable level in terms of data scale. The proposed IPN-V2 hasimproved the quality and speed of segmentation by a large margincompared with IPN, and achieved competitive results. We also showthat the disease diversity of OCTA-500 increases the challenge of thesegmentation task. The considered deep learning methods have not yetbeen saturated in this task. Future improvement will come from bettermethods and increased data.OCTA-500 allows us to implement a variety of segmentation tasks,which will provide a systematic quantitative framework for retinalimage analysis. We also discussed its potential applications in otherOCTA studies. Hence, we expect that it will stimulate research toward the quantification, analysis and application of OCT/OCTA images.Our future plans are to continue collecting images, annotating groundtruths, and optimizing methods for more OCTA studies.
我们介绍了新的 OCTA-500 数据集,该数据集包含来自 500 名受试者的 OCTA 成像,并提供了丰富的图像和标注集。基于所提供的分割标注,我们提出了一个新的 CAVF 分割任务,将动脉分割、静脉分割、毛细血管分割和 FAZ 分割集成在一个统一框架下。围绕所提出的 CAVF 任务,我们将 3D 到 2D 网络 IPN 优化为 IPN-V2 作为基线之一。我们还研究了数据集特征(如训练集规模、模型输入、基线和疾病)对 CAVF 任务的影响。
实验结果表明,数据是该分割任务性能的关键因素。我们的数据集在数据规模方面已达到了合理水平。相比 IPN,提出的 IPN-V2 显著提高了分割的质量和速度,且取得了具有竞争力的结果。同时,我们也展示了 OCTA-500 的疾病多样性增加了分割任务的挑战性。目前所用的深度学习方法在该任务中尚未达到饱和。未来的改进将来自更好的方法和更多的数据。
OCTA-500 使我们能够实现多种分割任务,从而为视网膜图像分析提供系统的定量框架。我们还讨论了其在其他 OCTA 研究中的潜在应用。因此,我们期望该数据集将推动 OCT/OCTA 图像的量化、分析和应用研究。我们未来的计划是继续收集图像、标注真实数据,并优化方法以支持更多的 OCTA 研究。
Figure
图
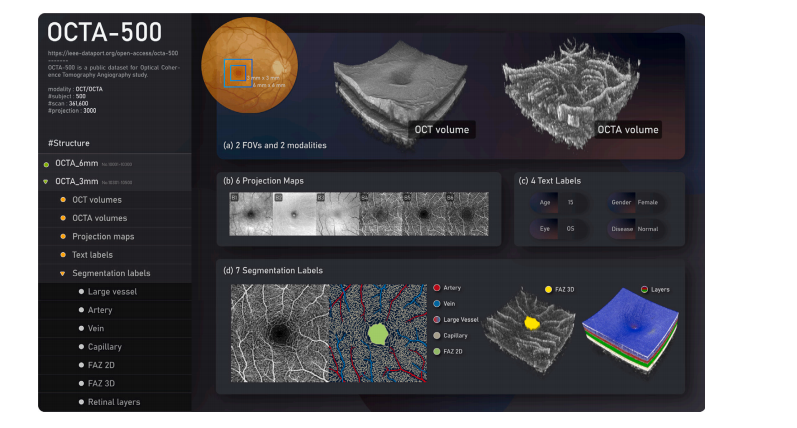
ig. 1. The structure and contents of the OCTA-500 dataset.
图 1. OCTA-500 数据集的结构和内容。
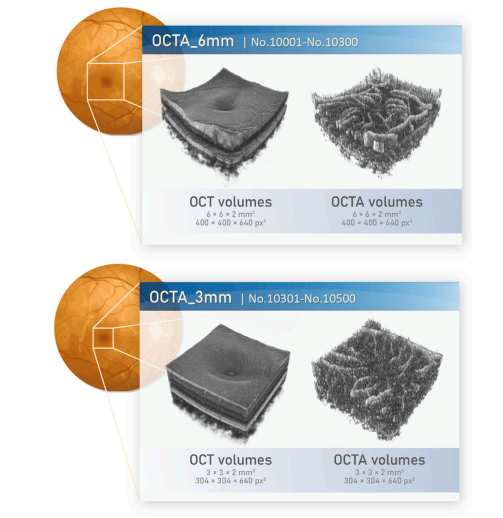
Fig. 2. 3D visualization of the OCT volumes and OCTA volumes in two fields of view.The order of size representation for the volumes is as follows: horizontal 𝑥 vertical 𝑥axial
图 2. 两种视野下 OCT 体积和 OCTA 体积的三维可视化。体积大小的表示顺序如下:水平 𝑥 垂直 𝑥 轴向。
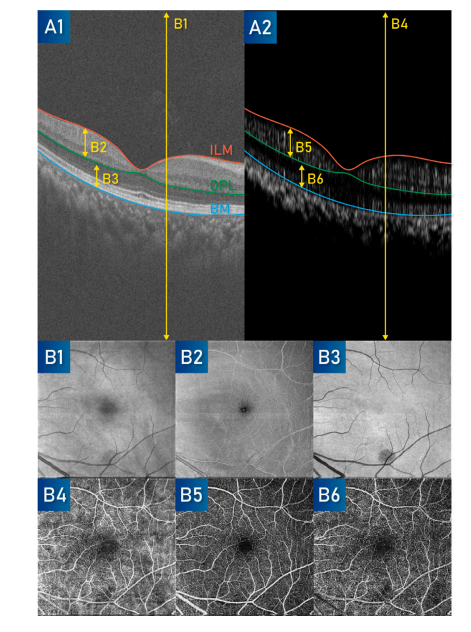
Fig. 3. Generation of the projection maps B1–B6. A1 and A2 show a B-scan ofOCT/OCTA and the layer segmentation used. The yellow arrows indicate the rangeof projection. B1–B4 use the average projection. B5-B6 use the maximum projection
图 3. 投影图 B1–B6 的生成过程。A1 和 A2 显示了 OCT/OCTA 的 B 扫描及所用的层分割。黄色箭头表示投影范围。B1–B4 使用平均投影,B5–B6 使用最大投影。
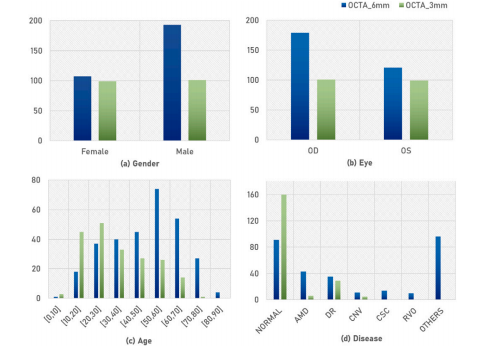
Fig. 4. Statistical histogram of text labels in OCTA-500: (a) gender, (b) eye, © age,(d) disease. ‘OD’ means right eye, ‘OS’ means left eye.
图 4. OCTA-500 数据集中文本标签的统计直方图:(a) 性别,(b) 眼别,© 年龄,(d) 疾病。‘OD’ 表示右眼,‘OS’ 表示左眼。
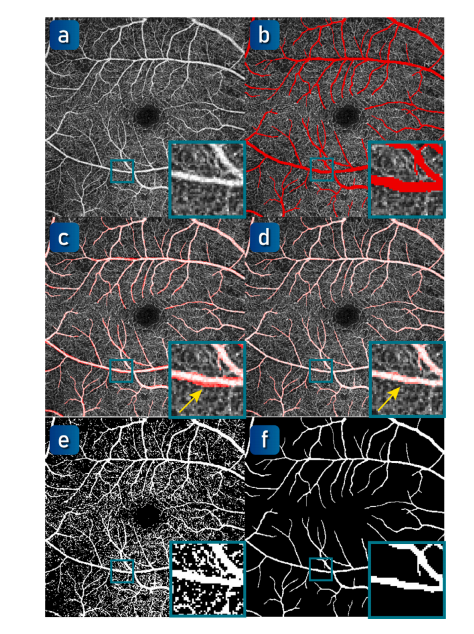
Fig. 5. Annotation of the large vessels in OCTA-500. (a) OCTA inner-retinal projection (B5). (b) Coarse-grained manual annotation. © Visualization of coarse-grainedannotation in ‘Screen’ mode. (d) Fine-grained manual corrections in ‘Screen’ mode. (e)Threshold result. (f) The final label of large vessels
图 5. OCTA-500 中大血管的标注。(a) OCTA 内视网膜投影 (B5)。(b) 粗粒度人工标注。© “屏幕”模式下的粗粒度标注可视化。(d) “屏幕”模式下的细粒度人工校正。(e) 阈值结果。(f) 大血管的最终标注。
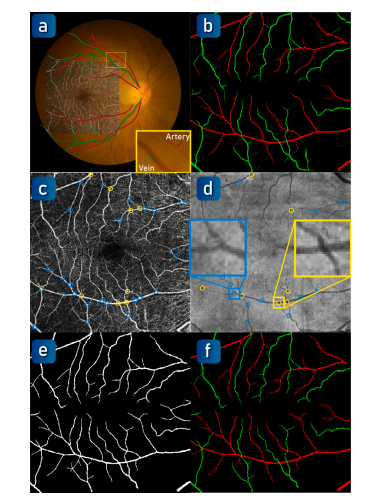
Fig. 6. Annotation of the arteries and veins in OCTA-500. (a) Color fundus image.(b) The mean results of models trained on an extra dataset. © OCTA inner-retinalprojection (B5). (d) OCT outer-retinal projection (B3). Blue circles represent branchpoints and yellow circles represent crossover points. (e) The large vessel label. (f) Thefinal artery-vein label. Red represents arteries. Green represents veins.
图 6. OCTA-500 中动脉和静脉的标注。(a) 彩色眼底图像。(b) 基于额外数据集训练的模型平均结果。© OCTA 内视网膜投影 (B5)。(d) OCT 外视网膜投影 (B3)。蓝色圆圈表示分叉点,黄色圆圈表示交叉点。(e) 大血管标注。(f) 最终的动脉-静脉标注。红色表示动脉,绿色表示静脉。
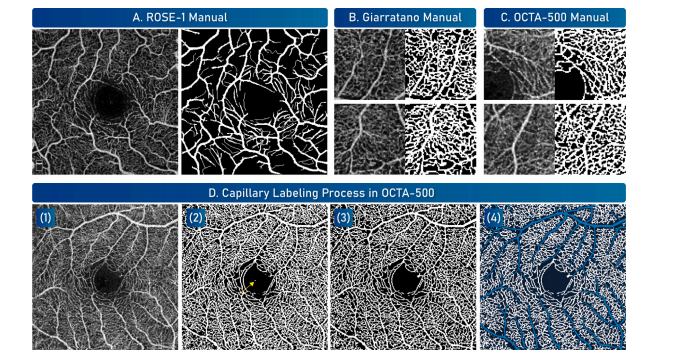
Fig. 7. Annotation of the capillary in OCTA-500. (A) An example of manual annotation from ROSE-1. (B) Examples of manual annotation in Giarratano. © Examples of manuallabeling of OCTA-500 slices. (D) Capillary labeling process in OCTA-500: (1) OCTA inner-retinal projection (B5). (2) The preliminary segmentation result using IMN. (3) The resultof topology optimization and denoising using LAL. (4) The final label. Blue represents the large vessels. White represents the capillaries.
图 7. OCTA-500 中毛细血管的标注。(A) ROSE-1 的人工标注示例。(B) Giarratano 数据集中人工标注的示例。© OCTA-500 切片的人工标注示例。(D) OCTA-500 中毛细血管的标注过程:(1) OCTA 内视网膜投影 (B5)。(2) 使用 IMN 进行的初步分割结果。(3) 使用 LAL 进行拓扑优化和去噪的结果。(4) 最终标注。蓝色表示大血管,白色表示毛细血管。
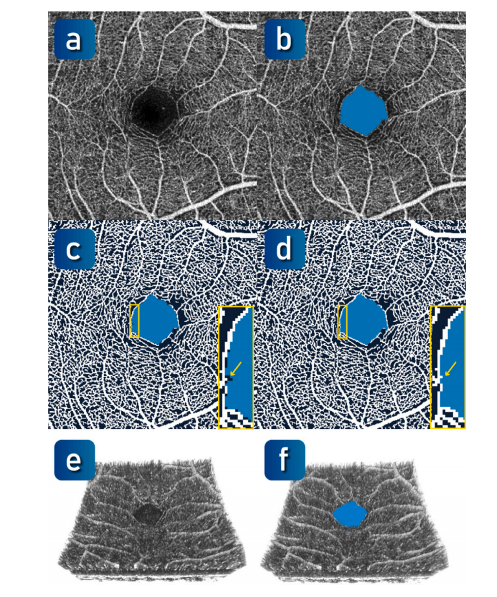
Fig. 8. Annotation of the FAZ in OCTA-500. (a) OCTA inner-retinal projection (B5).(b) Preliminary manual annotation of the FAZ. © Visualization of the FAZ label withthe capillary label. (d) The optimized FAZ label. (e) Visualization of the OCTA volume.(f) OCTA volume with 3D FAZ label.
图 8. OCTA-500 中 FAZ 的标注。(a) OCTA 内视网膜投影 (B5)。(b) FAZ 的初步人工标注。© 带有毛细血管标注的 FAZ 标签可视化。(d) 优化后的 FAZ 标签。(e) OCTA 体积可视化。(f) 带有 3D FAZ 标签的 OCTA 体积。
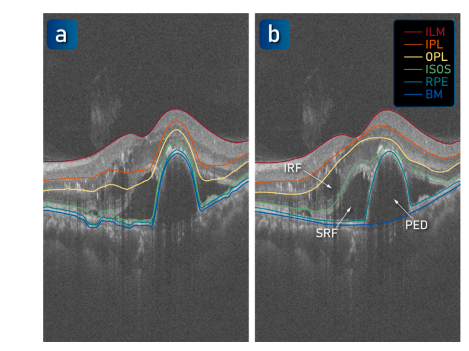
Fig. 9. Annotation of the retinal layers in OCTA-500. (a) An example of layersegmentation from an AMD patient using the Iowa software. (b) The layer labels aftermanual correction.
图 9. OCTA-500 中视网膜层的标注。(a) 使用 Iowa 软件对一名 AMD 患者进行层分割的示例。(b) 手动校正后的层标签。
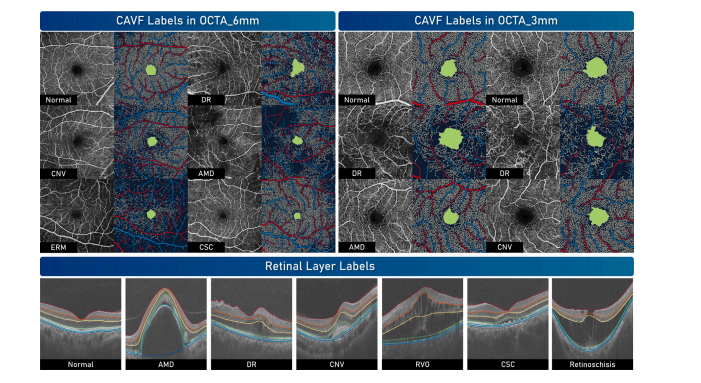
Fig. 10. Diversity of the segmentation labels in the OCTA-500 dataset
图 10. OCTA-500 数据集中分割标签的多样性。
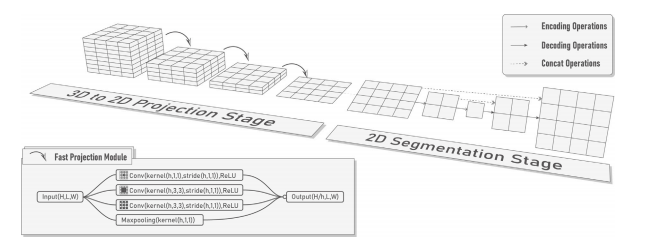
Fig. 11. Proposed IPN-V2 architecture for 3D-to-2D segmentation.
图 11. 用于 3D 到 2D 分割的 IPN-V2 架构。
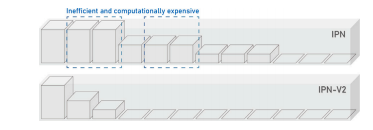
Fig. 12. Schematic representation of the computational space of IPN and IPN-V2.
图 12. IPN 和 IPN-V2 计算空间的示意图。
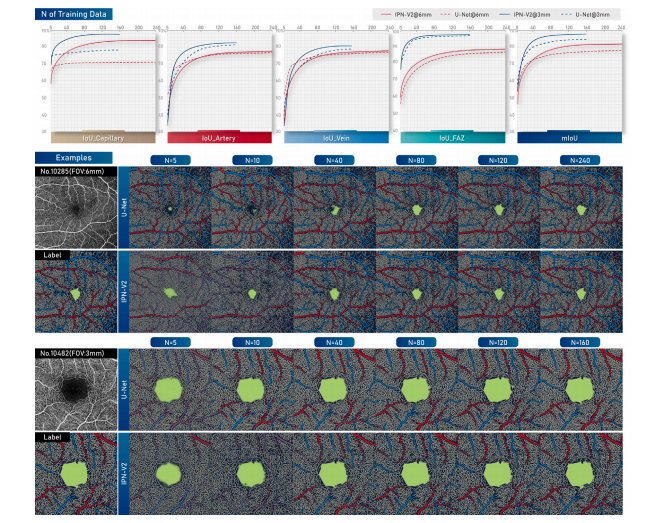
Fig. 13. Evaluations and examples in the experiment of varying training data size.
图 13. 不同训练数据规模实验中的评估结果和示例。
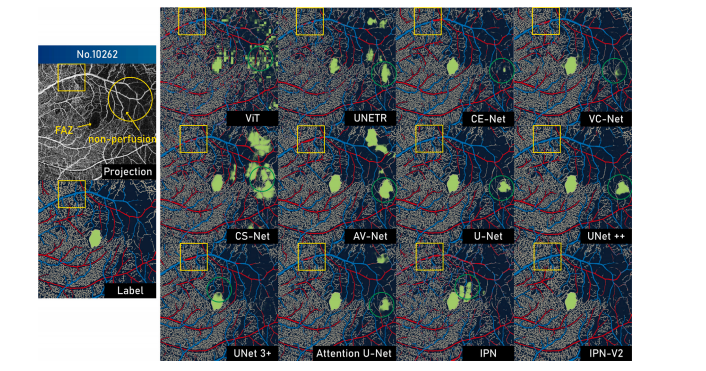
Fig. 14. An example of segmentation results using different baselines.
图 14. 使用不同基线的分割结果示例。
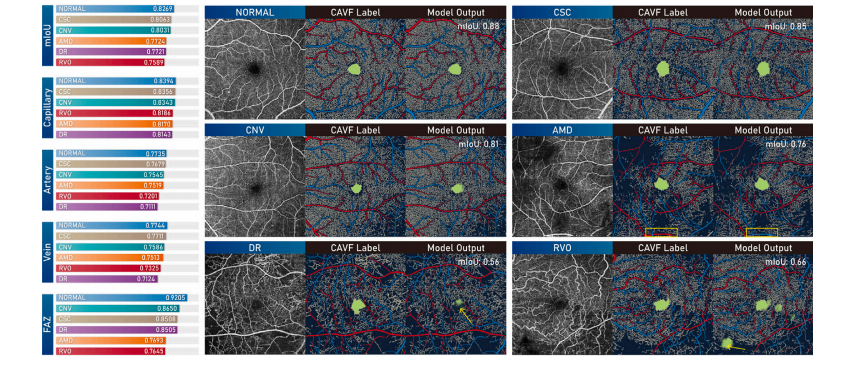
Fig. 15. Segmentation performance on different diseases using IPN-V2 on OCTA-6mm.
图 15. 在 OCTA-6mm 上使用 IPN-V2 对不同疾病的分割性能。
Table
表
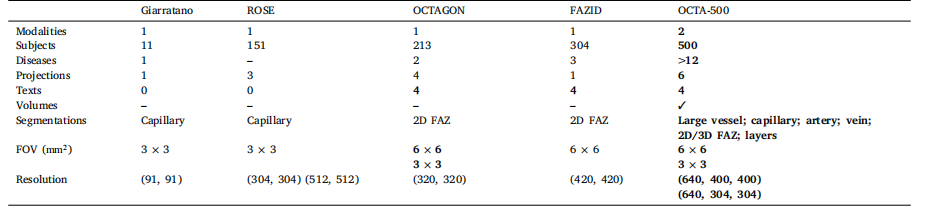
Table 1Summary of public OCTA datasets.
表 1公共 OCTA 数据集概览。
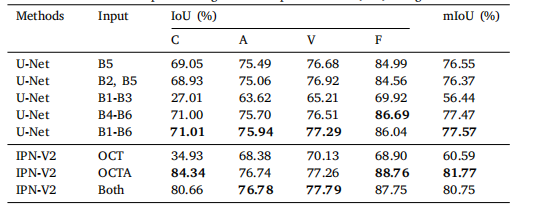
Table 2The effect of different inputs on segmentation performance (IoU) using OCTA-6mm.
表 2不同输入对 OCTA-6mm 分割性能 (IoU) 的影响。
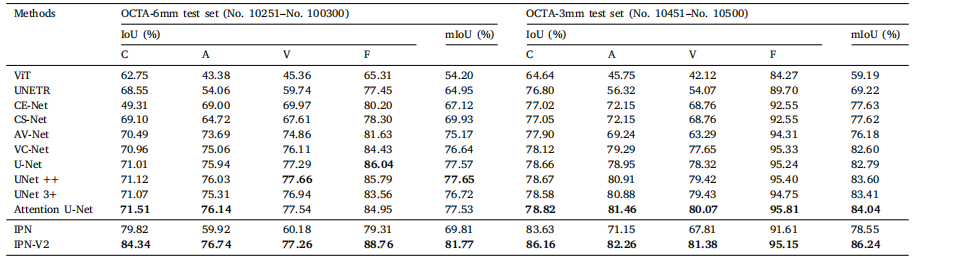
Table 3Results (IoU) of different baselines on OCTA-500.
表 3不同基线在 OCTA-500 上的结果 (IoU)。