Title
题目
GANDALF: Graph-based transformer and Data Augmentation Active Learning Framework with interpretable features for multi-label chest Xrayclassification
GANDALF: 基于图的Transformer与数据增强主动学习框架,具有可解释特征的多标签胸部X光分类
01
文献速递介绍
虽然标注的医学影像数据集对于深度学习(DL)方法取得最先进(SOTA)的性能至关重要,但由于标注医学数据需要高水平的专业知识和较高的成本,这一过程非常具有挑战性。主动学习(AL)方法使得专家可以选择信息性样本,从而允许模型在仅需少量标注样本的情况下获得高性能(即具有较高的学习率)。这对于医学图像分析任务尤为适用,因为AL方法需要适应不同的条件,如设备供应商、成像协议、机器学习模型等。
数据增强同样是一种有效的方法,它通过从训练数据集中生成合成样本为模型提供更多的训练数据(Perez 和 Wang, 2017)。尽管传统的数据增强方法(如翻转、旋转等)可以增加数据集的规模,但它并不能确保向训练集中添加了有信息的样本。近期的研究使用了神经网络方法进行数据增强,例如生成对抗网络(GAN)(Goodfellow等, 2014)、空间变换网络(STN)(Jaderberg等, 2015)和变分自编码器(VAE)(Kingma 和 Welling, 2013)。为更好地探索特征空间,Mixup(Zhang等, 2017)及其变体通过特征插值并使用对应的标签来增强数据集。
结合数据增强与主动学习可以发挥这两种方法的优势。Tran等(2019)提出了一种流水线式的方法,通过获取函数选择信息性样本,并从中生成增强样本。然而,这种顺序方法没有利用两步之间的相互作用,即获取函数并不评估增强样本可能带来的信息增益。因此,生成的数据不一定是信息性样本。Kim等(2021)提出了一种综合方法,称为前瞻性数据增强(LADA),它评估潜在增强样本的有用性并生成信息性样本。因此,增强样本与基础样本提供了质量上不同的信息。然而,正如我们实验所示,LADA方法在多标签环境中效果并不如单标签环境那样理想。
目前的AL和数据增强方法主要是为单标签设置开发的。相比之下,在某些情况下,如胸部X光影像,一张图像可能包含多个疾病标签。在多标签环境中选择信息性样本更具挑战性,因为需要考虑所有潜在类别标签的相互影响和相似性,以及不同类别的复杂程度(即某些疾病比其他疾病更容易检测)。此外,增强这些信息性样本时,也需要确保新样本的适当信息性。本文提出了一种新颖的多标签主动学习方法,并结合了一种针对信息性样本的数据增强的新方法。
Aastract
摘要
Informative sample selection in an active learning (AL) setting helps a machine learning system attain optimum performance with minimum labeled samples, thus reducing annotation costs and boosting performance of computer-aided diagnosis systems in the presence of limited labeled data. Another effective technique toenlarge datasets in a small labeled data regime is data augmentation. An intuitive active learning approachthus consists of combining informative sample selection and data augmentation to leverage their respectiveadvantages and improve the performance of AL systems. In this paper, we propose a novel approach calledGANDALF (Graph-based TrANsformer and Data Augmentation Active Learning Framework) to combine sampleselection and data augmentation in a multi-label setting. Conventional sample selection approaches in AL havemostly focused on the single-label setting where a sample has only one disease label. These approaches donot perform optimally when a sample can have multiple disease labels (e.g., in chest X-ray images). Weimprove upon state-of-the-art multi-label active learning techniques by representing disease labels as graphnodes and use graph attention transformers (GAT) to learn more effective inter-label relationships. We identifythe most informative samples by aggregating GAT representations. Subsequently, we generate transformationsof these informative samples by sampling from a learned latent space. From these generated samples, weidentify informative samples via a novel multi-label informativeness score, which beyond the state of theart, ensures that (i) generated samples are not redundant with respect to the training data and (ii) makeimportant contributions to the training stage. We apply our method to two public chest X-ray datasets, as wellas breast, dermatology, retina and kidney tissue microscopy MedMNIST datasets, and report improved resultsover state-of-the-art multi-label AL techniques in terms of model performance, learning rates, and robustness.
在主动学习(Active Learning, AL)设置中,信息性样本的选择可以帮助机器学习系统以最少的标注样本实现最佳性能,从而降低标注成本,并在有限标注数据的情况下提高计算机辅助诊断系统的性能。另一种有效的技术是在小规模标注数据的情况下扩大数据集,即数据增强。因此,一种直观的主动学习方法是结合信息性样本选择和数据增强,利用它们各自的优势来提高AL系统的性能。
在本文中,我们提出了一种新颖的方法,称为GANDALF(Graph-based TrANsformer and Data Augmentation Active Learning Framework),即基于图的Transformer与数据增强主动学习框架,用于在多标签环境下结合样本选择和数据增强。传统的样本选择方法大多集中于单标签环境,在这种环境下,每个样本只有一个疾病标签。然而,当一个样本可能有多个疾病标签时(例如在胸部X光影像中),这些方法无法获得最佳表现。
我们改进了现有的多标签主动学习技术,通过将疾病标签表示为图节点,并使用图注意力Transformer(Graph Attention Transformers, GAT)学习更有效的标签间关系。通过聚合GAT表示,我们识别出最具信息性的样本。随后,我们通过从学习的潜在空间中进行采样,生成这些信息性样本的转换版本。接着,我们使用一种新的多标签信息性评分来识别生成样本中的信息性样本,这超越了现有技术,确保了(i)生成的样本不会与训练数据冗余,且(ii)在训练阶段做出了重要贡献。
我们将该方法应用于两个公共胸部X光数据集,以及乳腺、皮肤病、视网膜和肾组织显微镜MedMNIST数据集,并在模型性能、学习速率和鲁棒性方面报告了相较于当前最先进的多标签主动学习技术的改进结果。
Method
方法
In our current work, we propose a model to identify multi-labelinformative samples by jointly considering the mutual influence of allpotential class labels. We also synthesize new samples from identifiedinformative real ones such that newly synthesized samples are warranted to be informative and provide new information to the trainingset. Fig. 1 depicts the different stages of our proposed workflow.We divide the description of the proposed active learning approachinto its two main components: (1) Sample selection based on multilabel informativeness scoring, and (2) Synthetic generation of informative and non-redundant samples. The general strategy is as follows:For the synthetic sample generation step, we take the initial batch oftraining images and train a variational autoencoder (VAE) to generateimages. In parallel, an initial classification model is trained with abaseline number of randomly chosen training samples (which can bethe same data used to train the VAE model, or a different one) to actas a baseline model for the active learning cycles. During test time,informative samples are identified by first deriving interpretabilitysaliency maps for each unlabeled sample (i.e, pool sample). Following Mahapatra et al. (2022a,b), we calculate saliency maps for allpotential class labels (intra-sample saliency maps), from which a graphis constructed with nodes corresponding to the latent representation ofeach class-specific saliency map, and edge weights characterizing thesimilarity between nodes. Different from Mahapatra et al. (2022a,b),in Section
在我们当前的工作中,我们提出了一种模型,旨在通过联合考虑所有潜在类别标签的相互影响来识别多标签信息性样本。我们还从已识别的信息性真实样本中合成新样本,确保新合成的样本具有信息性,并能为训练集提供新的信息。图1展示了我们提出的工作流程的各个阶段。
我们将所提出的主动学习方法分为两个主要部分来描述:(1) 基于多标签信息性评分的样本选择,(2) 合成生成信息性且无冗余的样本。其一般策略如下:
在合成样本生成步骤中,我们先选取初始的训练图像批次,并训练一个变分自编码器(VAE)来生成图像。同时,用随机选择的基准数量的训练样本(可以是用于训练VAE模型的数据,也可以是其他数据)训练一个初始分类模型,以作为主动学习循环的基线模型。在测试时,通过首先为每个未标记样本(即样本池)生成解释性显著图来识别信息性样本。
根据Mahapatra等人(2022a, b)的方法,我们为所有潜在类别标签计算显著图(即样本内部显著图),并基于此构建一个图结构,其中的节点对应于每个类别特定显著图的潜在表示,边的权重则表征节点之间的相似性。与Mahapatra等人的方法不同之处将在后续章节中详细讨论。
Conclusion
结论
In this paper, we present a novel approach for multi-label activelearning that combines active learning with data augmentation. We refer to the proposed method as ‘‘GANDALF’’ (Graph-based TrANsformerand Data Augmentation Active Learning Framework). The key motivation in combining active learning with data augmentation is toleverage their mutually complementary strengths and ensure that thedata augmentation step also generates informative samples from aninformative base sample.Unlike most current works that deal with multi-class AL, we focuson multi-label AL, where a given sample can have more than onedisease label. To learn the interaction between different disease labels,we use graphs to quantify the informativeness of each sample. Goingbeyond (Mahapatra et al., 2022a), which proposes simple aggregation strategies such as mean and the sum of node weights, we usegraph attention transformers with graph neural networks to learn morediscriminative graph aggregations.Complementing the improved graph aggregation strategy is theinformative augmentation step that takes a base informative image,generates augmented versions, and calculates a score based on labelpreservation and informativeness of the augmented images. The overallinformativeness of the augmented samples is the sum of the two scores,and the most informative samples are added to the training set forfurther training.Our proposed GANDALF method yields better results than our previous method, GESTALT (Mahapatra et al., 2022a), and other competingmethods. Subsequent ablation studies also highlight the importanceof the graph attention transformers and the informative augmentationstep in the overall performance of GANDALF.In future work, we aim to test our model on other multi-labelmedical image datasets. We also aim to test its robustness and generalizability to different classification architectures and segmentationmethods. We also anticipate that transformers will play a greaterrole in graph-based interpretability and active learning tasks. Hence,our future focus will be on exploiting the properties of graph attention transformers to learn more powerful graph representations onmulti-omics dataset combining imaging and non-imaging information.Current active learning methods are typically tested with initial datasetsizes in the order of 10% of the total available data, as also performedin this study. An interesting avenue of further research includes thecombination of new approaches proposed to work under more extremelow-label regimes, such as Taher et al. (2023) and Chen et al. (2023a,b)for active learning scenarios.
在本文中,我们提出了一种结合主动学习和数据增强的多标签主动学习新方法,称为“GANDALF” (Graph-based TrANsformer and Data Augmentation Active Learning Framework)。结合主动学习与数据增强的关键动机在于利用它们互补的优势,确保数据增强步骤从信息性基础样本中生成信息性样本。
与当前大多数处理多类主动学习(AL)的工作不同,我们专注于多标签主动学习,即一个样本可以有多个疾病标签。为了学习不同疾病标签之间的交互,我们使用图来量化每个样本的信息性。不同于(Mahapatra等, 2022a)中提出的简单聚合策略(如节点权重的均值和求和),我们使用图注意力Transformer结合图神经网络来学习更具区分性的图聚合方式。
在改进的图聚合策略基础上,信息性增强步骤通过从基础信息性图像生成增强版本,并基于标签保留和增强图像的信息性计算分数。增强样本的总体信息性是这两个分数的总和,最具信息性的样本将被添加到训练集中以进一步训练。
我们提出的GANDALF方法比我们之前的方法GESTALT(Mahapatra等, 2022a)和其他竞争方法表现更佳。随后的消融研究还突出了图注意力Transformer和信息性增强步骤在GANDALF整体性能中的重要性。
在未来的工作中,我们计划在其他多标签医学影像数据集上测试我们的模型。我们还计划测试它在不同分类架构和分割方法中的鲁棒性和泛化能力。我们也预计Transformer将在基于图的可解释性和主动学习任务中发挥更大的作用。因此,我们未来的重点将是利用图注意力Transformer的特性,在结合成像和非成像信息的多组学数据集上学习更强大的图表示。
当前的主动学习方法通常在初始数据集大小为总数据的10%左右的情况下进行测试,正如本研究所执行的那样。未来研究的一个有趣方向是结合新提出的方法来应对更极端的低标注场景,例如Taher等(2023)和Chen等(2023a,b)在主动学习情境下的工作。
Results
结果
5.1. Comparative results for CheXpert dataset
For each baseline method, we measured the Area Under the Curve(AUC) for every 10% increment of training data to simulate an activelearning scenario. Table 1 shows the performance of different methodsat different percentages of the training data. Except for the randombased sample selection method, all the AL-based methods outperformthe fully-supervised learning model (FSL), confirming the benefits ofselecting samples based on their informativeness. Among the other ALmethods, the uncertainty-based approach required 70% of the trainingdata to surpass FSL. This finding aligns with other similar works ,where it has been observed AL methods outperforming the FSL baselinewith lower percentages of data, Mayer and Timofte (2018), Yang et al.(2017) and Sourati et al. (2019) indicating the capability of AL methodsto boost the learning rate of trained models further. This behavior hasnot been fully explained in the literature, but we provide a possiblereason below. In any given dataset there are many samples with noisylabels (inaccurate labels) or noisy images. When a classifier is fedall samples at random it encounters samples with different levels ofinformativeness and quality. As a result the classifier’s learning rateand final performance can be negatively impacted with respect to thesituation where a model only uses highly informative samples that donot have ambiguous labels, leading to improved performance and fasterlearning rates than a fully-supervised model (FSL).Amongst other methods, AlphaMix and GAL are more competitiveand show similar results. LEMAL and CVIRS perform slightly betterthan a vanilla uncertainty approach since they are based on uncertaintycalculation. Our previously proposed method, GESTALT, does betterthan these methods, while LADA is slightly worse than GESTALT. Weshow results for two versions of GANDALF — GANDALF𝐷𝑇 , whenusing saliency maps obtained with the Deep Taylor method, andGANDALF𝐺𝐶, when using GradCAM saliency maps. Of the two,GANDALF𝐷𝑇 shows better results and we refer to it as GANDALF insubsequent discussions.As evidenced by the results, GANDALF yielded significant improvements over GESTALT and LADA by integrating data augmentationwith multi-label AL. GESTALT outperforms FSL at 45% of labeleddata, whereas GANDALF outperforms FSL at 37% of training data.This proves that the proposed approach requires significantly less labeled data to attain better performance. Although LADA combines dataaugmentation with active learning, it does not consider the multilabel scenario. The improved performance of GANDALF is attributedto the fact that: (a) it uses a better method to select most informativesamples based on multi-label interactions, and (2) it leverages dataaugmentation to generate more informative synthetic samples basedon multi-label interactions. We demonstrate this through a series ofablation experiments, presented below.
5.1. CheXpert数据集的比较结果
对于每种基线方法,我们在每10%的训练数据增量上测量了AUC值,以模拟主动学习(AL)的情境。表1展示了不同方法在不同训练数据百分比下的性能表现。除了基于随机选择的样本选择方法外,所有基于AL的方法都优于完全监督学习模型(FSL),这验证了基于样本信息性选择的益处。其他AL方法中,基于不确定性的选择方法需要70%的训练数据才能超过FSL。这个发现与其他类似工作一致,Mayer和Timofte (2018)、Yang等 (2017)、Sourati等 (2019)的研究表明,AL方法可以在较少数据的情况下提升模型的学习速度。
这种现象在文献中并没有得到充分解释,但我们在这里提供了一个可能的原因。在任何给定的数据集中,都可能存在许多带有噪声标签(不准确标签)或噪声图像的样本。当分类器以随机方式接收所有样本时,会遇到不同信息性和质量的样本。因此,与仅使用高度信息性且标签明确的样本相比,分类器的学习率和最终性能可能受到负面影响。而仅使用这些高信息性样本时,模型性能会提高,学习速度会更快,超过完全监督学习模型(FSL)。
在其他方法中,AlphaMix和GAL的竞争力较强,表现相似。LEAML和CVIRS表现略优于基于不确定性的方法,因为它们基于不确定性计算。我们之前提出的方法GESTALT比这些方法表现更好,而LADA则稍逊于GESTALT。我们展示了GANDALF的两个版本的结果——GANDALFDT使用Deep Taylor方法生成的显著性图,GANDALFGC使用GradCAM显著性图。两者中,GANDALFDT表现更佳,后续讨论中我们将其称为GANDALF。
结果表明,GANDALF通过结合数据增强与多标签AL,显著提升了性能。GESTALT在45%的标注数据上超越了FSL,而GANDALF在37%的训练数据上就已经超过了FSL。这表明所提出的方法需要显著更少的标注数据即可获得更好的性能。尽管LADA结合了数据增强与主动学习,但它未考虑多标签场景。GANDALF的改进归因于:(a) 它基于多标签交互使用了更好的方法来选择最具信息性的样本,(b) 它利用数据增强生成基于多标签交互的更多信息性合成样本。我们通过一系列消融实验证明了这一点,详见后续讨论。
Figure
图
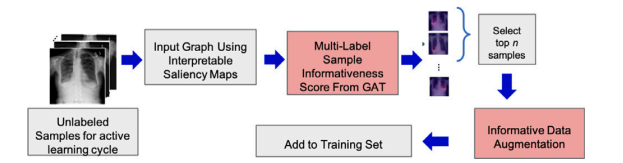
Fig. 1. Workflow of proposed GANDALF method. Given unlabeled pool samples inan active learning cycle, an input graph is constructed using interpretable saliencymaps. They serve as input to a graph-multiset transformer (Fig. 2) which outputs ainformativeness score to rank informative samples. Selected samples are then usedtofurther synthesize informative and non-redundant samples. Selected samples and theirsynthetic derivatives are added to the training dataset for the next active learning cycle.
图1. GANDALF方法的工作流程:在一个主动学习周期中,给定未标记的样本池,首先利用解释性显著图构建输入图。该图作为输入传递给图多重集Transformer(如图2所示),Transformer输出一个信息性评分,用于对信息性样本进行排序。然后,从这些选定的样本中进一步合成信息性且无冗余的样本。最后,选定的样本及其合成样本被添加到训练数据集中,为下一个主动学习周期做准备。
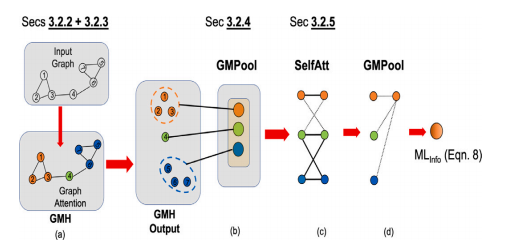
Fig. 2. Graph Multiset Transformer: A graph with 𝑛 nodes depicting multi-labelinformation of a sample is passed through several message-passing layers (a) and anattention-based pooling block (GMPool𝑘 ) (b) to get 𝑘(< 𝑛) nodes. A self-attention block(SelfAtt) © encodes the relationship between 𝑘 nodes, and passes through GMPool1(d), to obtain a single node value. Different node colors indicate different classes andthe edge length denotes node similarity
图2. 图多重集Transformer:该图展示了一个包含n个节点的图,表示一个样本的多标签信息。图经过若干消息传递层(图a)和一个基于注意力的池化块(GMPoolₖ,图b),得到k个节点(k < n)。接着,这些k个节点通过一个自注意力模块(SelfAtt,图c),编码这些节点之间的关系。然后通过GMPool₁池化(图d),得到一个单一的节点值。图中的不同节点颜色表示不同的类别,边的长度则表示节点之间的相似性。
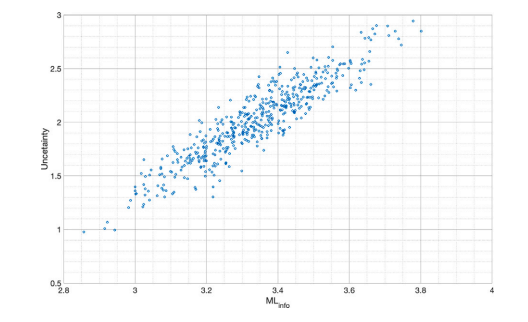
Fig. 3. Scatter plot between 𝑀𝐿𝑖𝑛𝑓 𝑜 and uncertainty values of 500 samples. The pointsare concentrated around a line with a high correlation coefficient (0.92), suggestingthat 𝑀𝐿𝑖𝑛𝑓 𝑜 is a good measure of sample informativeness since uncertainty estimateshave been used previously as a measure of sample informativeness
图3. 500个样本的𝑀𝐿𝑖𝑛𝑓 𝑜和不确定性值之间的散点图:图中的点集中在一条线附近,显示出高度的相关性系数(0.92)。这表明𝑀𝐿𝑖𝑛𝑓 𝑜是衡量样本信息性的一项有效指标,因为不确定性估计以前已经被用作衡量样本信息性的标准。
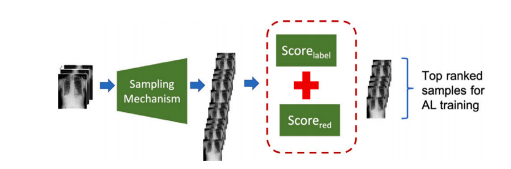
Fig. 4. Steps for augmenting and choosing informative samples from a base informativeone. Given a few base informative samples we generate additional ones by samplingfrom a variational autoencoder (trained with the current training set). However, toensure that only informative generated samples are added to the training set, wecalculate two scores — the label score Score𝑙𝑎𝑏𝑒𝑙 and the redundancy avoidance scoreScore𝑟𝑒𝑑 . The final informativeness of a sample is the weighted sum of these two scores.The top-ranked informative samples are added to the training set for further classifier training
图4. 从基础信息性样本生成和选择信息性样本的步骤:给定少量的基础信息性样本,通过从一个使用当前训练集训练的变分自编码器(VAE)中进行采样生成额外的样本。然而,为了确保只将信息性生成样本添加到训练集中,我们计算两个评分——标签评分Scoreₗₐbₑₗ和冗余避免评分Scoreᵣₑd。样本的最终信息性得分是这两个评分的加权和。得分最高的信息性样本被添加到训练集中,以进行进一步的分类器训练。
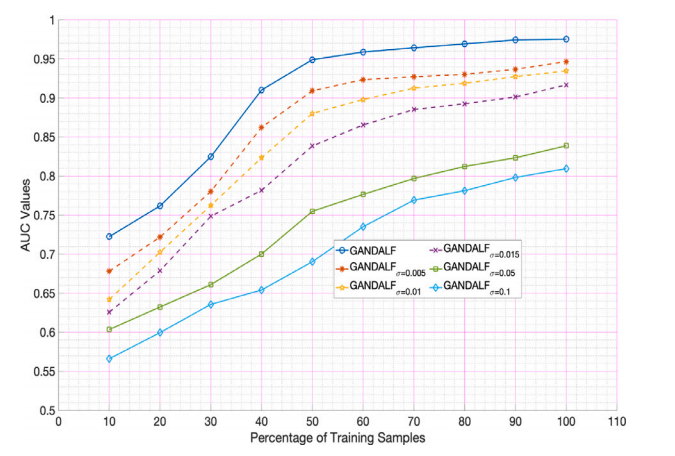
Fig. 5. AUC measures for different features for added Gaussian noise of 𝜇 = 0 and different ?
图5. 不同特征在加入均值为 𝜇 = 0 的高斯噪声后,不同 ? 的AUC衡量。
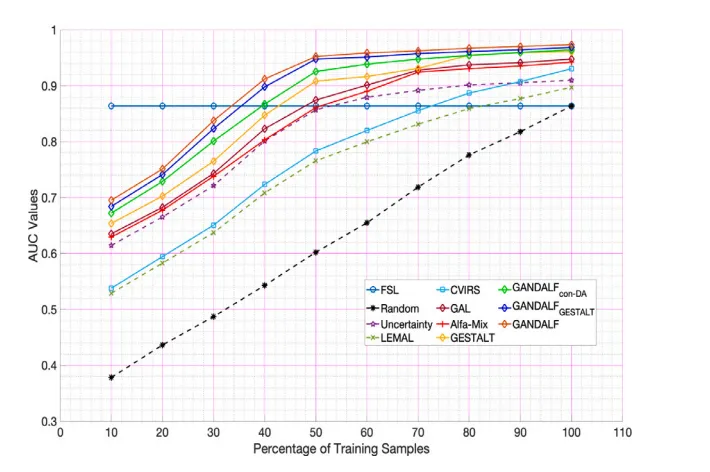
Fig. 6. Additional dataset — NIH DataSet: AUC measures at different percentage levelsof training percentage for baselines (indicated with dotted lines) and the proposedGANDALF approach, including three investigated variants. As a reference, the AUC ofa fully-supervised model (FSL) is also included as a horizontal line. Improved learningrates and model performance is observed for the proposed GANDALF approach.
图6. 附加数据集 — NIH 数据集:展示了基线模型(用虚线表示)和提出的 GANDALF 方法(包括三种变体)在不同训练数据百分比下的 AUC 测量结果。作为参考,完全监督学习模型(FSL)的 AUC 以水平线表示。
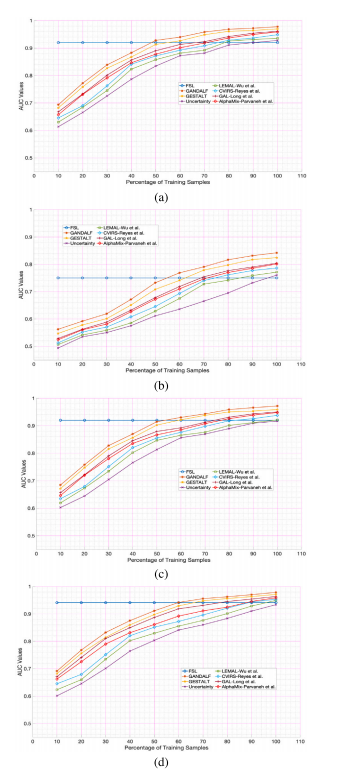
Fig. 7. MedMNIST DataSets: AUC measures at different percentage levels of trainingpercentage for the proposed GANDALF approach and four multi-label Al learningapproaches. As reference, AUC of a fully-supervised model (FSL) is also included asan horizontal line. Improved learning rates and model performance is observed for ourproposed GANDALF approach which outperforms other state of the art methods. (a)Dermatology; (b) Retinal fundus images; © Breast dataset; (d) Tissue dataset.
图7. MedMNIST 数据集:展示了提出的 GANDALF 方法和四种多标签主动学习方法在不同训练数据百分比下的 AUC 测量结果。作为参考,完全监督学习模型(FSL)的 AUC 以水平线表示。可以观察到,提出的 GANDALF 方法显示出更快的学习速度和更好的模型性能,优于其他最先进的方法。具体展示了以下数据集的结果:(a) 皮肤病学数据集;(b) 视网膜眼底图像数据集;© 乳腺数据集;(d) 组织数据集。
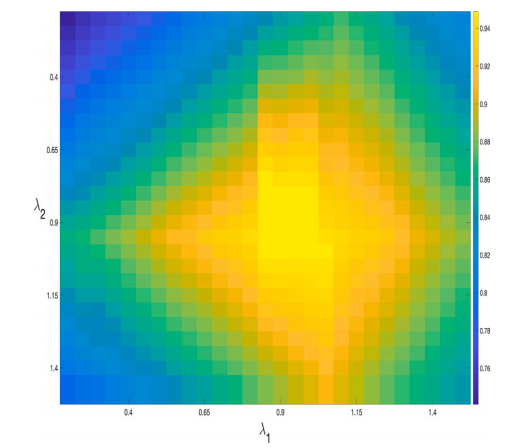
Fig. 8. Heat map showing AUC values at 50% labeled training data for different valuesof 𝜆1 , 𝜆2 .
图8. 热力图展示了在50%标注训练数据下,不同 𝜆₁ 和 𝜆₂ 值对应的 AUC 值。
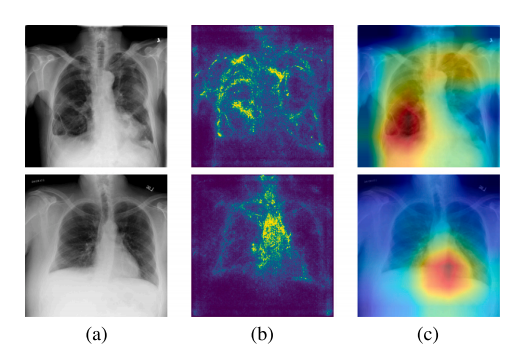
Fig. 9. Comparative visualization of GradCAM and Deep Taylor models. (a) originalimage; Saliency maps using (b) Deep Taylor method; © Grad-CAM method. Especiallyfor the bottom row image, the Deep Taylor method gives a more accurate localizationof informative regions than Grad-CAM.
图9. GradCAM 和 Deep Taylor 模型的对比可视化。(a)原始图像;显著图:(b)Deep Taylor 方法;(c)Grad-CAM 方法。尤其是对于底部的图像,Deep Taylor 方法比 Grad-CAM 更准确地定位了信息区域。
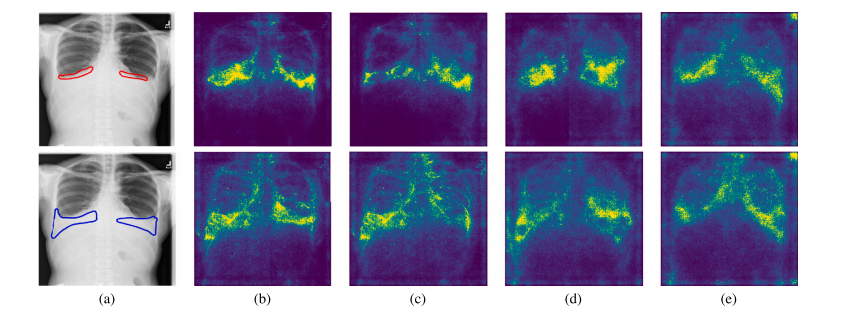
Fig. 10. (a) Base informative image with expert-annotated outlines of diagnosed conditions. Saliency maps for different methods: (b) base image; ©–(e) Different informative images generated from the base image. Top row shows Pleural Effusion image and bottom row shows Atelactasis
图10. (a) 基础信息性图像,带有专家标注的诊断条件轮廓。不同方法的显著图:(b)基础图像;(c)–(e)从基础图像生成的不同信息性图像。上排显示的是胸腔积液图像,下排显示的是肺不张图像。
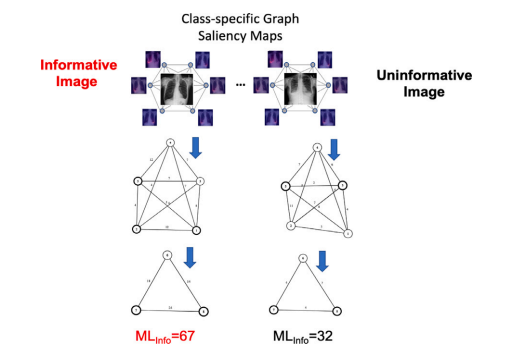
Fig. 11. Illustration of graph construction of informative and non-informative images.
图11. 信息性图像和非信息性图像的图结构构建示意图。
Table
表
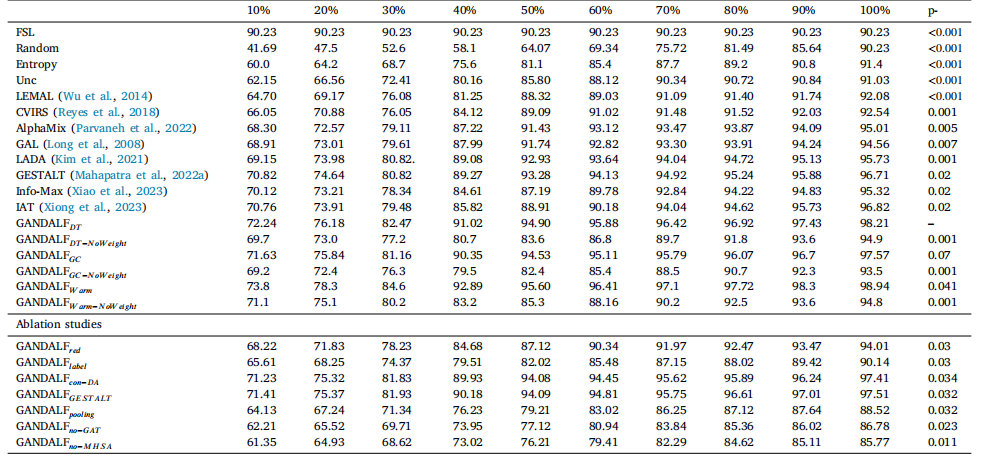
Table 1AUC values for different baselines and proposed approach along with ablation studies. The 𝑝−values are with respect to GANDALF. DT: DeepTaylor; GC: GradCAM; Warm: WarmAL start; red: GANDALF with only redundancy avoidance; label: GANDALF with only label preservation; con-DA: Conventional Data Augmentation; GESTALT: GESTALT for sampleinformativeness, and GANDALF for informative augmentation; pooling: Conventional pooling; no-GAT: No Graph Attention; no-SA: No Self-Attention
表1 不同基线和提出的方法的AUC值以及消融研究。𝑝-值是相对于GANDALF的。DT:DeepTaylor;GC:GradCAM;Warm:WarmAL启动;red:仅包含冗余规避的GANDALF;label:仅包含标签保留的GANDALF;con-DA:传统数据增强;GESTALT:GESTALT用于样本信息性,GANDALF用于信息增强;pooling:传统池化;no-GAT:无图注意力机制;no-SA:无自注意力机制。

Table 2Ranking Loss (lower is better) and label ranking average precision (LRAP, higher is better) values for different methods in the multi-label 𝐾 = 5setting
表2:在多标签 𝐾 = 5 设置下,不同方法的排序损失(Ranking Loss,越低越好)和标签排序平均精度(Label Ranking Average Precision,LRAP,越高越好)值。
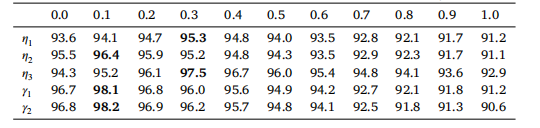
Table 3AUC values for GANDALF for different values of the parameters 𝜂1 , 𝜂2 , 𝜂3 , 𝛾1 , 𝛾2 .
表3:GANDALF 方法在不同参数 𝜂₁、𝜂₂、𝜂₃、𝛾₁、𝛾₂ 下的 AUC 值。

Table 4Training and inference time for different methods
表4:不同方法的训练和推理时间。
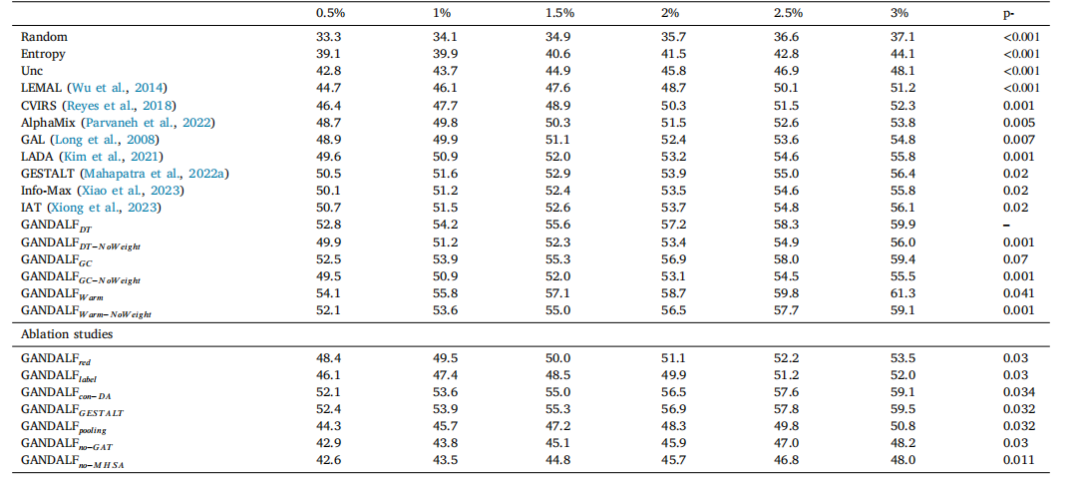
Table 5Results for low data scenarios: AUC values for different baselines and proposed approach along with ablation studies. The 𝑝−values are with respect to GANDALF. DT😃eepTaylor; GC: GradCAM; Warm: Warm AL start; red: GANDALF with only redundancy avoidance; label: GANDALF with only label preservation; con-DA: Conventional DataAugmentation; GESTALT: GESTALT for sample informativeness, and GANDALF for informative augmentation; pooling: Conventional pooling; no-GAT: No Graph Attention; no-SA: NoSelf-Attention.
表5 少量数据场景的结果:不同基线和提出的方法的AUC值以及消融研究。𝑝-值是相对于GANDALF的。DT:DeepTaylor;GC:GradCAM;Warm:Warm AL启动;red:仅包含冗余规避的GANDALF;label:仅包含标签保留的GANDALF;con-DA:传统数据增强;GESTALT:GESTALT用于样本信息性,GANDALF用于信息增强;pooling:传统池化;no-GAT:无图注意力机制;no-SA:无自注意力机制。