Title
题目
Detection and subtyping of basal cell carcinoma in whole-slide histopathology using weakly-supervised learning
利用弱监督学习在全切片病理图像中检测和分型基底细胞癌
01
文献速递介绍
基底细胞癌 (BCC) 的发病率正在给病理诊断带来压力。BCC 的发病率在所有肿瘤中最高,占美国、澳大利亚和欧洲所有皮肤癌病例的 70%以上。此外,BCC 的全球发病率正在迅速上升,有报告显示,在过去三十年中,发病率几乎翻了一倍(De Vries 等, 2005; Leiter 等, 2017; Lomas 等, 2012; Apalla 等, 2017)。
病理学家经常需要评估组织切片中是否存在 BCC 并提供其形态亚型信息。尽管目前没有正式的 BCC 分级系统,通常会根据特定的形态亚型将 BCC 区分为低风险和高风险(Kim 等, 2019)。虽然诊断 BCC 对于病理学家来说并不算困难,但需要评估的切片数量过多,尤其是在许多国家病理学家短缺的情况下,这种工作量可能是巨大的。
为了在未来使 BCC 诊断变得更加可控,人工智能 (AI) 算法在减少皮肤病理学家所需的时间和精力方面可以发挥重要作用。以往的研究中已使用深度学习,尤其是深度卷积神经网络 (CNN),用于 BCC 的检测。这些方法通常使用经验丰富的病理学家标注的像素级注释的小区域(Arevalo 等, 2015; Jiang 等, 2020)。然而,这种方法可能非常耗时且在 BCC 亚型分型上存在问题,因为高风险生长模式的精确标注更具挑战性。另一种替代方法是多实例学习 (MIL),该方法在处理大量皮肤活检并训练精确的 BCC 检测模型上效果显著,且不需要注释区域(Campanella 等, 2019)。但该方法假设良性切片中的所有区域均为良性,而标记为 BCC 的切片至少包含一个含有 BCC 的区域,这种假设会使网络局限于单个区域大小,从而导致数据利用效率较低。
聚类约束注意力多实例学习 (CLAM) 方法利用预训练的卷积神经网络将全切片图像编码为较小的特征集(每个区域一个特征向量)。然后,将聚合的特征向量通过一个注意力门、一个聚类头和一个分类头。此方法减少了网络视野的限制,且数据效率更高(Lu 等, 2021)。然而,该方法使用的是基于 ImageNet 预训练的固定编码器进行特征提取,可能会导致下游分类任务的特征次优,同时限制了输入图像的数据增强。
在本研究中,我们的目标是使用弱监督方法解决 BCC 及其亚型检测相关的挑战。我们的研究分为两个主要部分。第一部分聚焦于利用弱监督技术实现高准确度检测和分类结果的可行性,考虑到获取精确亚型信息和注释的实际影响和限制。
第二部分中,我们假设 Streaming CLAM 相较于 CLAM 能更有效地解决该问题。考虑 Streaming CLAM 模型的原因在于其能够利用 Pinckaers 等人(2019)提出的端到端 CNN 流式处理方法。该方法使得编码器可以学习 WSI 的任务特定特征表示(Pinckaers 等, 2022)并允许数据增强。我们假设这将带来更高的性能和更数据高效的弱监督学习方法,用于 BCC 的检测和分型。
1.1. 研究贡献
本研究在自动化 BCC 分类方面做出了几项重要贡献。首先,提出了一种基于弱监督学习的创新 BCC 检测和分型算法,结合流式和注意力机制,并与先进的基准进行对比。其次,本研究首次将该算法的分型性能与两位专家病理学家进行了对比,并在两个外部数据集上进行了进一步验证。第三,我们公开了最大规模的皮肤全切片图像数据集,涵盖含有和不含 BCC 的共计 5147 张图像,总计 666 GB。
Aastract
摘要
The frequency of basal cell carcinoma (BCC) cases is putting an increasing strain on dermatopathologists. BCCis the most common type of skin cancer, and its incidence is increasing rapidly worldwide. AI can play asignificant role in reducing the time and effort required for BCC diagnostics and thus improve the overallefficiency of the process. To train such an AI system in a fully-supervised fashion however, would require alarge amount of pixel-level annotation by already strained dermatopathologists. Therefore, in this study, ourprimary objective was to develop a weakly-supervised for the identification of basal cell carcinoma (BCC) andthe stratification of BCC into low-risk and high-risk categories within histopathology whole-slide images (WSI).We compared Clustering-constrained Attention Multiple instance learning (CLAM) with StreamingCLAM andhypothesized that the latter would be the superior approach. A total of 5147 images were used to train andvalidate the models, which were subsequently tested on an internal set of 949 images and an external setof 183 images. The labels for training were automatically extracted from free-text pathology reports usinga rule-based approach. All data has been made available through the COBRA dataset. The results showedthat both the CLAM and StreamingCLAM models achieved high performance for the detection of BCC, withan area under the ROC curve (AUC) of 0.994 and 0.997, respectively, on the internal test set and 0.983and 0.993 on the external dataset. Furthermore, the models performed well on risk stratification, with AUCvalues of 0.912 and 0.931, respectively, on the internal set, and 0.851 and 0.883 on the external set. In everysingle metric the StreamingCLAM model outperformed the CLAM model or is on par. The performance ofboth models was comparable to that of two pathologists who scored 240 BCC positive slides. Additionally, inthe public test set, StreamingCLAM demonstrated a comparable AUC of 0.958, markedly superior to CLAM’s0.803. This difference was statistically significant and emphasized the strength and better adaptability of theStreamingCLAM approach.
基底细胞癌 (BCC) 病例的频率正对皮肤病理学家造成日益增加的压力。BCC 是最常见的皮肤癌类型,且其发病率在全球范围内迅速上升。人工智能 (AI) 在减少 BCC 诊断所需的时间和精力方面可以发挥重要作用,从而提高整个过程的效率。然而,要以全监督的方式训练这样一个 AI 系统,则需要大量的像素级标注,这对已然负担过重的皮肤病理学家来说是极具挑战的。因此,本研究的主要目标是开发一种弱监督方法,以便在病理全切片图像 (WSI) 中识别基底细胞癌 (BCC) 并将其分为低风险和高风险类别。
我们比较了聚类约束的注意力多实例学习 (CLAM) 和 StreamingCLAM,假设后者是更优的方法。共有 5147 张图像用于模型的训练和验证,随后在内部测试集 (949 张图像) 和外部测试集 (183 张图像) 上进行了测试。训练标签通过基于规则的方法从病理报告的自由文本中自动提取。所有数据都通过 COBRA 数据集公开提供。结果显示,CLAM 和 StreamingCLAM 模型在检测 BCC 方面都表现出高性能,在内部测试集上分别达到了 0.994 和 0.997 的 ROC 曲线下面积 (AUC),在外部数据集上则分别达到了 0.983 和 0.993。此外,这些模型在风险分层方面的表现也很好,在内部数据集上的 AUC 值分别为 0.912 和 0.931,而在外部数据集上分别为 0.851 和 0.883。在每一个指标上,StreamingCLAM 模型均优于或与 CLAM 模型持平。两种模型的性能与两位对 240 张 BCC 阳性切片进行评分的病理学家相当。此外,在公开测试集上,StreamingCLAM 展现出 0.958 的 AUC,显著优于 CLAM 的 0.803。此差异在统计学上具有显著性,突显了 StreamingCLAM 方法的优势及其更强的适应性。
Method
方法
3.1. Tissue segmentation and packing
In the process of digitizing pathological biopsies, a significantamount of white space may be present due to the small size of thebiopsy resulting in cuts that take up little space on the glass slide. Toorganize the data more efficiently for training both weakly-supervisedtechniques, two pre-processing steps were implemented.The first step involved the detection of tissue within the biopsy samples. By identifying tissue regions, the white space can be eliminated orskipped. A fully-supervised, patch-based, DenseNet model was trainedon 50 annotated slides for this purpose (see Fig. 2A). To enhance theaccuracy of segmentation around edges, a higher sampling rate wasapplied to annotations located near the edges of tissue, as opposedto random sampling outside of tissue, which often results in emptypatches. Additionally, more sampling was done in areas containingartifacts such as scratches and stains outside of tissue regions.An additional second step was executed for StreamingCLAM tominimize the input image size in order to process the entire imagewith the network. To accomplish this, a packer algorithm was implemented to tightly pack sections of tissue. This was achieved by utilizingthe findContours function from the opencv-python (4.5) library todetect individual objects in the tissue segmentation mask, extracting thebounding boxes, and using the python library rectangle-packer (2.0.1)to efficiently fill the canvas and minimize white space (see Fig. 2B).
3.1. 组织分割和包装
在数字化病理活检的过程中,由于活检样本的尺寸较小,切片在玻片上占据的空间很少,因此常会出现大量的空白区域。为提高数据的训练效率,特别是在弱监督技术的应用中,我们实施了两步预处理过程。
第一步是检测活检样本中的组织区域。通过识别组织区域,可以消除或跳过空白区域。为此,训练了一个基于全监督的、以切片为单位的 DenseNet 模型,模型基于 50 张标注的切片进行训练(见图 2A)。为了提高边缘区域的分割准确性,在组织边缘附近应用了更高的采样率,而非在组织外的随机采样,这样可以避免空白区域。此外,还增加了在包含划痕和污渍等伪影的区域进行采样的频率,以提升模型对这些区域的识别能力。
第二步是为 StreamingCLAM 优化输入图像的尺寸,以便网络能够处理整个图像。为此,我们实施了一种打包算法,以紧凑地排列组织区域。具体方法是利用 opencv-python (4.5) 库中的 findContours 函数检测组织分割掩膜中的单个对象,提取边界框,并使用 python 库 rectangle-packer (2.0.1) 高效填充画布,以最大限度地减少空白区域(见图 2B)。
Results
结果
4.1. Model performance
We evaluated the performance of the two models designed for eachdistinct task. The first task is to differentiate between non-BCC andBCC cases, while the second task involved assessing the risk of BCCin low-risk and high-risk cases.For the first task, on the internal test set, the StreamingCLAMmodel yielded a mean AUC of 0.997 (95% CI 0.995–0.999), marginallyoutperforming the CLAM model which had an AUC of 0.994 (95%CI 0.990–0.997). Although this performance gap is minute, spanningonly thousandths, DeLong’s test confirmed the difference to be statistically significant (Z = −2.2555, 𝑝-value = 0.0241, 95% CI: −0.0070 to−0.0004). On the external test set, while the StreamingCLAM model’smean AUC of 0.993 (95% CI 0.986–1.000) showed a slightly largergap over the CLAM model’s mean AUC of 0.983 (95% CI 0.969–0.998)compared to the internal set, this difference did not reach statisticalsignificance (Z = −1.5534, 𝑝-value = 0.1203, 95% CI: −0.0220 to0.0025).
我们评估了两个模型在各自不同任务中的性能。第一个任务是区分非基底细胞癌 (non-BCC) 和基底细胞癌 (BCC) 病例,第二个任务是评估 BCC 的低风险和高风险。
对于第一个任务,在内部测试集中,StreamingCLAM 模型的平均 AUC 为 0.997(95% 置信区间 0.995–0.999),略微优于 CLAM 模型,其 AUC 为 0.994(95% 置信区间 0.990–0.997)。尽管这一性能差异仅为千分位,但 DeLong 检验确认了该差异在统计学上显著(Z = -2.2555,𝑝 值 = 0.0241,95% 置信区间:-0.0070 至 -0.0004)。在外部测试集中,StreamingCLAM 模型的平均 AUC 为 0.993(95% 置信区间 0.986–1.000),相较于 CLAM 模型的平均 AUC 0.983(95% 置信区间 0.969–0.998),差距稍大于内部测试集,但该差异未达到统计显著性(Z = -1.5534,𝑝 值 = 0.1203,95% 置信区间:-0.0220 至 0.0025)。
Figure
图
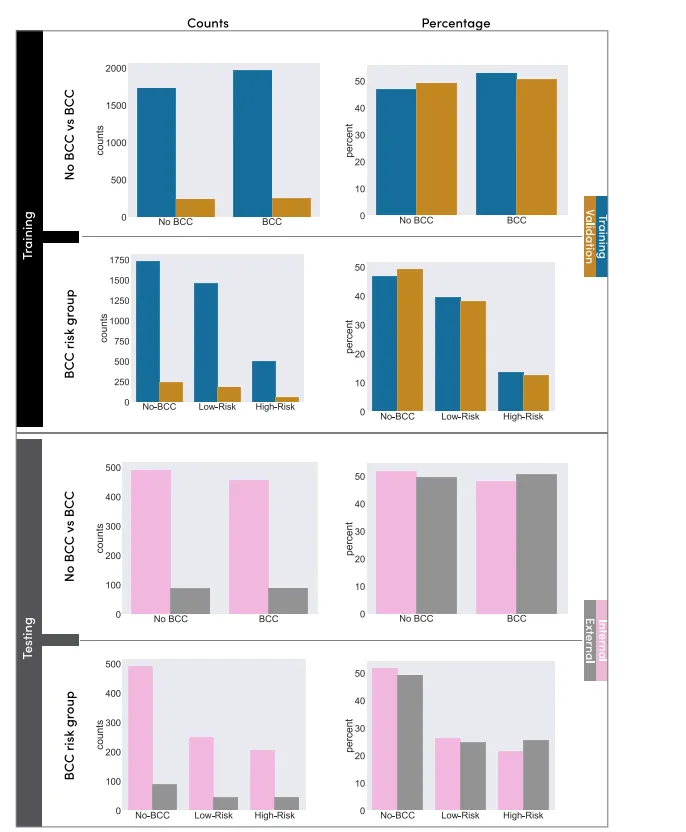
Fig. 1. Overview of the data and label distribution of the training and testing sets. The training set is divided into a training subset and a validation subset, while the testing setis divided into an internal and an external test set. The first column shows the absolute number of cases for each set, and the second column presents the ratio.
1. 训练集和测试集的数据和标签分布概览。训练集分为训练子集和验证子集,而测试集分为内部测试集和外部测试集。第一列显示每个数据集的绝对病例数,第二列显示相应的比例。
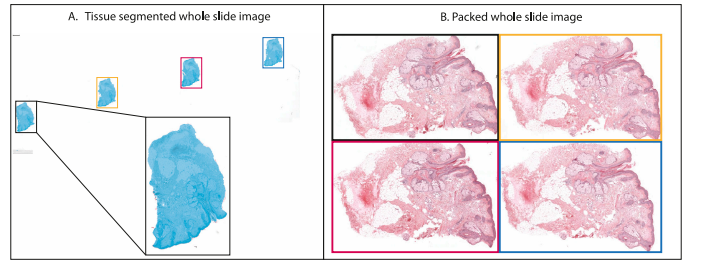
Fig. 2. Example of tissue segmentation and image packing. The left figure shows the output of the tissue segmentation model as a light-blue overlay on top of the originalwhole-slide image. The right figure shows the individual tissue pieces packed such as to minimize the white space between them.
图 2. 组织分割和图像打包示例。左图展示了组织分割模型的输出结果,作为浅蓝色叠加层覆盖在原始全切片图像上。右图展示了紧密排列的各个组织块,以最大程度减少它们之间的空白区域。
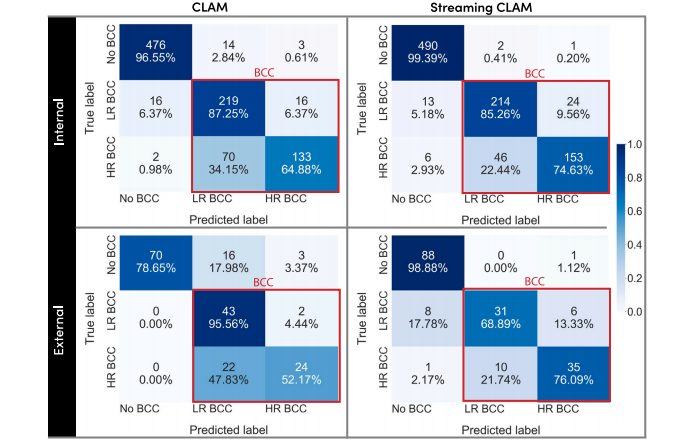
Fig. 3. Confusion matrices for both CLAM (left) and StreamingCLAM (right). The confusion matrices for both the internal and external test set are shown. The red box groups are true positive BCC.
图 3. CLAM(左)和 StreamingCLAM(右)的混淆矩阵。展示了内部和外部测试集的混淆矩阵。红色框内的部分表示基底细胞癌(BCC)的真正例数。
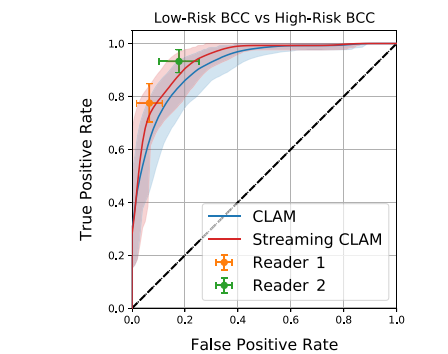
Fig. 4. Bootstrapped ROC Analysis for Discriminating Between Low-Risk and High-RiskBCC. The ROC curve displays the performance of two models in comparison to twopathologists in differentiating between low-risk and high-risk BCC. The curves of themodels are plotted with 95% CI and compared to the performance of the pathologists,also shown with 95% CI
4. 用于区分低风险和高风险基底细胞癌 (BCC) 的自助法 ROC 分析。ROC 曲线展示了两个模型在区分低风险和高风险 BCC 时的性能,并与两位病理学家的表现进行比较。模型的曲线绘制了 95% 置信区间,与病理学家的表现(同样带有 95% 置信区间)进行了对比。
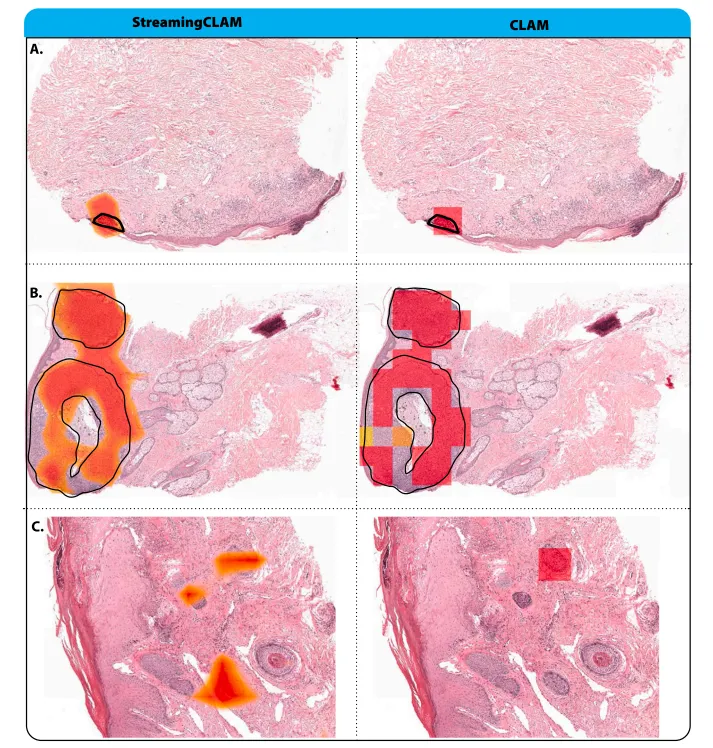
Fig. 5. Attention maps for both StreamingCLAM and CLAM models. (A) Both models show high attention values in a confined region corresponding to the tumor area (indicatedby the black line). Inflammated areas and crusts, often present near tumor sites, receive low attention values. (B) A larger tumor region (highlighted by the black line) whereboth models exhibit high attention values. Adnex structures and color artifacts are disregarded by the models, as evidenced by their low attention values. © Illustration of afalse positive: both models concentrate on hair follicles. It is probable that the models identified these slides as positive due to the resemblance of these hair follicles to basal cellcarcinoma (BCC) features.
图 5. StreamingCLAM 和 CLAM 模型的注意力图。(A) 两个模型在与肿瘤区域(黑线标示)相对应的限定区域内显示出较高的注意力值。炎症区域和痂皮,通常位于肿瘤部位附近,表现出低注意力值。(B) 一个更大的肿瘤区域(黑线标示),两个模型均在此区域显示出高注意力值。附属结构和颜色伪影被模型忽略,表现为低注意力值。© 错误阳性示例:两个模型都集中在毛囊上。模型可能将这些切片识别为阳性,因为这些毛囊的特征与基底细胞癌 (BCC) 的特征相似。
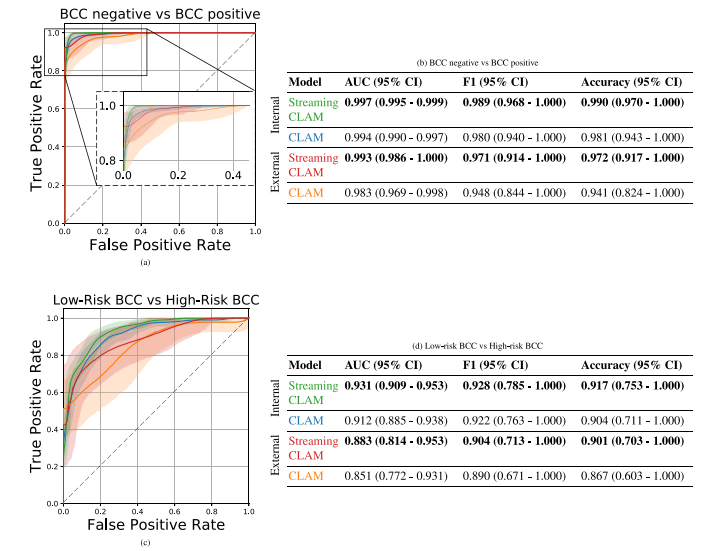
Fig. 6. Figure a. and Table b. show the ROC curves and metrics for discriminating between non-BCC and BCC lesions, while Figure c. and Table d. show the ROC curves andmetrics for stratifying BCC risk into low-risk and high-risk categories. The ROC curves are generated using bootstrapped samples, with the shaded areas representing 95% confidenceintervals. The figures display the ROC curves of two models (CLAM and StreamingCLAM) evaluated on an internal and external dataset, represented by the corresponding colorsin the tables. The tables show the mean AUC, mean F1, and mean accuracy for both tasks with 95% CI.
图 6. 图 a 和表 b 显示了用于区分非 BCC 和 BCC 病灶的 ROC 曲线和指标,而图 c 和表 d 显示了用于将 BCC 风险分为低风险和高风险类别的 ROC 曲线和指标。ROC 曲线基于自助法样本生成,阴影区域表示 95% 置信区间。图中展示了在内部和外部数据集上评估的两个模型(CLAM 和 StreamingCLAM)的 ROC 曲线,不同颜色对应于表中的数据集。表格显示了两个任务的平均 AUC、平均 F1 和平均准确率,以及 95% 置信区间。
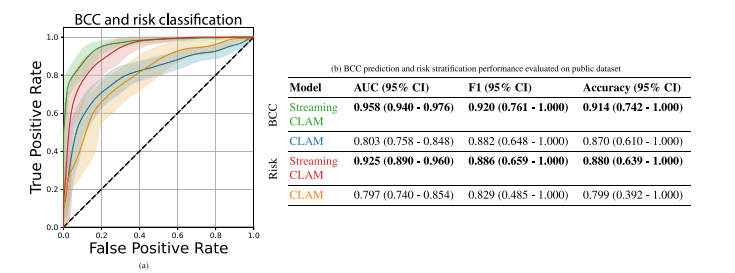
Fig. 7. All evaluations performed on a public dataset. Figure a. and Table b. display the ROC curves and performance metrics for two tasks: (1) discriminating between non-BCCand BCC lesions and (2) stratifying BCC risk into low-risk and high-risk categories. Both the Streaming CLAM and CLAM models’ results are included for each task. The ROCcurves, generated using bootstrapped samples, are shown with shaded areas indicating the 95% confidence intervals. Different colors in the figures correspond to the two models’results, which are elaborated in the tables. Table b. provides the mean AUC, mean F1, and mean accuracy for both detection and risk classification tasks with their 95% CI.
图 7. 在公共数据集上进行的所有评估。图 a 和表 b 显示了两个任务的 ROC 曲线和性能指标:(1)区分非 BCC 和 BCC 病灶,(2)将 BCC 风险分为低风险和高风险类别。每个任务均包含 Streaming CLAM 和 CLAM 模型的结果。ROC 曲线基于自助法样本生成,阴影区域表示 95% 置信区间。图中不同颜色表示两个模型的结果,具体数据在表中详细列出。表 b 提供了检测和风险分类任务的平均 AUC、平均 F1 和平均准确率及其 95% 置信区间。
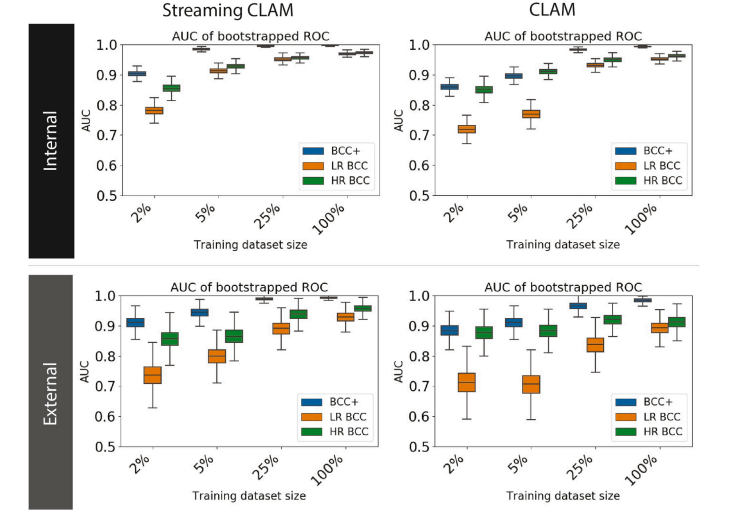
Fig. 8. Each boxplot displays the distribution of AUC values from bootstrapped samples of two models (StreamingCLAM and CLAM) on two datasets (Internal and External). Thefour groups in each boxplot correspond to the amount of data used to train the models (2%, 5%, 25%, and 100%). The discrimination tasks shown are non-BCC vs BCC (BCC+),and the stratification of BCC risk into low-risk (LR BCC) and high-risk (HR BCC) categories. The AUC values are shown for each task and dataset combination. The horizontalline within each box represents the median, the box represents the interquartile range (IQR), and the whiskers extend to the most extreme data points within 1.5 times the IQR.Outliers are not shown.
图 8. 每个箱线图展示了两个模型(StreamingCLAM 和 CLAM)在两个数据集(内部和外部)上的 AUC 值分布,这些值来自自助法样本。每个箱线图中的四组数据分别对应用于训练模型的数据量(2%、5%、25% 和 100%)。显示的区分任务包括非 BCC 与 BCC(BCC+)的区分,以及将 BCC 风险分为低风险(LR BCC)和高风险(HR BCC)类别的分层。AUC 值展示了每个任务和数据集组合的结果。箱线图中的水平线表示中位数,箱体表示四分位距 (IQR),须线延伸到 1.5 倍 IQR 范围内的最极端数据点。离群值未显示。
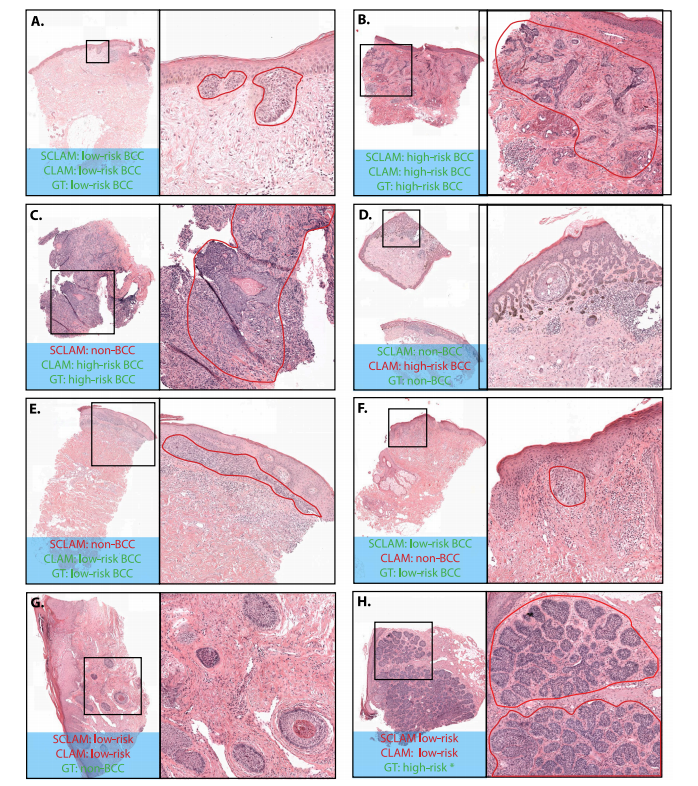
Fig. 9. Whole Slide Images of BCC lesions with close-up Regions of Interest (ROIs) and model predictions. In the ROIs, the tumor area is outlined in red. The top row (A–B)shows cases where StreamingCLAM (SCLAM) and CLAM agree with the ground truth (GT). The second (C–D) and third (E–F) rows show cases where either StreamingCLAM orCLAM makes an incorrect prediction in either BCC detection or risk stratification. The last row (G–H) shows a false positive case where both models predict low-risk BCC insteadof non-BCC, and another case where both models predict low-risk BCC while the ground truth is high-risk BCC. After inspection by pathologists LH and AA, it was determinedthat this case had been mislabeled as high-risk BCC and should have been labeled as low-risk BCC.
图 9. 基底细胞癌 (BCC) 病灶的全切片图像及感兴趣区域 (ROIs) 的特写和模型预测。在 ROIs 中,肿瘤区域用红色勾勒。第一行 (A–B) 显示了 StreamingCLAM (SCLAM) 和 CLAM 与真实值 (GT) 一致的案例。第二行 (C–D) 和第三行 (E–F) 显示了 StreamingCLAM 或 CLAM 在 BCC 检测或风险分层中出现错误预测的案例。最后一行 (G–H) 显示了一个假阳性案例,其中两个模型都预测为低风险 BCC 而非非 BCC,以及另一个两个模型都预测为低风险 BCC 而真实值为高风险 BCC 的案例。* 在病理学家 LH 和 AA 的检查下,确认该案例被错误标记为高风险 BCC,实际应标记为低风险 BCC。