Title
题目
PathoDuet: Foundation models for pathological slide analysis of H&E and IHC stains
PathoDuet: H&E 和 IHC 染色病理切片分析的基础模型
01
文献速递介绍
组织学评估一直是诊断特定癌症的黄金标准,主要依赖于病理学家的专业知识。评估通常基于对苏木精-伊红(H&E)染色切片的分析,这为诊断提供了基本的结构信息。病理学家还可能利用免疫组织化学(IHC)等功能性染色方法,提供额外的诊断见解。随着技术的进步,具有高通量的数字扫描仪彻底改变了病理数据的获取。然而,尽管数据量庞大,深度学习技术在诊断过程中的应用仍然相对缓慢,这部分归因于某些任务的标注数据有限。与自然图像的标注不同,病理图像的标注需要专家的参与,因此耗费大量资源和时间。
为了解决这一挑战,基础模型作为一种前景广阔的解决方案应运而生。这些模型通常利用未标注数据的潜力,从而减少对标注数据的依赖,并高效地迁移至下游任务。
现有的基础模型主要依赖自监督学习(SSL)方法。SSL的核心是直接从数据中生成监督信号,这一过程通常称为预训练任务。作为SSL方法的一个重要分支,对比学习(CL)引起了广泛关注。一般来说,CL通过利用图像相似性来区分和分类图像。另一分支是通过掩码自动编码器(MAE),利用图像生成来提升模型的理解能力。与生成型SSL方法相比,CL在迁移到区分任务时表现更佳。因此,考虑到许多病理学任务与识别高度相关,我们选择在此工作中优先采用CL。
然而,将为自然图像设计的CL方法直接应用于组织病理图像时需谨慎。CL假定大多数图像在语义上是独特的,通常的做法是对同一图像的不同视图进行对比,并在语义空间中分离不同的图像。病理全切片图像通常被裁剪为较小的图像块以符合大多数模型的输入要求,这限制了裁剪图像块与邻近图像块在语义上的独特性。过度隔离这些图像块可能导致语义空间的过度碎片化,从而影响模型的性能。因此,我们需要针对组织病理图像的特性设计特殊的对比预训练任务。
病理学家的典型工作方法之一是习惯性地在检查过程中进行放大和缩小操作。他们通常先使用低倍镜观察整体结构和组织,找到需要进一步检查的感兴趣区域,然后在高倍镜下分析单个细胞或细胞群体,以细化他们对已识别区域的理解。为了模拟病理学家在检查过程中进行的放大和缩小操作,我们定义了“跨尺度定位”任务,利用大规模公开的H&E数据集开发H&E染色的基础模型。除了常用的CL中不同增强视图的两个分支外,我们还增加了一个分支,学习图像块与其邻近区域的表示。这一操作使图像块从更广泛的角度被理解,缓解了CL对语义分离的要求与病理图像块集中性之间的冲突。
此外,病理学家常常利用额外的功能性切片进行更全面的诊断,IHC标记物经常被用于癌症的亚型分类。然而,仅凭IHC染色切片难以进行有效的解释,H&E染色切片作为基本的参考,提供了必要的上下文信息和结构细节,补充了从IHC切片中获得的分子信息。因此,理想的IHC染色基础模型应能够根据标记物的表达水平评估IHC图像,并在组织结构方面与H&E模型在语义空间中对齐。由于公开的IHC数据有限,我们利用已训练的H&E基础模型并引入了“跨染色迁移”预训练任务,以加深对不同染色方式的病理图像的理解。具体而言,我们通过自适应实例归一化(AdaIN)对齐IHC表示与IHC风格转化的H&E表示。此对齐注入了H&E图像中可直接获取的结构信息,同时保留了IHC图像中的诊断信息。
我们还引入了一个预文本标记机制来统一这两个预训练任务。两项任务都需要一种不同形式的辅助输入,即一个更小的图像块或一个染色提示。与设计单独的网络来处理额外输入相反,我们将包含辅助信息的额外标记输入Vision Transformer(ViT)模型,并通过网络训练过程将跨尺度或跨染色信息与原始表示结合。随后通过一个精心设计的模块,即任务增强器,明确关联这两种形式的输入。该机制以轻量化的方式增强了模型发现和利用任务与染色模式之间内在关联的能力。
Abatract
摘要
Large amounts of digitized histopathological data display a promising future for developing pathologicalfoundation models via self-supervised learning methods. Foundation models pretrained with these methodsserve as a good basis for downstream tasks. However, the gap between natural and histopathological imageshinders the direct application of existing methods. In this work, we present PathoDuet, a series of pretrainedmodels on histopathological images, and a new self-supervised learning framework in histopathology. Theframework is featured by a newly-introduced pretext token and later task raisers to explicitly utilize certainrelations between images, like multiple magnifications and multiple stains. Based on this, two pretext tasks,cross-scale positioning and cross-stain transferring, are designed to pretrain the model on Hematoxylin andEosin (H&E) images and transfer the model to immunohistochemistry (IHC) images, respectively. To validatethe efficacy of our models, we evaluate the performance over a wide variety of downstream tasks, includingpatch-level colorectal cancer subtyping and whole slide image (WSI)-level classification in H&E field, togetherwith expression level prediction of IHC marker, tumor identification and slide-level qualitative analysis in IHCfield. The experimental results show the superiority of our models over most tasks and the efficacy of proposedpretext tasks.
大量数字化的组织病理学数据为通过自监督学习方法开发病理学基础模型展示了光明的前景。通过这些方法预训练的基础模型为下游任务提供了良好的基础。然而,自然图像与组织病理图像之间的差异阻碍了现有方法的直接应用。在这项工作中,我们提出了PathoDuet,这是一个基于组织病理图像的预训练模型系列,并引入了一种新的组织病理学自监督学习框架。该框架的特点是引入了一个全新的预文本标记和后续任务增强器,以明确利用图像之间的某些关系,例如多重放大率和多种染色方式。在此基础上,我们设计了两个预训练任务,分别为跨尺度定位和跨染色迁移,来对模型进行预训练,使其适用于苏木精-伊红(H&E)染色图像,并将其迁移至免疫组织化学(IHC)染色图像领域。为了验证我们模型的有效性,我们在多个下游任务中评估了其性能,包括H&E领域的病理切片级别结直肠癌亚型分类、全切片图像(WSI)级别的分类,以及IHC领域的标记物表达水平预测、肿瘤识别和切片级别的定性分析。实验结果表明,我们的模型在大多数任务上表现出优越性,并证明了所提出的预训练任务的有效性。
Method
方法
In this section, we first describe the introduction of a pretext tokenand subsequent task raiser module to unify the proposed two pretexttasks. The details of the tasks are discussed in the following subsections, including the real-world inspiration and the imitation with thecontrastive learning framework.
在本节中,我们首先介绍预文本标记的引入以及后续的任务增强模块,以统一所提出的两个预训练任务。接下来的小节中将详细讨论这些任务的细节,包括其在现实世界中的灵感来源以及与对比学习框架的模拟过程。
Conclusion
结论
We introduce PathoDuet, a series of foundation models on computational pathology, covering both H&E and IHC images, and proposea new self-supervised learning framework with two pretext tasks inpathology. The key to this framework is the introduction of a pretexttoken and following task raisers. It consists of both a model pretrainingtask, cross-scale positioning, and a model adaptation task, cross-staintransferring. In cross-scale positioning, we bridge the local and globalrepresentations of H&E patches to enhance pathological image understanding in various magnifications. In cross-stain transferring, weutilize adaptive instance normalized H&E features to provide pseudoIHC features injected with structural information. The original H&Emodel is therefore transferred to an interpreter of IHC images. Weevaluate the performance of our models over a wide variety of downstream tasks, and the experimental results show the efficacy of ourmodels on most tasks. Besides, we also investigate the downstreamdata requirements and comparison with giant pathological models,to discover the power of data and delicately designed SSL methodstailored to pathological images. PathoDuet highlights the importanceof training strategy, while the giants, UNI and Virchow, point out theadvantage of preparing sufficient training data. Hence, we will take allefforts to collect more data to iterate and upgrade our models in thefuture.
我们介绍了PathoDuet,这是一个在计算病理学领域的基础模型系列,涵盖了H&E和IHC图像,并提出了一个新的自监督学习框架,包含两个病理学预训练任务。该框架的关键在于引入了预文本标记和后续的任务增强器。它由模型预训练任务(跨尺度定位)和模型适应任务(跨染色迁移)组成。在跨尺度定位任务中,我们连接了H&E图像块的局部和全局表示,以增强在不同放大倍率下对病理图像的理解。在跨染色迁移任务中,我们利用自适应实例归一化的H&E特征,生成注入了结构信息的伪IHC特征,从而将原始H&E模型转化为IHC图像的解释器。
我们在多个下游任务上评估了模型的性能,实验结果显示我们的模型在大多数任务上都表现出色。此外,我们还研究了下游数据需求,并与巨型病理模型进行了比较,探索了数据的力量和针对病理图像精心设计的自监督学习方法的效果。PathoDuet强调了训练策略的重要性,而巨型模型UNI和Virchow则展示了充分准备训练数据的优势。因此,我们将全力收集更多数据,以便在未来迭代和升级我们的模型。
Results
结果
In Table 1, we evaluate our H&E model using the linearprobing method under different amounts of data. From the result,we can see that our model performs well across various amountsof training data over other pretrained models. Meanwhile, it can beobserved that a generally consistent increasing trend exists with thegrowth of amounts of training data, but the difference is relativelysmall for most models. A further study is conducted in Section 5.2on the training data requirements of foundation models. Notably, thegiant UNI shows a dominant performance when the training data isextremely limited, which demonstrates its general interpretability ofpathological images. In Table 2, we present the evaluation of models’performance under different training strategies using the whole NCTCRC-HE dataset. The results demonstrate that the proposed model is agood interpreter of H&E images under both a quick linear transferringmanner and a thorough full fine-tuning protocol. The performance gaincan be owed to the cross-scale positioning task, which enhances themodel’s understanding under a broader view. To verify the assumption,an ablating study is discussed in Section 5.1. UNI also provides decentperformance, which shows its great understanding in pathology andpowerful ViT-Large architecture.
在表 1 中,我们使用线性探测方法在不同的数据量下评估了我们的 H&E 模型。结果显示,我们的模型在不同训练数据量下相较于其他预训练模型表现良好。同时,可以观察到随着训练数据量的增加,模型的表现呈现出总体一致的增长趋势,但对于大多数模型来说,差异相对较小。在第 5.2 节中对基础模型的训练数据需求进行了进一步研究。值得注意的是,巨大的 UNI 模型在训练数据极其有限时表现出色,展示了其对病理图像的广泛解释能力。
在表 2 中,我们展示了使用完整 NCT CRC-HE 数据集在不同训练策略下的模型表现评估。结果表明,所提出的模型无论是在快速线性迁移方式下还是在完整的全量微调协议下,都是 H&E 图像的良好解释者。性能的提升归因于跨尺度定位任务,该任务增强了模型在更广泛视角下的理解能力。为了验证这一假设,第 5.1 节讨论了消融研究。UNI 也提供了出色的性能,展示了其在病理学中的卓越理解能力和强大的 ViT-Large 架构。
Figure
图
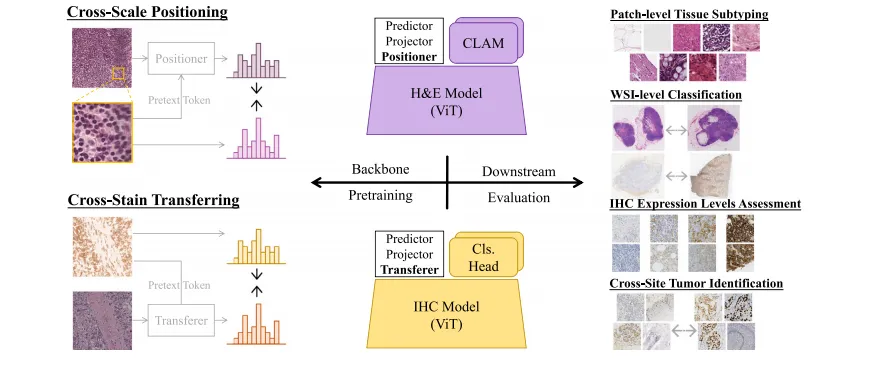
Fig. 1. An overview of PathoDuet. Left: two pretext tasks, cross-scale positioning and cross-stain transferring, are designed to develop H&E and IHC models. Right: a series ofdownstream tasks, covering both H&E and IHC ones, are used to evaluate models’ performance in application.
图 1. PathoDuet 概览。左侧:设计了跨尺度定位和跨染色迁移两个预训练任务,用于开发 H&E 和 IHC 模型。右侧:一系列涵盖 H&E 和 IHC 的下游任务用于评估模型在实际应用中的性能。
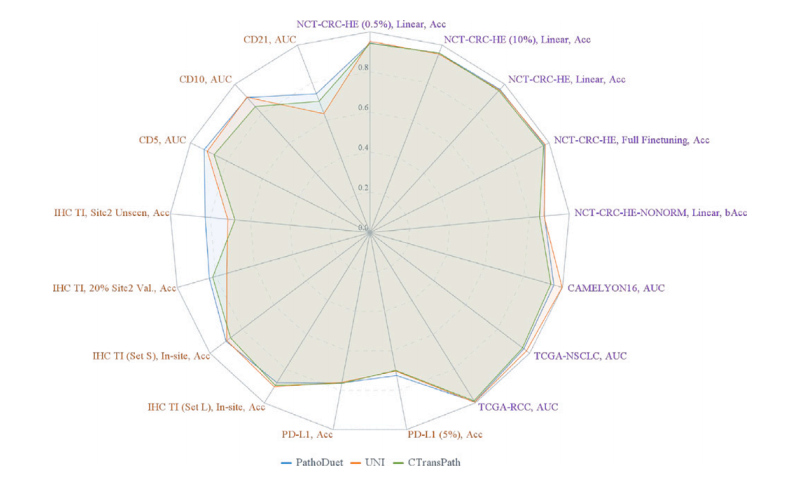
Fig. 2. An overall performance visualization. Each task is named as training dataset,(special settings,) evaluating metric. H&E tasks are colored purple, and IHC ones areyellow.
图 2. 整体性能可视化。每个任务以 训练数据集, (特殊设置,) 评估指标 命名。H&E 任务以紫色表示,IHC 任务以黄色表示。
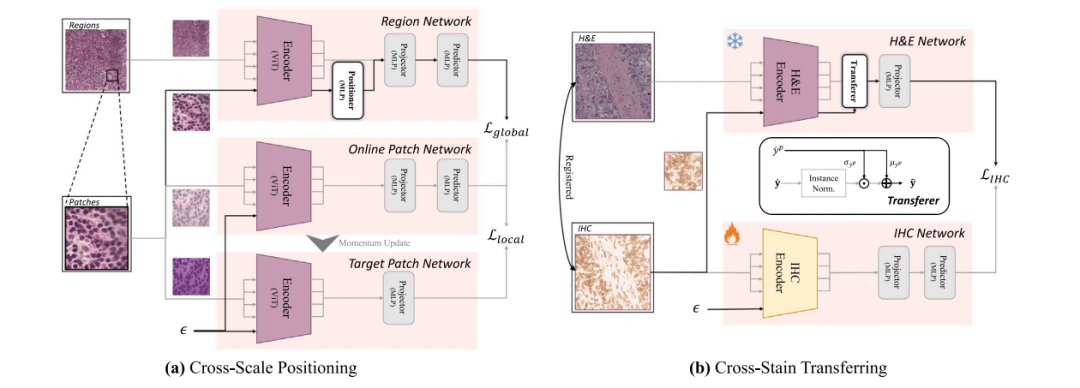
Fig. 3. Detailed networks of two pretext tasks. The flow of pretext token is represented by the black arrows, 𝜖 is the placeholder, and the task raisers (positioner and transferer)are presented in the white blocks. (a) Three-branch cross-scale positioning network. (b) Two-branch cross-stain transferring network and the transferer module.
图 3. 两个预训练任务的详细网络结构。预文本标记的流动由黑色箭头表示,𝜖 为占位符,任务增强模块(定位器和迁移器)在白色块中显示。(a) 三分支跨尺度定位网络。(b) 双分支跨染色迁移网络及迁移模块。
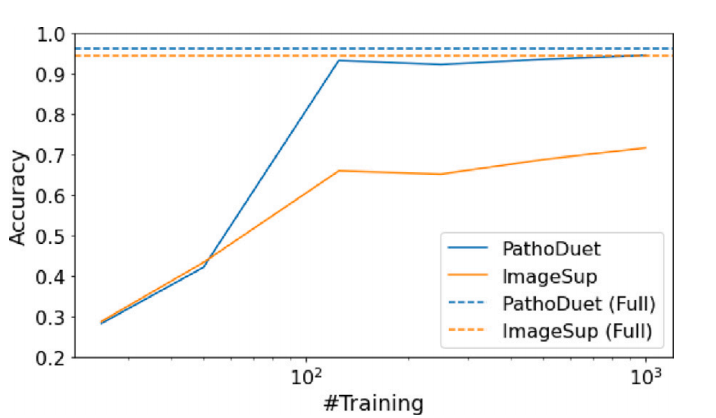
Fig. 4. Data requirement study on NCT-CRC-HE dataset. PathoDuet is compared withImageSup and the performance with the full dataset as an upper bound is representedby the dotted line with the same color.
图 4. NCT-CRC-HE 数据集上的数据需求研究。将 PathoDuet 与 ImageSup 进行比较,并以相同颜色的虚线表示完整数据集的性能上限。
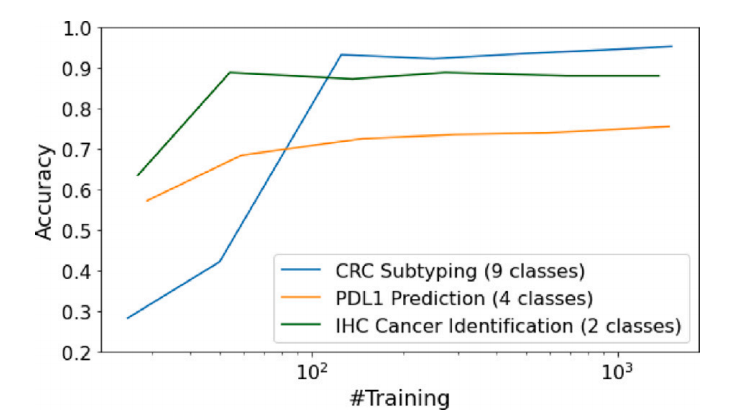
Fig. 5. Data requirement study on different datasets using PathoDuet
图 5. 使用 PathoDuet 在不同数据集上的数据需求研究。
Table
表
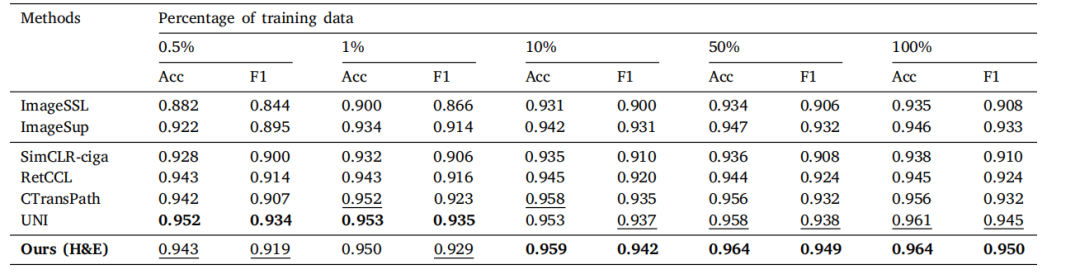
Table 1Linear evaluation results on NCT-CRC-HE dataset with different amounts of training data. The best performance in each column is bold, andthe second best is underlined.
表 1 不同训练数据量下NCT-CRC-HE数据集的线性评估结果。每列中的最佳性能以粗体显示,次优性能以下划线标出。
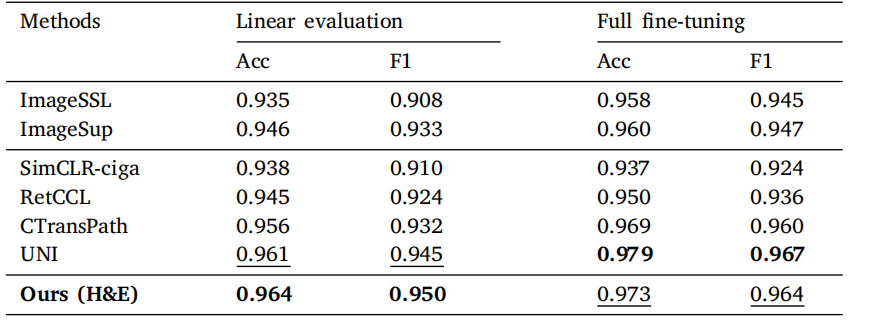
Table 2Results on NCT-CRC-HE dataset for 2 different strategies: linear evaluation, fullfine-tuning. The best performance in each column is bold, and the second best isunderlined.
表 2 NCT-CRC-HE 数据集上两种不同策略的结果:线性评估和全量微调。每列中的最佳性能以粗体显示,次优性能以下划线标出。
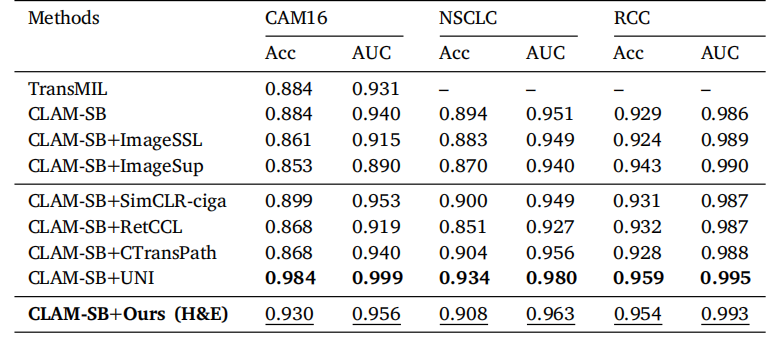
Table 3Results of weakly-supervised WSI classification on three public datasets. The bestperformance in each column is bold, and the second best is underlined.
表 3三个公开数据集上弱监督WSI分类的结果。每列中的最佳性能以粗体显示,次优性能以下划线标出。
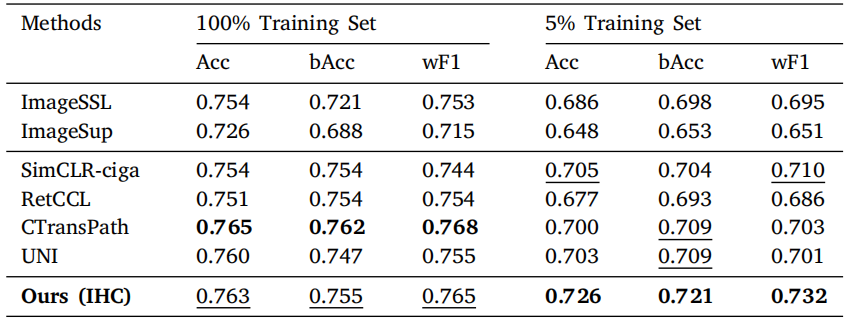
Table 4Results of PD-L1 expression level assessment. The best performance in each column isbold, and the second best is underlined.
表 4PD-L1 表达水平评估的结果。每列中的最佳性能以粗体显示,次优性能以下划线标出。
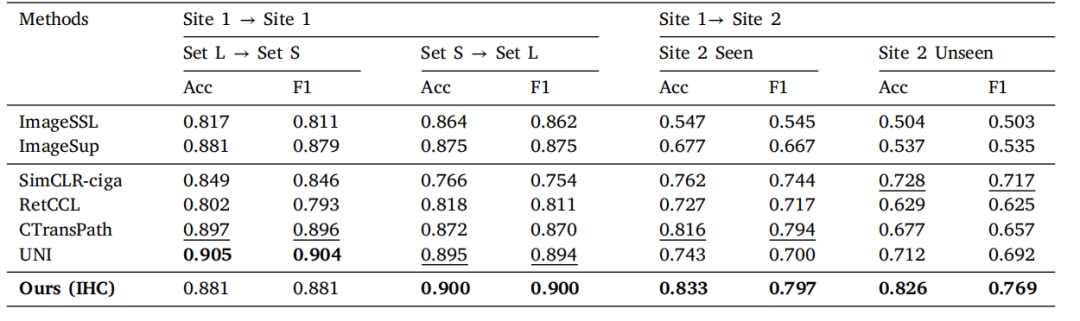
Table 5Results of patch-level tumor identification in IHC images. The best performance in each column is bold, and the second bestis underlined.
表 5IHC 图像中切片级别肿瘤识别的结果。每列中的最佳性能以粗体显示,次优性能以下划线标出。
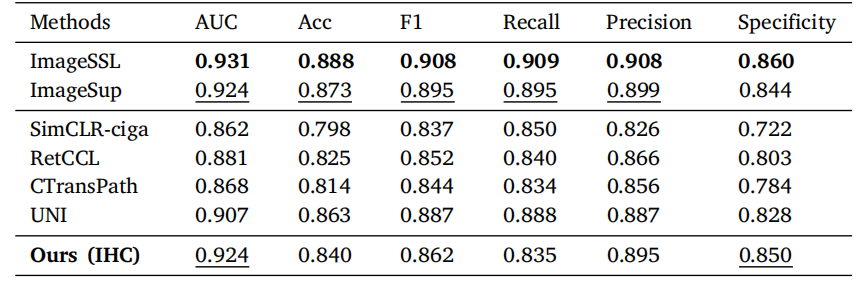
Table 6Results of slide-level prediction of CD5. The best performance in each column is bold,and the second best is underlined.
表 6CD5 切片级别预测的结果。每列中的最佳性能以粗体显示,次优性能以下划线标出。
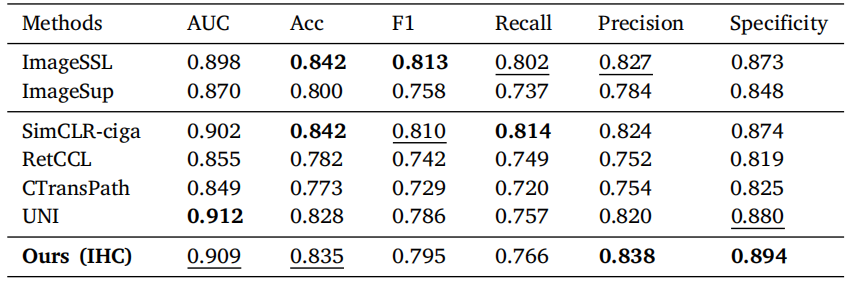
Table 7Results of slide-level prediction of CD10. The best performance in each column is bold,and the second best is underlined.
表 7CD10 切片级别预测的结果。每列中的最佳性能以粗体显示,次优性能以下划线标出
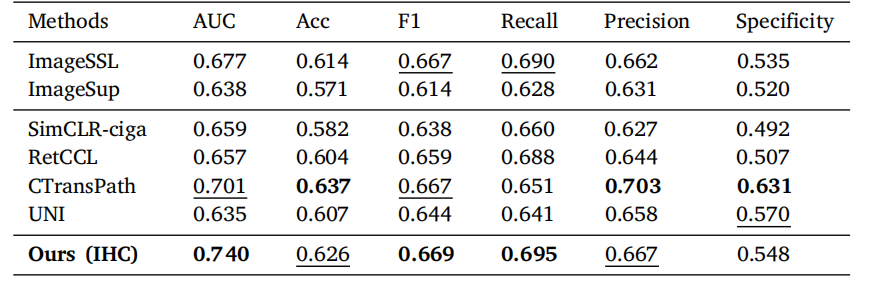
Table 8Results of slide-level prediction of CD21. The best performance in each column is bold,and the second best is underlined.
表 8CD21 切片级别预测的结果。每列中的最佳性能以粗体显示,次优性能以下划线标出。
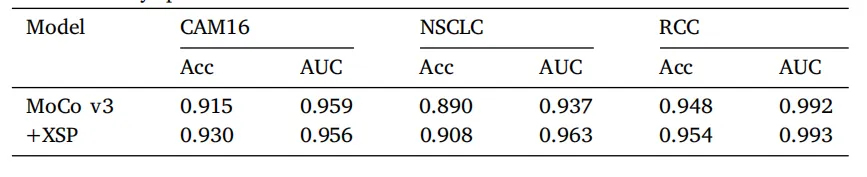
Table 9Ablation study: performance on WSI classification.
表 9消融研究:WSI 分类任务的性能表现。
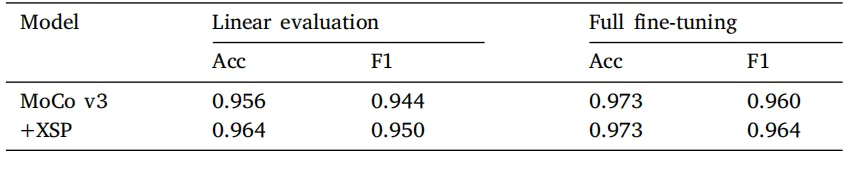
Table 10Ablation study: performance on H&E patch classification.
表 10消融研究:H&E 切片分类任务的性能表现。

Table 11Ablation study: performance on PD-L1 expression level assessment.
表 11消融研究:PD-L1 表达水平评估的性能表现。

Table 12Comparative study on NCT-CRC-HE and NCT-CRC-HE-NONORM dataset. SwinT* means a hybrid model of CNN and Swin Transformer. Thebest performance in each column is bold, and the second best is underlined.
表 12NCT-CRC-HE 和 NCT-CRC-HE-NONORM 数据集的比较研究。SwinT 表示 CNN 和 Swin Transformer 的混合模型。每列中的最佳性能以粗体显示,次优性能以下划线标出。